Preventing Opioid Overdose: From Prediction to Operationalization
Social Science Research Network(2021)
摘要
The opioid epidemic remains a significant public health challenge in the US. A catalyst for reducing the incidence of opioid-related harm could be the development and operationalization of patient risk stratification models. Prior work has focused on the statistical performance of such models, usually tailored to specific adverse events or sub-populations, without considering operational implications. A particular challenge in this context is predicting the most severe outcomes (fatal overdoses) due to imbalanced datasets. We develop a single population-level model for predicting a full range of adverse opioid-related events. We further explore the capacity requirements of interventions based on our model and address salient implementation trade-offs. We partner with Staten Island Performing Provider System to access claims data and electronic health records for the patient population on Staten Island. We develop a machine learning model for predicting adverse opioid-related outcomes. Subsequently, we conduct a rolling horizon analysis to evaluate the capacity requirements of intervention policies leveraging the model. Finally, we quantify the trade-off in predictive performance against concerns that arise in implementation, such as interpretability, data delay, and prediction horizon length. Our single model risk stratification framework achieves an Area Under the Receiver Operating characteristic Curve (AU-ROC) of 0.95, 0.87, 0.83 for the outcomes of any adverse opioid event, opioid overdose, and fatal opioid overdose, respectively. This model can be used to identify a small intervention cohort (1% of the highest-risk patients) which includes the majority (69%) of adverse opioid events. Our results suggest that predictive performance does not need to be sacrificed to satisfy implementation constraints. Accurate risk stratification models are operationally feasible for implementation, even in the absence of training data on fatal overdoses. The implementation of such models allows for targeted interventions with limited intervention capacity and in the presence of operational constraints.
更多查看译文
AI 理解论文
溯源树
样例
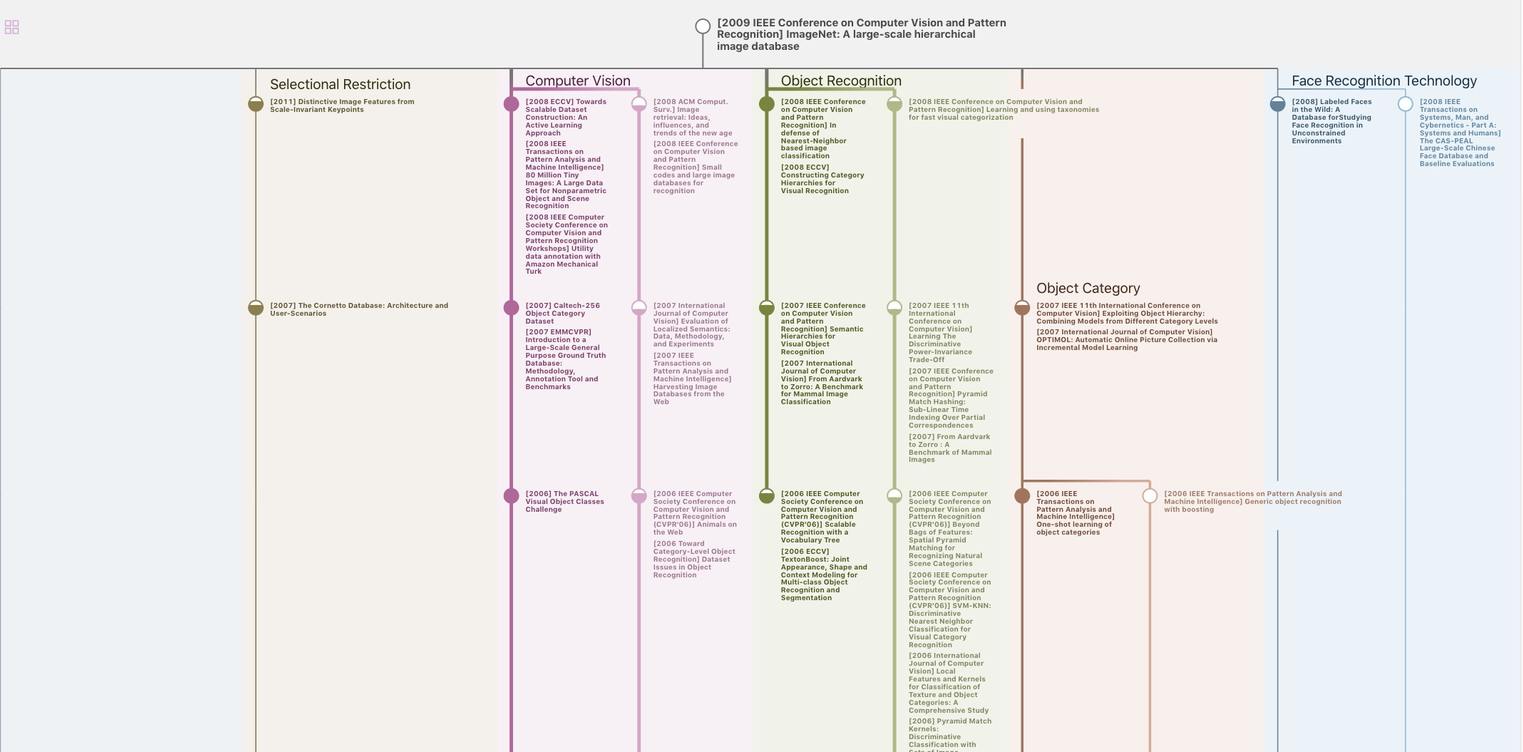
生成溯源树,研究论文发展脉络
Chat Paper
正在生成论文摘要