CNGAT: A Graph Neural Network Model for Radar Quantitative Precipitation Estimation
IEEE TRANSACTIONS ON GEOSCIENCE AND REMOTE SENSING(2022)
摘要
Radar quantitative precipitation estimation (RQPE) is the most common measurement for area rainfall estimation with high spatial and temporal resolution. The radar reflectivity (Z) measured by the Doppler weather radar is strongly related to precipitation rate (R). However, conventional RQPE methods have limited capability of modeling the complex relationship between the radar echoes and the precipitation field. In this article, we propose a graph neural network (GNN)-based RQPE model named categorical node graph attention network (CNGAT) to model complex spatial-temporal features of precipitation field reflected by the radar echo field. CNGAT is derived from graph attention networks (GAT) utilizing attention mechanism to learn the importance of neighboring points to the central point, which is beneficial for learning varying local spatial patterns. Furthermore, CNGAT can handle multiple types of graph nodes by using different transform functions for different types of nodes, which makes it better at capturing diverse features of precipitation field indicated by strong and weak radar echo areas. The proposed model was trained and tested on radar network and rain gauge data distributed in East China during 2017 and 2018. The results of several experiments show that CNGAT greatly improves the estimation precision and detection rate than Z-R relation models and conventional data-driven RQPE methods, and alleviates under-estimation of higher precipitation rates, which validates that CNGAT can effectively represent complex spatial-temporal features of precipitation field.
更多查看译文
关键词
Graph convolution,graph neural network (GNN),radar quantitative precipitation estimation (RQPE),Z-R relationship
AI 理解论文
溯源树
样例
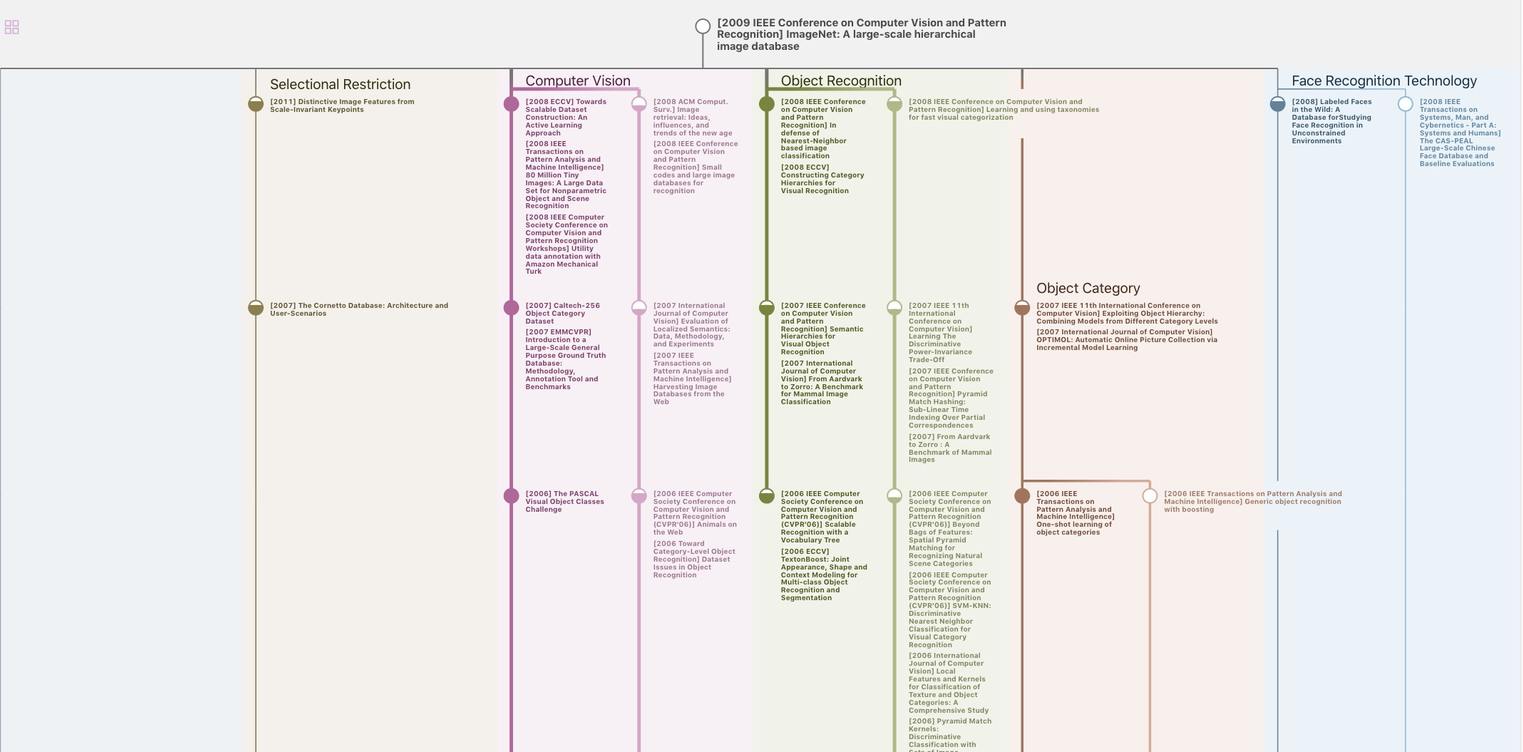
生成溯源树,研究论文发展脉络
Chat Paper
正在生成论文摘要