Forecasting residential gas consumption with machine learning algorithms on weather data
E3S Web of Conferences(2019)
摘要
Machine learning models have proven to be reliable methods in the forecasting of energy use in commercial and office buildings. However, little research has been done on energy forecasting in dwellings, mainly due to the difficulty of obtaining household level data while keeping the privacy of inhabitants in mind. Gaining insight into the energy consumption in the near future can be helpful in balancing the grid and insights in how to reduce the energy consumption can be received. In collaboration with OPSCHALER, a measurement campaign on the influence of housing characteristics on energy costs and comfort, several machine learning models were compared on forecasting performance and the computational time needed. Nine months of data containing the mean gas consumption of 52 dwellings on a one hour resolution was used for this research. The first 6 months were used for training, whereas the last 3 months were used to evaluate the models. The results showed that the Deep Neural Network (DNN) performed best with a 50.1 % Mean Absolute Percentage Error (MAPE) on a one hour resolution. When comparing daily and weekly resolutions, the Multivariate Linear Regression (MVLR) outperformed other models, with a 20.1 % and 17.0 % MAPE, respectively. The models were programmed in Python.
更多查看译文
关键词
Load Forecasting,Building Energy Consumption,Energy Efficiency,Short-Term Forecasting,Electricity Price Forecasting
AI 理解论文
溯源树
样例
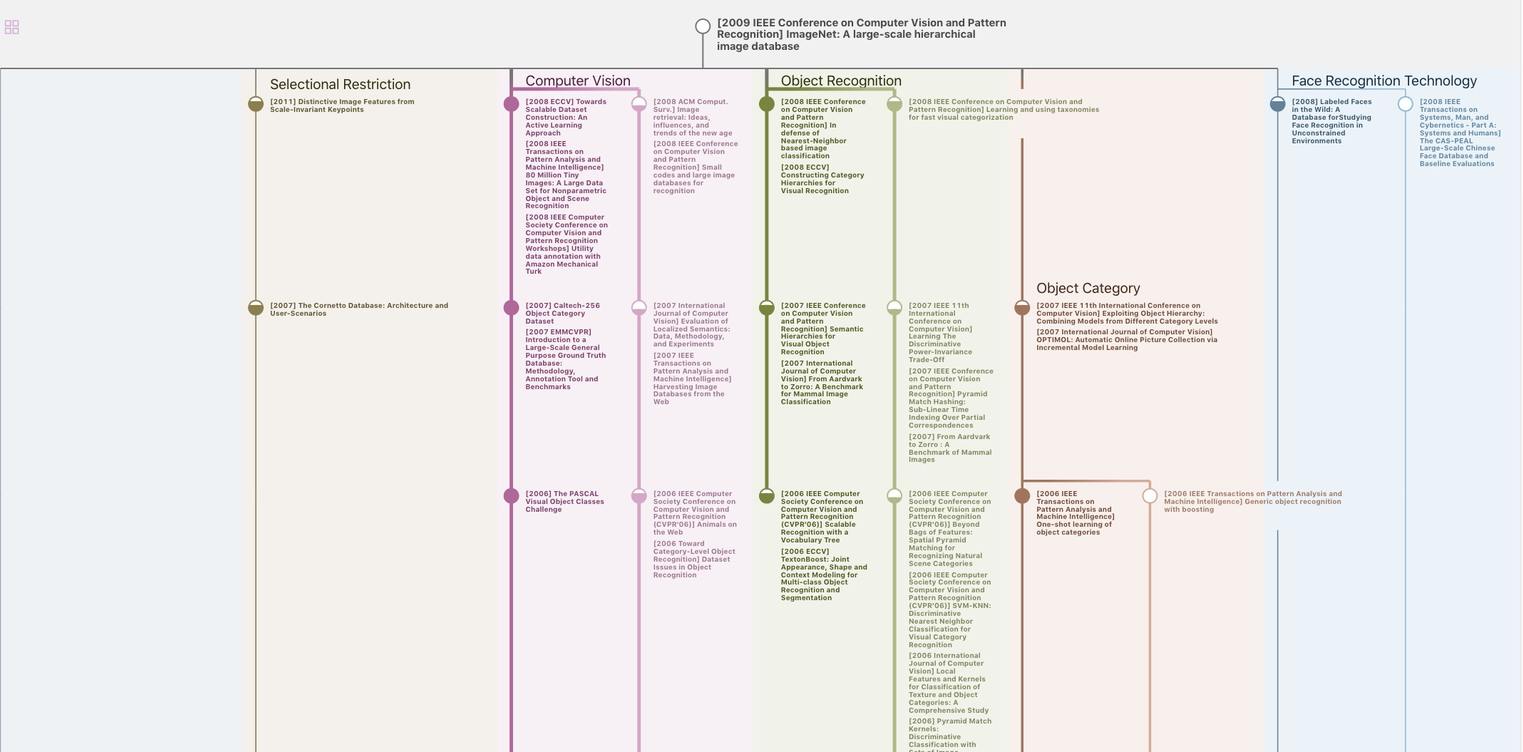
生成溯源树,研究论文发展脉络
Chat Paper
正在生成论文摘要