Terminal Temperature Prediction of Molten Steel in LF Furnace based on Stacking Model Fusion
2021 IEEE 10th Data Driven Control and Learning Systems Conference (DDCLS)(2021)
摘要
Ladle Furnace (LF) is a kind of refining equipment outside the furnace, and the end temperature of its molten steel is an important factor affecting the quality of later finished steel. Aiming at the characteristic that it is difficult to continuously measure the temperature of molten steel in LF, based on the field data of Baosteel's LF furnace, a two-layer structure Stacking integrated model framework is designed, the first layer contains four base learners: ridge regression algorithm, support vector regression, random forest, and XGBoost, the second layer uses the XGBoost model. Based on five modeling methods of ridge regression algorithm, support vector regression, random forest, XGBoost and Stacking designed, after analyzing and selecting the main factors affecting the LF temperature, the prediction models of the LF furnace molten steel endpoint temperature were established. The experimental results show that compared with a single model, the prediction effect and stability of the Stacking model combining ridge regression algorithm, support vector regression, random forest, and XGBoost have been significantly improved. The prediction model can not only reflect the effect of different factors on LF, but also, it can predict the end temperature of LF molten steel relatively accurately. The prediction accuracy of the end temperature of molten steel at ± 10°C can reach more than 80%.
更多查看译文
关键词
LF,ridge regression,SVR,random forest,XGBoost,Stacking,prediction
AI 理解论文
溯源树
样例
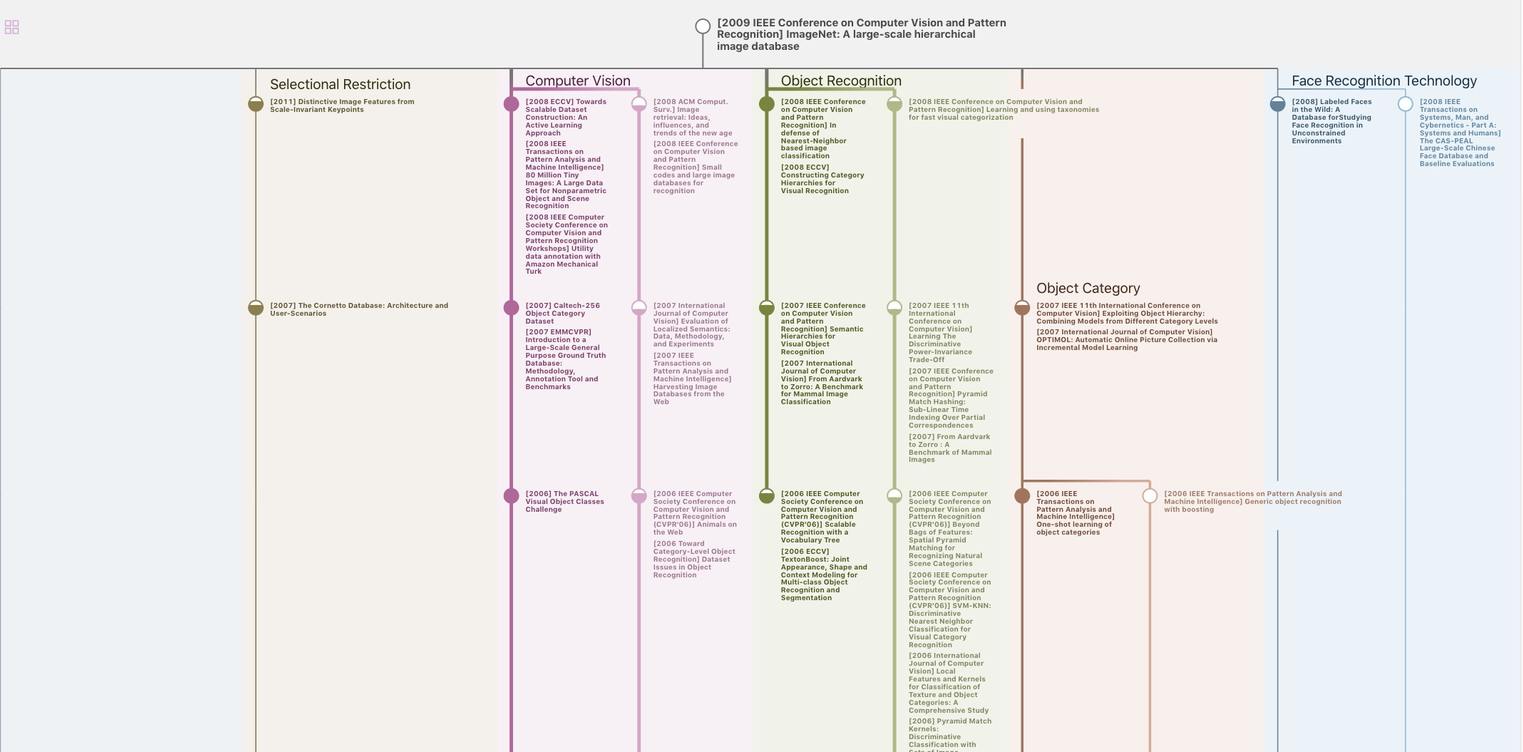
生成溯源树,研究论文发展脉络
Chat Paper
正在生成论文摘要