Lessons from the COVID-19 pandemic for advancing computational drug repurposing strategies
Nature Computational Science(2021)
摘要
Responding quickly to unknown pathogens is crucial to stop uncontrolled spread of diseases that lead to epidemics, such as the novel coronavirus, and to keep protective measures at a level that causes as little social and economic harm as possible. This can be achieved through computational approaches that significantly speed up drug discovery. A powerful approach is to restrict the search to existing drugs through drug repurposing, which can vastly accelerate the usually long approval process. In this Review, we examine a representative set of currently used computational approaches to identify repurposable drugs for COVID-19, as well as their underlying data resources. Furthermore, we compare drug candidates predicted by computational methods to drugs being assessed by clinical trials. Finally, we discuss lessons learned from the reviewed research efforts, including how to successfully connect computational approaches with experimental studies, and propose a unified drug repurposing strategy for better preparedness in the case of future outbreaks. Computational approaches for drug repurposing can accelerate the identification of treatments during a pandemic. In this Review, the authors discuss this topic in the context of COVID-19 and propose a strategy to make computational drug repurposing more effective in future pandemics.
更多查看译文
关键词
Drug discovery,High-throughput screening,Virtual drug screening,Computer Science,general
AI 理解论文
溯源树
样例
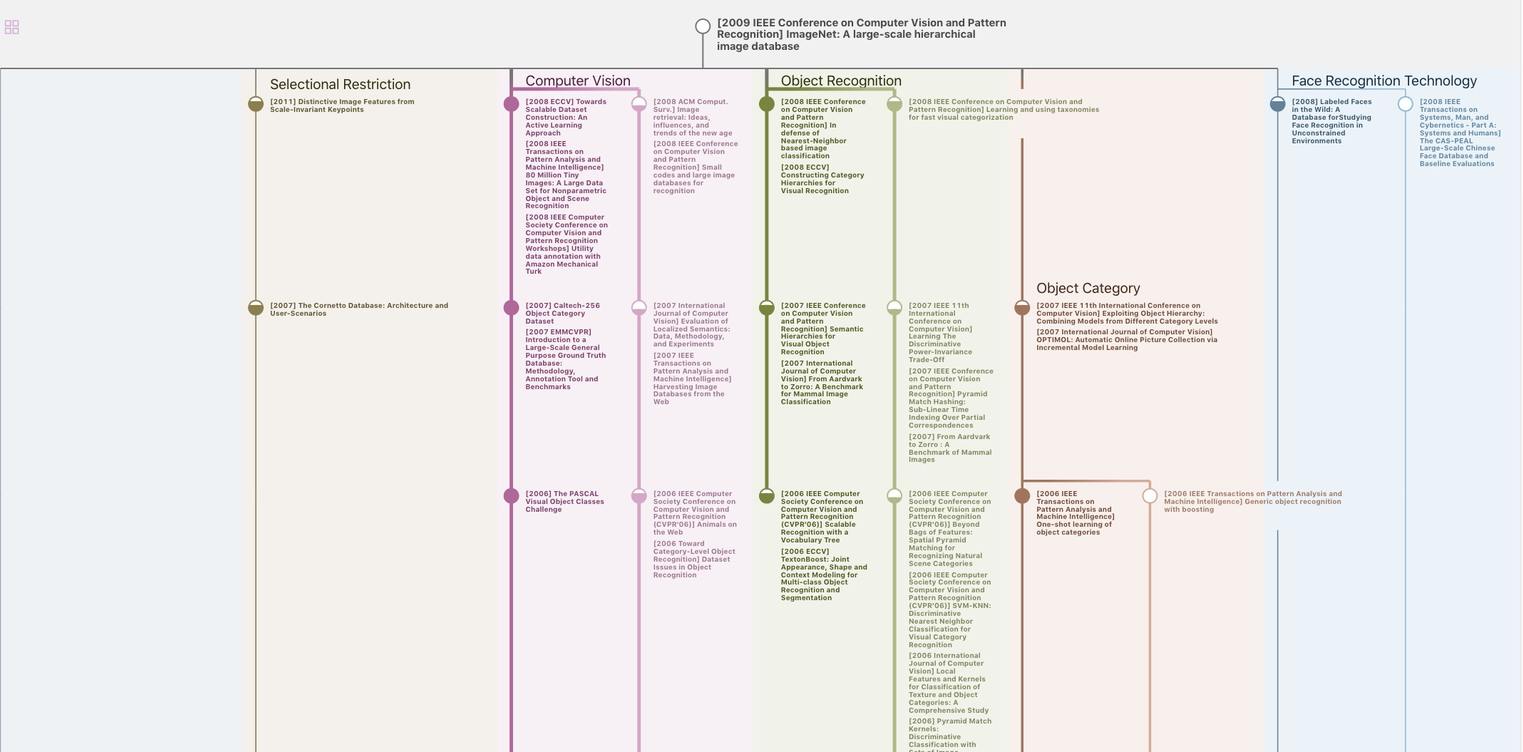
生成溯源树,研究论文发展脉络
Chat Paper
正在生成论文摘要