A Fuzzy Decision Variables Framework for Large-Scale Multiobjective Optimization
IEEE Transactions on Evolutionary Computation(2023)
摘要
In large-scale multiobjective optimization, too many decision variables hinder the convergence search of evolutionary algorithms. Reducing the search range of the decision space will significantly alleviate this puzzle. With this in mind, this article proposes a fuzzy decision variables framework for large-scale multiobjective optimization. The framework divides the entire evolutionary process into two main stages: 1) fuzzy evolution and 2) precise evolution. In fuzzy evolution, we blur the decision variables of the original solution to reduce the search range of the evolutionary algorithm in the decision space so that the evolutionary population can quickly converge. The degree of fuzzification gradually decreases with the evolutionary process. Once the population approximately converges, the framework will turn to precise evolution. In precise evolution, the actual decision variables of the solution are directly optimized to increase the diversity of the population so as to be closer to the true Pareto optimal front. Finally, this article embeds some representative algorithms into the proposed framework and verifies the framework's effectiveness through comparative experiments on various large-scale multiobjective problems with 500 to 5000 decision variables. The experimental results show that in large-scale multiobjective optimization, the framework proposed in this article can significantly improve the performance and computational efficiency of multiobjective optimization algorithms.
更多查看译文
关键词
Optimization,Fuzzy sets,Pareto optimization,Convergence,Search problems,Statistics,Sociology,Decision variable,evolutionary algorithms,fuzzy evolution,large-scale optimization,multiobjective optimization
AI 理解论文
溯源树
样例
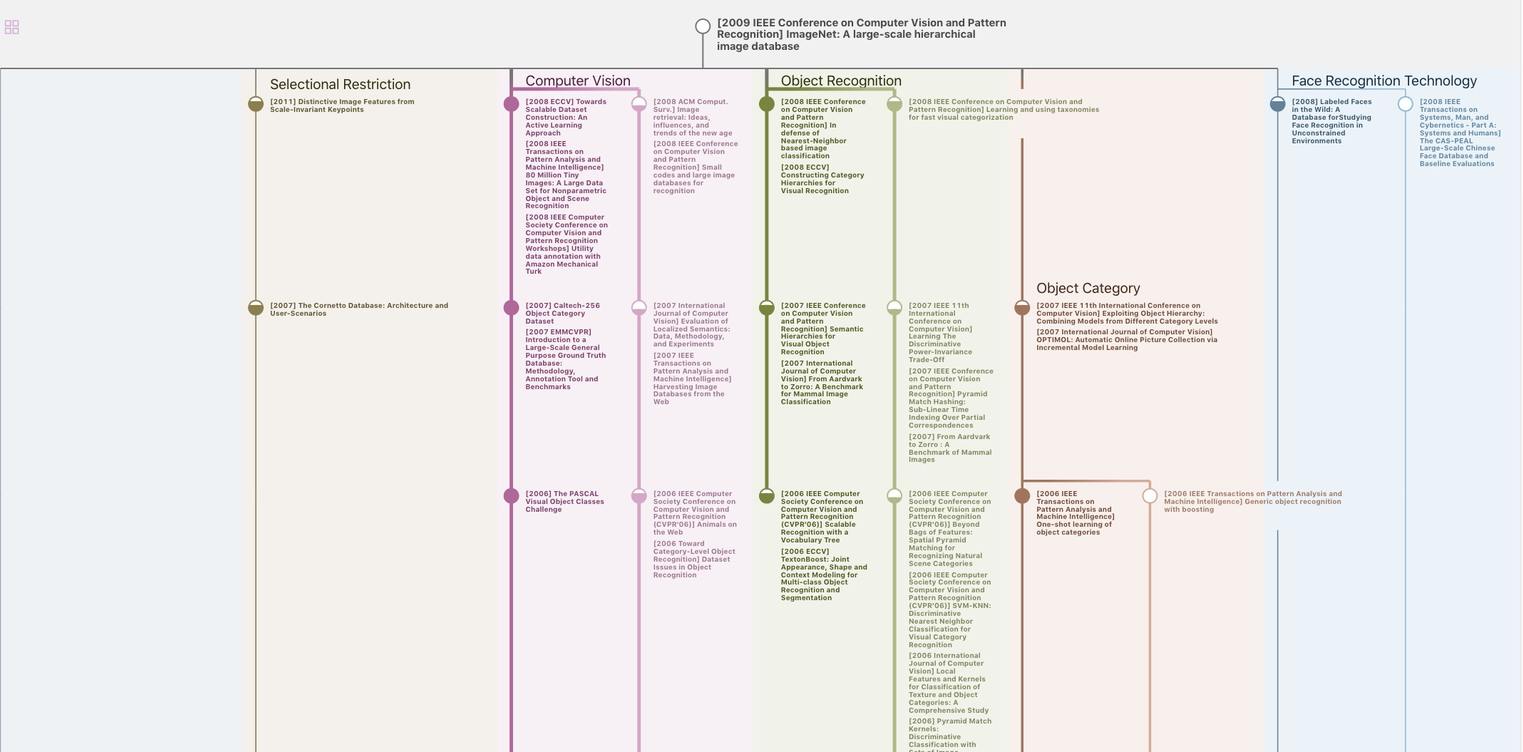
生成溯源树,研究论文发展脉络
Chat Paper
正在生成论文摘要