Forecasting a Nonstationary Time Series with a Mixture of Stationary and Nonstationary Factors as Predictors
Social Science Research Network(2020)
Abstract
This paper develops a method for forecasting a nonstationary time series, such as GDP, using a set of high-dimensional panel data as predictors. To this end, we use what is known as a factor augmented regression [FAR] model that contains a small number of estimated factors as predictors; the factors are estimated using time series data on a large number of potential predictors. The validity of this method for forecasting has been established when all the variables are stationary and also when they are all nonstationary, but not when they consist of a mixture of stationary and nonstationary ones. This paper fills this gap. More specifically, we develop a method for constructing an asymptotically valid prediction interval using the FAR model when the predictors include a mixture of stationary and nonstationary factors; we refer to this as mixture-FAR model. This topic is important because typically time series data on a large number of economic variables is likely to contain a mixture of stationary and nonstationary variables. In a simulation study, we observed that the mixture-FAR performed better than its competitor that requires all the variables to be nonstationary. As an empirical illustration, we evaluated the aforementioned methods for forecasting the nonstationary variables, GDP and Industrial Production [IP], using the quarterly panel data on US macroeconomic variables, known as FRED-QD. We observed that the mixture-FAR model proposed in this paper performed better than its aforementioned competitors.
MoreTranslated text
Key words
Forecast Combination,Forecasting,Demand Forecasting
AI Read Science
Must-Reading Tree
Example
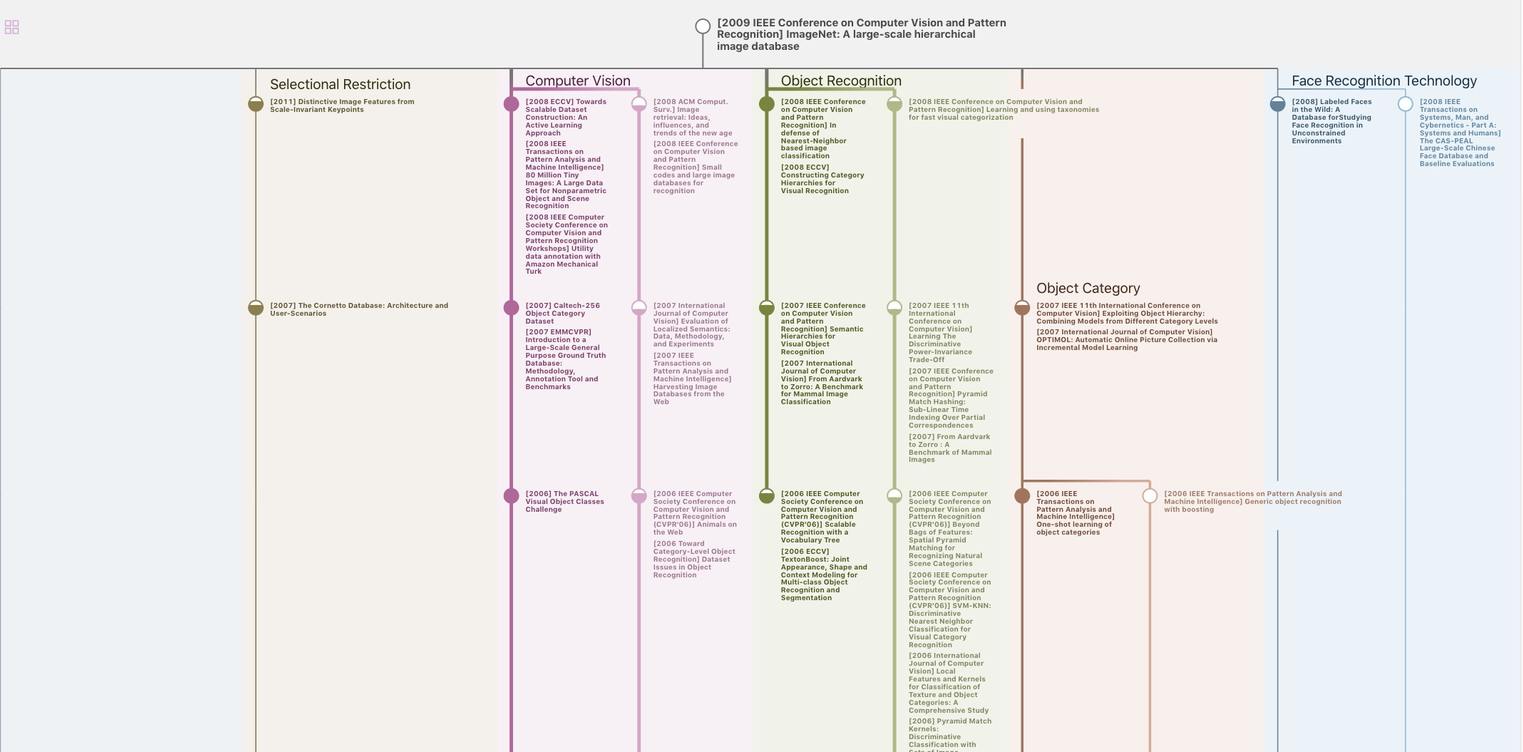
Generate MRT to find the research sequence of this paper
Chat Paper
Summary is being generated by the instructions you defined