Field Tests of Smart Mos Gas Sensor Systems for Selective Quantification of VOCs
239th ECS Meeting with the 18th International Meeting on Chemical Sensors (IMCS) (May 30-June3, 2021)(2021)
Abstract
Metal oxide semiconductor (MOS) gas sensors are excellent candidates for real-time indoor air quality monitoring due to their outstanding sensitivity for a broad spectrum of gases and low price. Selective detection and quantification in complex environments represent the ultimate challenge since some gases may be harmful at very low concentration while others are tolerable at much higher levels. To achieve this selective quantification with sensor systems based on physical (several sensitive layers) or virtual (temperature cycling) multisensors complex calibration and application of machine learning algorithms is needed [1]. These models are normally trained in complex gas mixing systems [2] and testing for generalizability is often omitted. In this work we show the performance of lab-calibrated smart sensors in field measurements of volatile organic compounds (VOCs) using release tests accompanied by reference measurements wherever possible. For this multilayer SGP30 sensors (Sensirion AG, Switzerland, [3], 4 sensors in total were measured, presented results are based on one of the sensors) in temperature cycled operation (TCO) were calibrated with randomized gas mixtures [4] containing hydrogen (H 2 , 400-4000 ppb), carbon monoxide (CO, 150-2000 ppb), ethanol (4-1000 ppb), toluene (4-1000 ppb), formaldehyde (1-400 ppb) and acetone (17-1000 ppb). The temperature cycle with a duration of 120 s comprises 10 phases at high temperature (400 °C, 5 s) each followed by a low temperature phase (100 °C, 125 °C, ..., 375 °C, 7 s). Features are extracted by computing the slope of 120 equidistant ranges, yielding 120 features per layer and 480 per sensor. For each sensor, a feature selection by recursive feature elimination is performed and PLSR (partial least squares regression) models predicting the concentration of the individual gases and the TVOC concentration (total volatile organic compounds, for this measurement: sum of gases except H 2 and CO) both in ppb and µg/m³ are trained. The obtained RMSE values from a 10-fold cross-validation can be found in Tab. 1. The sensors are then brought to the field – a regular office at our lab – where they operated for 2-3 weeks followed by a repetition of the lab measurement followed by another 2-3 weeks of operation in the field followed by a final lab measurement. In the field several VOC release tests were conducted: toluene, acetone, ethanol, isopropanol, xylene, and limonene were released by evaporating liquid in the middle of the room. H 2 is released via a controlled mass flow from a gas cylinder and CO generated by a tealight candle. Reference measurements were performed via thermal desorption (Tenax TA and Graphitized Carbon Universal, Markes International GmbH, Germany) with GC-MS analysis (for toluene, xylene, acetone), a portable GC-PID device (Draeger X-PID, Drägerwerk AG & Co. KGaA, Germany; acetone, isopropanol, toluene, xylene) and via a Peak Performer 1 RCP (Peak Laboratories, LLC, USA) chromatograph for H 2 . For the