Upscaling in Situ Site-Based Albedo Using Machine Learning Models: Main Controlling Factors on Results
IEEE TRANSACTIONS ON GEOSCIENCE AND REMOTE SENSING(2022)
摘要
Validation of satellite albedo products is an essential step because their quantitative application lie in their ability to record the real state of the earth surface. Upscaling in situ measurements to the corresponding pixel scale is necessary due to the spatial scale mismatch between in situ and satellite measurements. Machine learning-based models have been increasingly used for upscaling because they can yield more reliable results than traditional methods. Nevertheless, the main controlling factors on upscaled results have rarely been discussed. This article explores the control factors that bring uncertainties to the upscaled results based on machine learning models. Three machine learning models, including random forest (RF), k-nearest neighbor (KNN), and Cubist models, were selected to upscale single site in situ-based albedo to the coarse pixel scale. The upscaled results were carefully assessed through comparison with pixel scale albedo reference. The results indicate that the accuracy of upscaled results depends on the machine learning models, the inclusion of key variables related to albedo, the dataset selection of these variables, the amount of training data, and the sensitivity of machine learning models to these factors. Despite the dependence on control factors, the machine learning-based upscaling methods generally have excellent applicability across different spatial scales and over other untrained areas. Therefore, they open the door to generating a time series of globally, spatially continuous distributed reference datasets with sufficient length, consistency, and continuity to adequately fulfill the requirement of a comprehensive validation.
更多查看译文
关键词
Land surface,Machine learning,Satellites,Biological system modeling,Uncertainty,Rivers,Remote sensing,Albedo,control factors,machine learning method,remote sensing,uncertainty,upscaling,validation
AI 理解论文
溯源树
样例
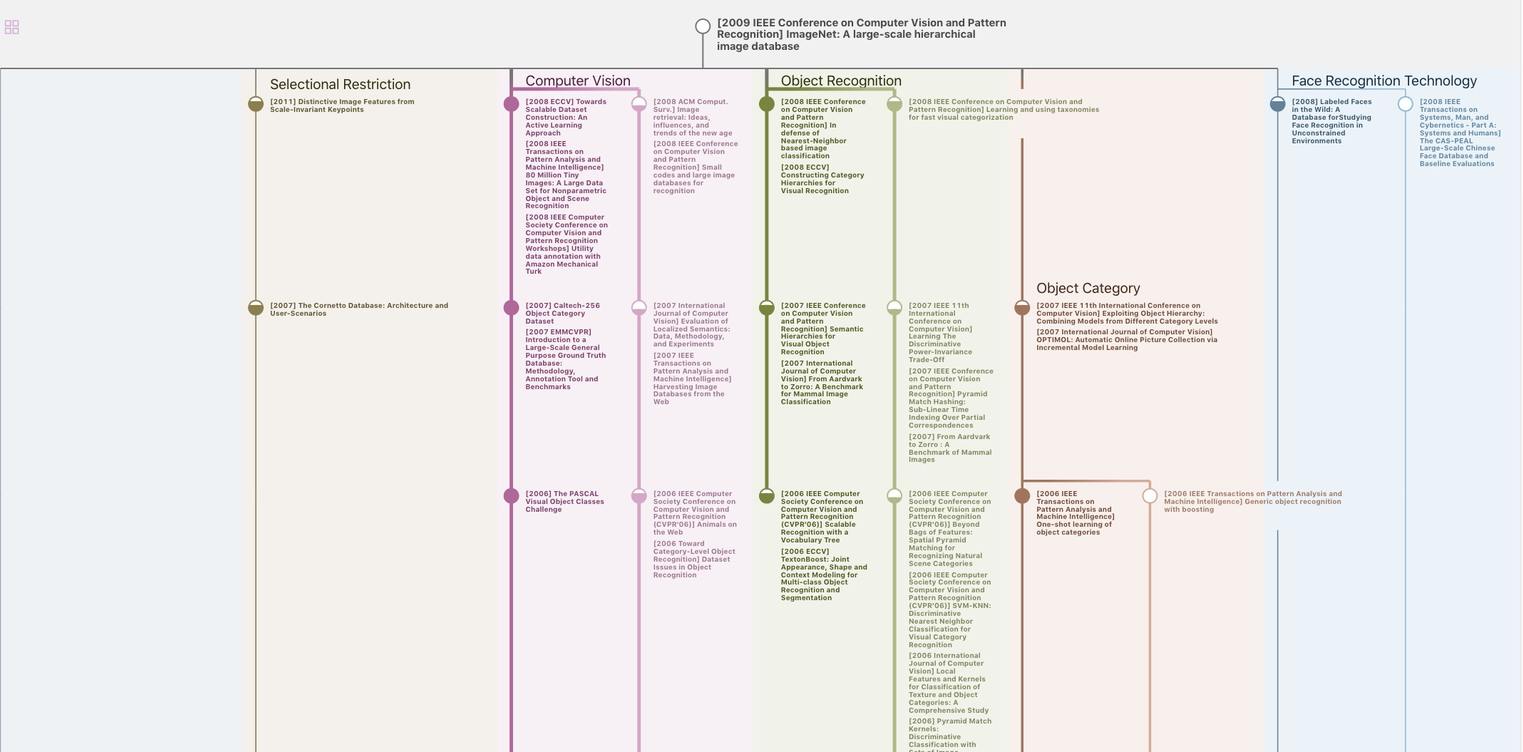
生成溯源树,研究论文发展脉络
Chat Paper
正在生成论文摘要