A deep learning approach for joint inversion of DC Resistivity and MT data
82nd EAGE Annual Conference & Exhibition(2021)
Abstract
Summary Joint inversion of multiple geophysical datasets has its own set of advantages for interpreting the geology of an area. Using neural networks (NN), we propose the joint inversion of MT and DC apparent resistivity datasets to delineate the subsurface conductivity distribution. The NN model used is inspired by the Siamese networks to provide different prediction channels for the two different datasets before integrating them to get the layered earth parameters. The NN model trained on the specified range of model parameters has predicted each layers’ resistivity and thickness close to the true values for all the four types of resistivity distribution (A, Q, H, and K) for a three-layered earth model and takes advantage of the two different datasets to see through the equivalence problem to detect the thin second layer for an H type curve. The NN model accurately estimated the resistivity distribution even when the true data was corrupted with 10% Gaussian noise. Not only the method proposed provides good results for all the models considered but also saves time over other optimisation techniques where every model requires separate simulation. The method, therefore, proves to be a fast, efficient and reliable way for joint inversion of geophysical datasets.
MoreTranslated text
Key words
dc resistivity,deep learning approach,joint inversion
AI Read Science
Must-Reading Tree
Example
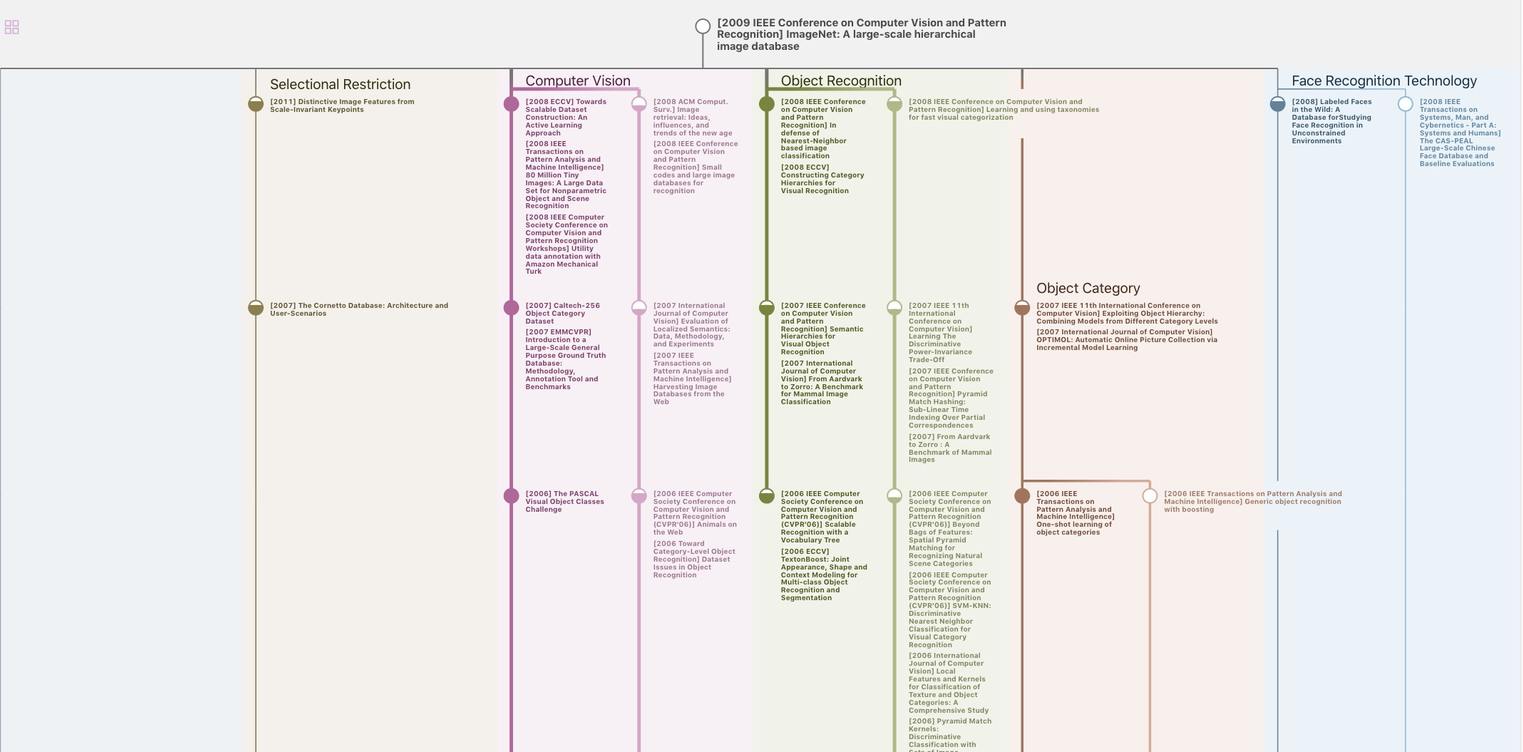
Generate MRT to find the research sequence of this paper
Chat Paper
Summary is being generated by the instructions you defined