'Small Data': Efficient Inference with Occasionally Observed States
Social Science Research Network(2021)
摘要
We study the estimation of dynamic economic models for which some of the state variables are observed only occasionally by the econometrician—a common problem in many fields, ranging from industrial organization to marketing to finance. If such occasional state observations are serially correlated, the likelihood function of the model becomes a potentially high-dimensional integral over a nonstandard domain. We propose a method that generalizes the recursive likelihood function integration procedure (RLI; Reich, 2018) to numerically approximate this integral, and demonstrate its statistical efficiency in several well-understood examples from finance and industrial organization. In extensive Monte Carlo studies, we compare the performance of our approach to a recently suggested method of simulated moments. In all our demonstrations, we consistently and efficiently identify all model parameters, and we find that the additional variance of our estimator when going from full to occasional state observations is small for the parameters of interest.
更多查看译文
AI 理解论文
溯源树
样例
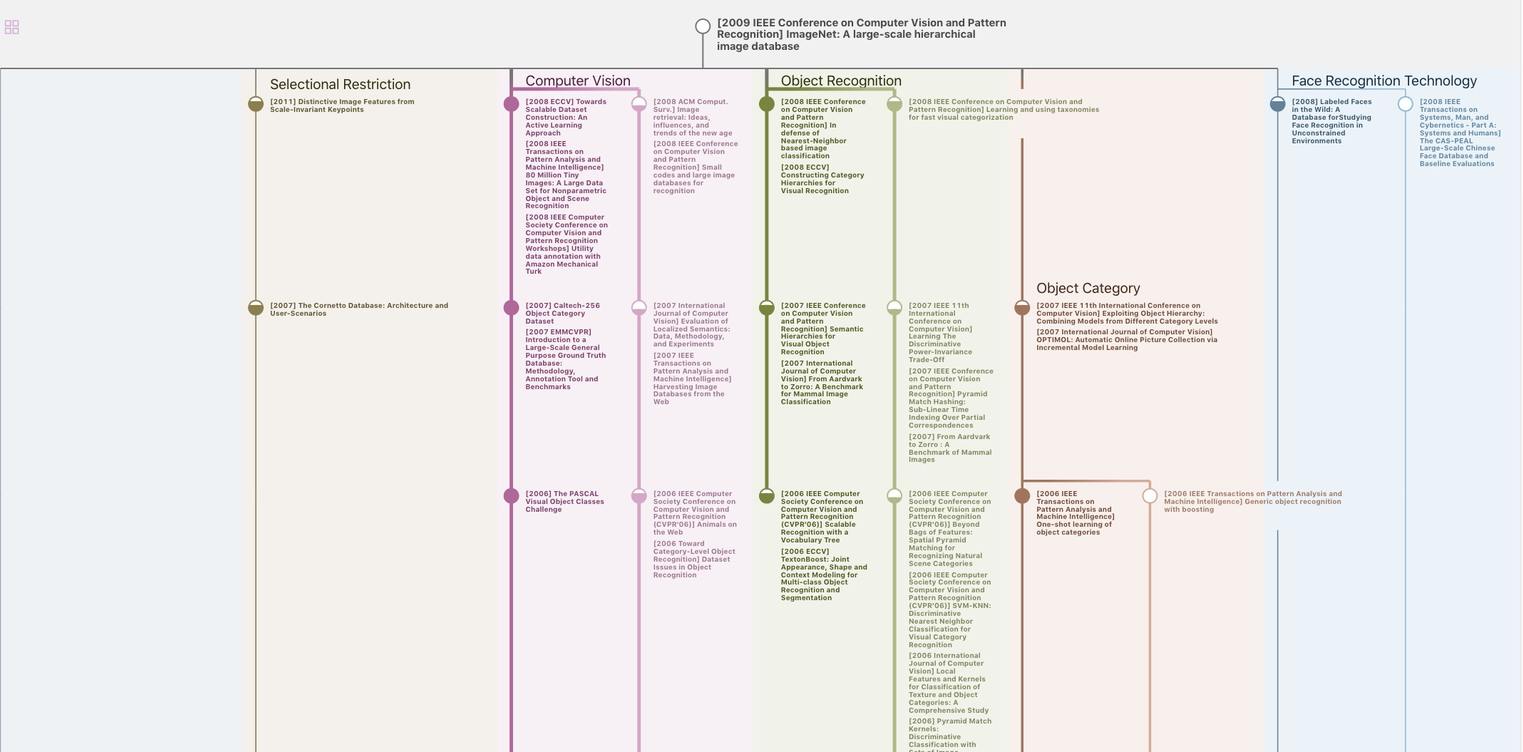
生成溯源树,研究论文发展脉络
Chat Paper
正在生成论文摘要