lab-calibrated substances the results show that selective signals can be obtained from the smart sensor system: only the output signal corresponding to the released component increases significantly during the test and recovers over hours through self-ventilation and decomposition inside the room. The predicted concentrations are a little below the theoretical maximum concentration determined from the volumes of the room and liquid released. Where reference data are available, we sometimes observe an offset between sensor and reference, especially with increasing time from last calibration, but relative values (signal height and shape over time) show an excellent match in all cases. The TVOC concentrations quantified in ppb and µg/m³ also match the released amount of gas. As an example, Fig. 1 shows the toluene sensor signal and obtained reference values and Fig. 2 all sensor signals during a release of 0.164 ml toluene and 0.114 ml acetone (corresponding to 600 ppb in the room, if evenly distributed, two release tests are shown). For substances not covered by the lab calibration different types of behaviors were observed. Xylene release affects the toluene quantification signal, indicating that identification of a chemical group was learned by the model. The sensitivity is slightly lower than for toluene. Releasing isopropanol does not affect any sensor signal, thus the ethanol signal does not represent the quantification of alcohols as a chemical group. Limonene which has no representative from its chemical group inside the calibration does not affect any signal. During the first field test period, we observed a drift of the predicted concentrations, especially for toluene, reaching negative values after some time. By extending the training with the repeated calibration after the field test this drift was corrected for all data, i.e. both the first and second field test period. This means that the model learns to compensate sensor drift and/or selects more stable features for the quantification if drift is included in the calibration. Data evaluation is ongoing, among others more types of machine learning algorithms will be tested and optimization of the calibration (less data samples for calibration and recalibration, transferability between sensors) will be studied. [1] M. Leidinger et al.: Selective detection of hazardous VOCs for indoor air quality applications using a virtual gas sensor array , J. Sens. Sens. Syst., 3 (2014), pp. 253-263, doi:10.5194/jsss-3-253-2014. [2] N. Helwig et al.: Gas mixing apparatus for automated gas sensor characterization , Meas. Sci. Technol. 25 (2014) 055903, doi:10.1088/0957-0233/25/5/055903 [3] D. Rüffner, F. Hoehne, J. Bühler, New Digital Metal-Oxide (MOx) Sensor Platform , Sensors, 18/4 (2018), 1052, doi:10.3390/s18041052. [4] T. Baur et al.: Random gas mixtures for efficient gas sensor calibration , J. Sens. Sens. Syst. (2020) 9, 411-424, doi:10.5194/jsss-9-411-2020. Figure 1
MoreTranslated text
Key words
mos,sensor,gas
AI Read Science
Must-Reading Tree
Example
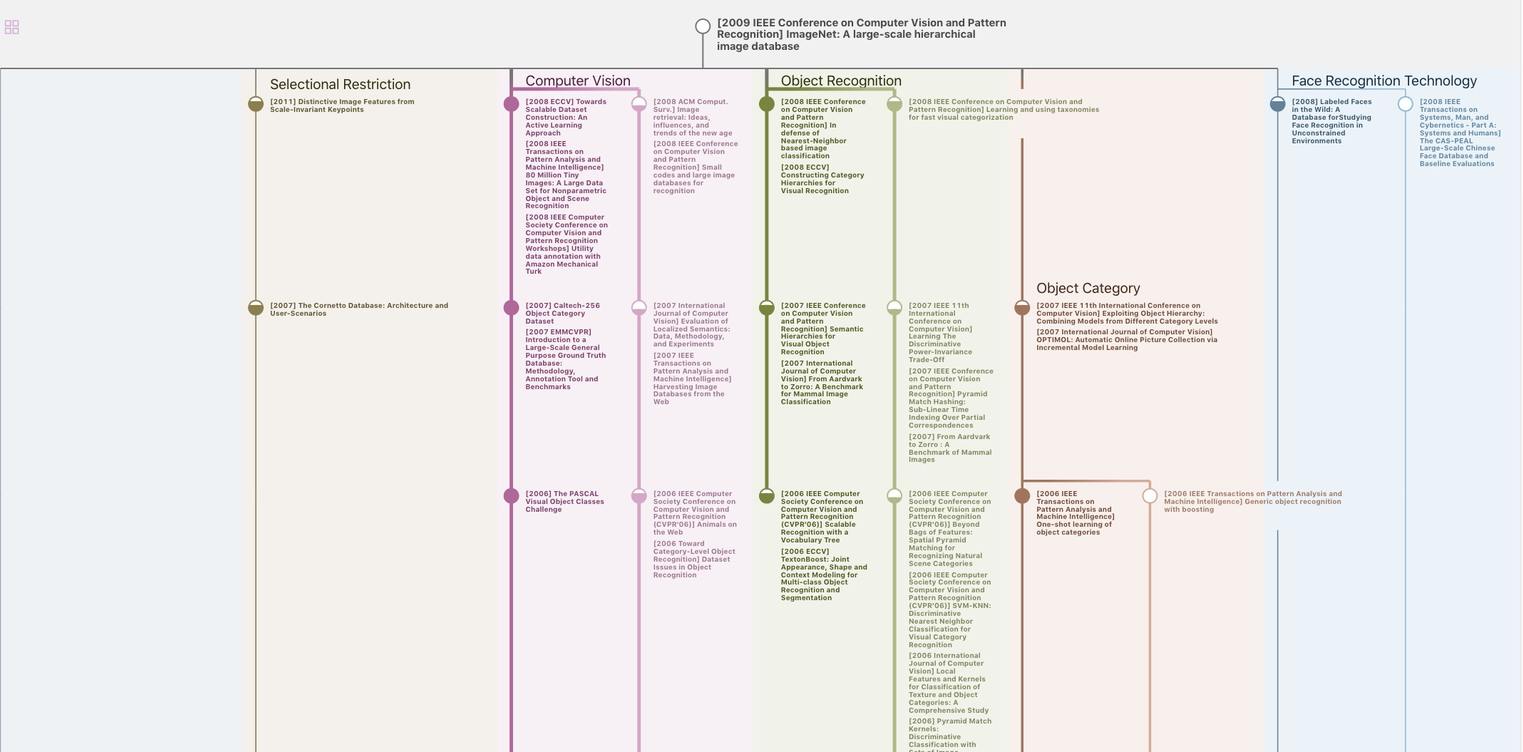
Generate MRT to find the research sequence of this paper
Chat Paper
Summary is being generated by the instructions you defined