Development of FPGA-based neural network regression models for the ATLAS Phase-II barrel muon trigger upgrade
Epj Web of Conferences(2021)
摘要
Effective selection of muon candidates is the cornerstone of the LHC physics programme. The ATLAS experiment uses a two-level trigger system for real-time selection of interesting collision events. The first-level hardware trigger system uses the Resistive Plate Chamber detector (RPC) for selecting muon candidates in the central (barrel) region of the detector. With the planned upgrades, the entirely new FPGA-based muon trigger system will be installed in 2025-2026. In this paper, neural network regression models are studied for potential applications in the new RPC trigger system. A simple simulation model of the current detector is developed for training and testing neural network regression models. Effects from additional cluster hits and noise hits are evaluated. Efficiency of selecting muon candidates is estimated as a function of the transverse muon momentum. Several models are evaluated and their performance is compared to that of the current detector, showing promising potential to improve on current algorithms for the ATLAS Phase-II barrel muon trigger upgrade.
更多查看译文
关键词
neural network regression models,neural network,fpga-based
AI 理解论文
溯源树
样例
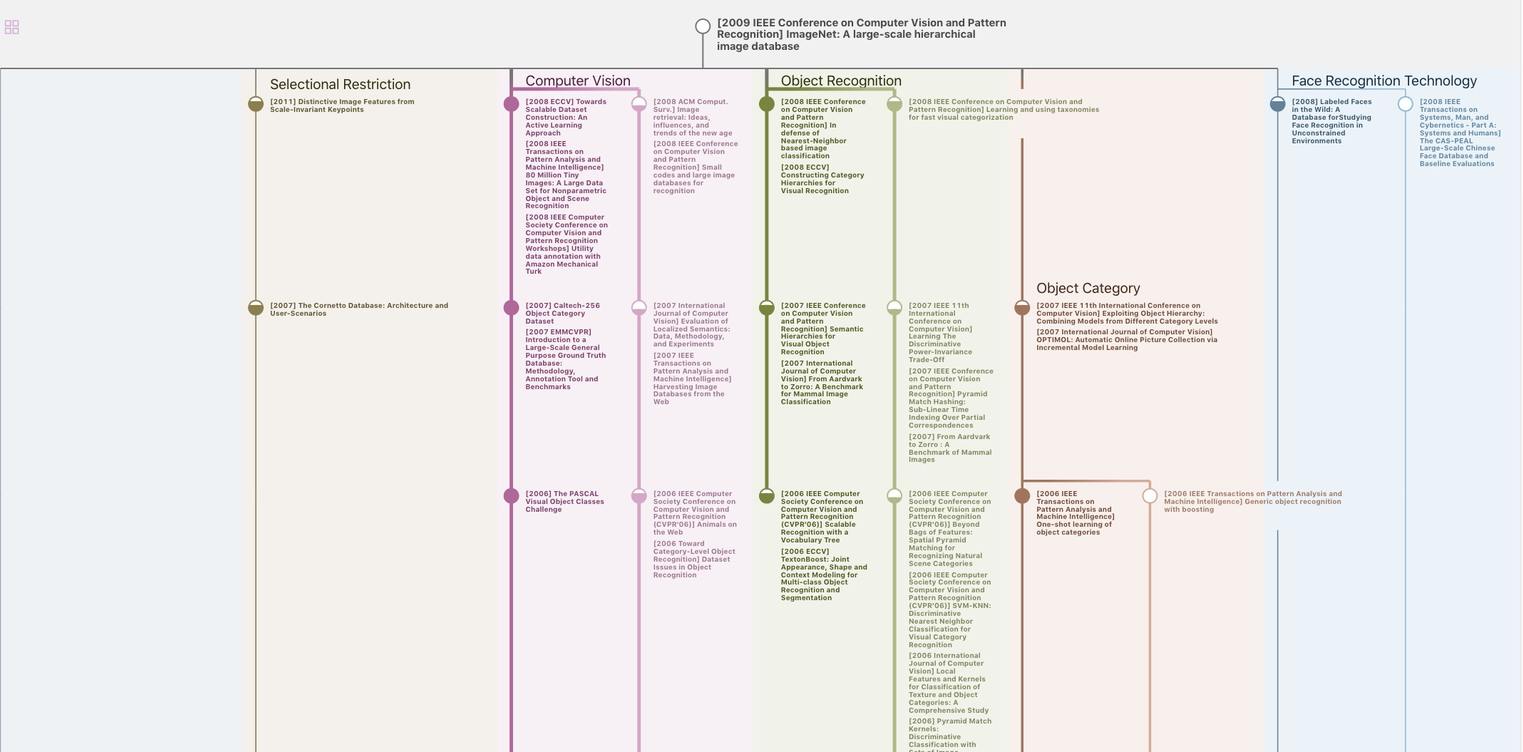
生成溯源树,研究论文发展脉络
Chat Paper
正在生成论文摘要