Estimating nosocomial infection and its outcomes in hospital patients in England with a diagnosis of COVID-19 using machine learning
Social Science Research Network(2023)
摘要
Our aim was to provide a comprehensive account of COVID-19 nosocomial infections (NIs) in England and identify their characteristics and outcomes using machine learning. From the Hospital Episodes Statistics database, 374,244 adult hospital patients in England with a diagnosis of COVID-19 and discharged between March 1, 2020, and March 31, 2021, were identified. A cohort of suspected COVID-19 NIs was identified using four empirical methods linked to hospital coding. A random forest classifier was designed to model the characteristics of these infections. The model estimated a mean NI rate of 10.5%, with a peak close to 18% during the first wave, but much lower rates (7%) thereafter. NIs were highly correlated with longer lengths of stay, high trust capacity strain, greater age and a higher degree of patient frailty, and associated with higher mortality rates and more severe COVID-19 sequelae, including pneumonia, kidney disease and sepsis. Identification of the characteristics of patients who acquire NIs should help trusts to identify those most at risk. The evolution of the NI rate over time may reflect the impact of changes in hospital management practises and vaccination efforts. Variations in NI rates across trusts may partly reflect different data recording and coding practise.
更多查看译文
关键词
COVID-19,Coronavirus,Mortality,Hospital acquired infection,Nosocomial infection
AI 理解论文
溯源树
样例
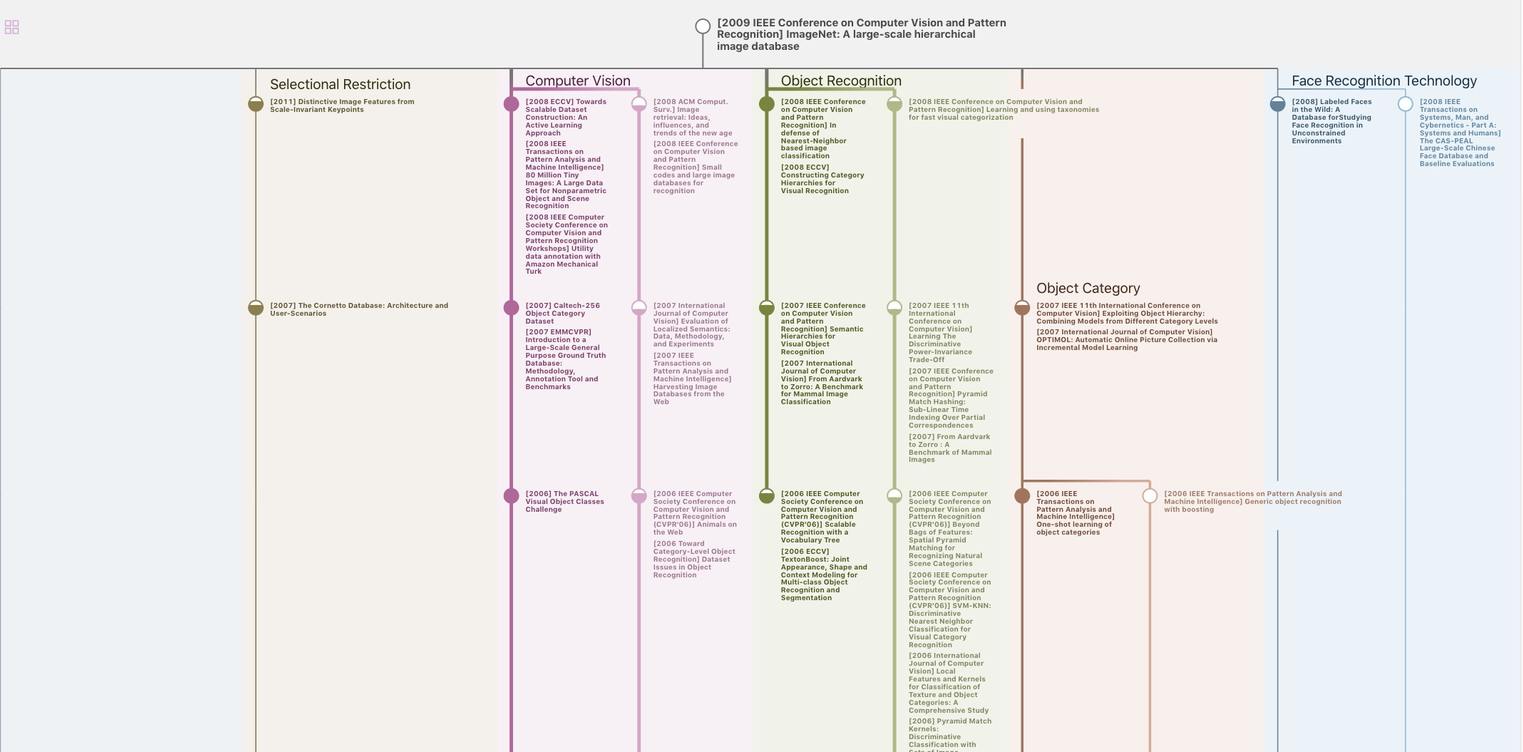
生成溯源树,研究论文发展脉络
Chat Paper
正在生成论文摘要