EAPT: Efficient Attention Pyramid Transformer for Image Processing
IEEE TRANSACTIONS ON MULTIMEDIA(2023)
摘要
Recent transformer-based models, especially patch-based methods, have shown huge potentiality in vision tasks. However, the split fixed-size patches divide the input features into the same size patches, which ignores the fact that vision elements are often various and thus may destroy the semantic information. Also, the vanilla patch-based transformer cannot guarantee the information communication between patches, which will prevent the extraction of attention information with a global view. To circumvent those problems, we propose an Efficient Attention Pyramid Transformer (EAPT). Specifically, we first propose the Deformable Attention, which learns an offset for each position in patches. Thus, even with split fixed-size patches, our method can still obtain non-fixed attention information that can cover various vision elements. Then, we design the Encode-Decode Communication module (En-DeC module), which can obtain communication information among all patches to get more complete global attention information. Finally, we propose a position encoding specifically for vision transformers, which can be used for patches of any dimension and any length. Extensive experiments on the vision tasks of image classification, object detection, and semantic segmentation demonstrate the effectiveness of our proposed model. Furthermore, we also conduct rigorous ablation studies to evaluate the key components of the proposed structure.
更多查看译文
关键词
Transformers,attention mechanism,pyramid,classification,object detection,semantic segmentation
AI 理解论文
溯源树
样例
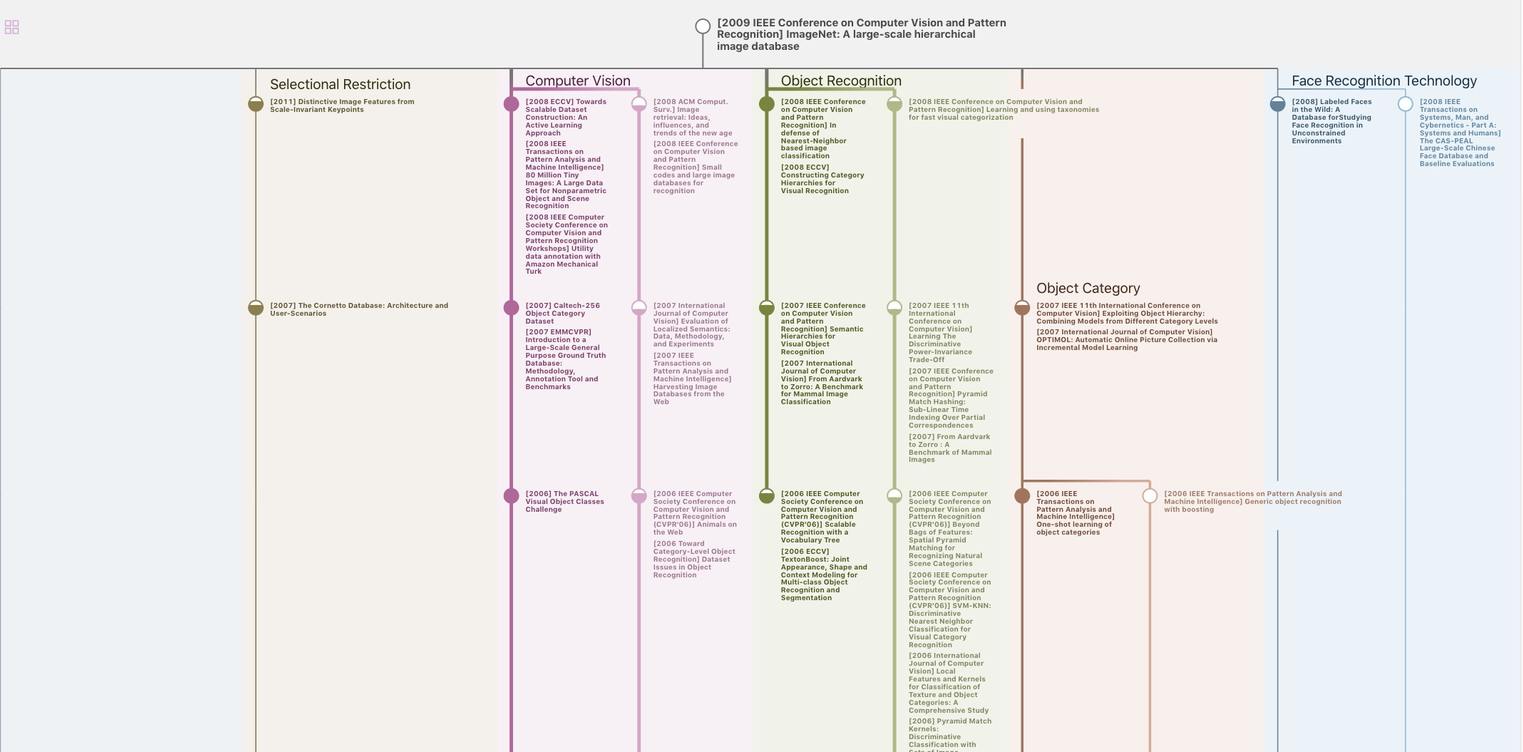
生成溯源树,研究论文发展脉络
Chat Paper
正在生成论文摘要