Bridging Truthfulness and Corruption-Robustness in Multi-Armed Bandit Mechanisms
international conference on machine learning(2020)
摘要
We study pay-per-click auctions where both the principal and the agents employ learning algorithms to learn the click-through-rates and intrinsic values respectively. In this setting, we illustrate a trade-off between a) effective learning in a truthful manner and b) robustness to the presence of adversarial corruptions. We design a mechanism that balances these two conflicting forces and achieves a graceful degradation in performance with the amount of corruption in the data without compromising the performance when there is no corruption. On the way we demonstrate that agent-learning introduces additional challenges in multi-armed bandit mechanisms even at the absence of corruptions, which may be of independent interest.
更多查看译文
AI 理解论文
溯源树
样例
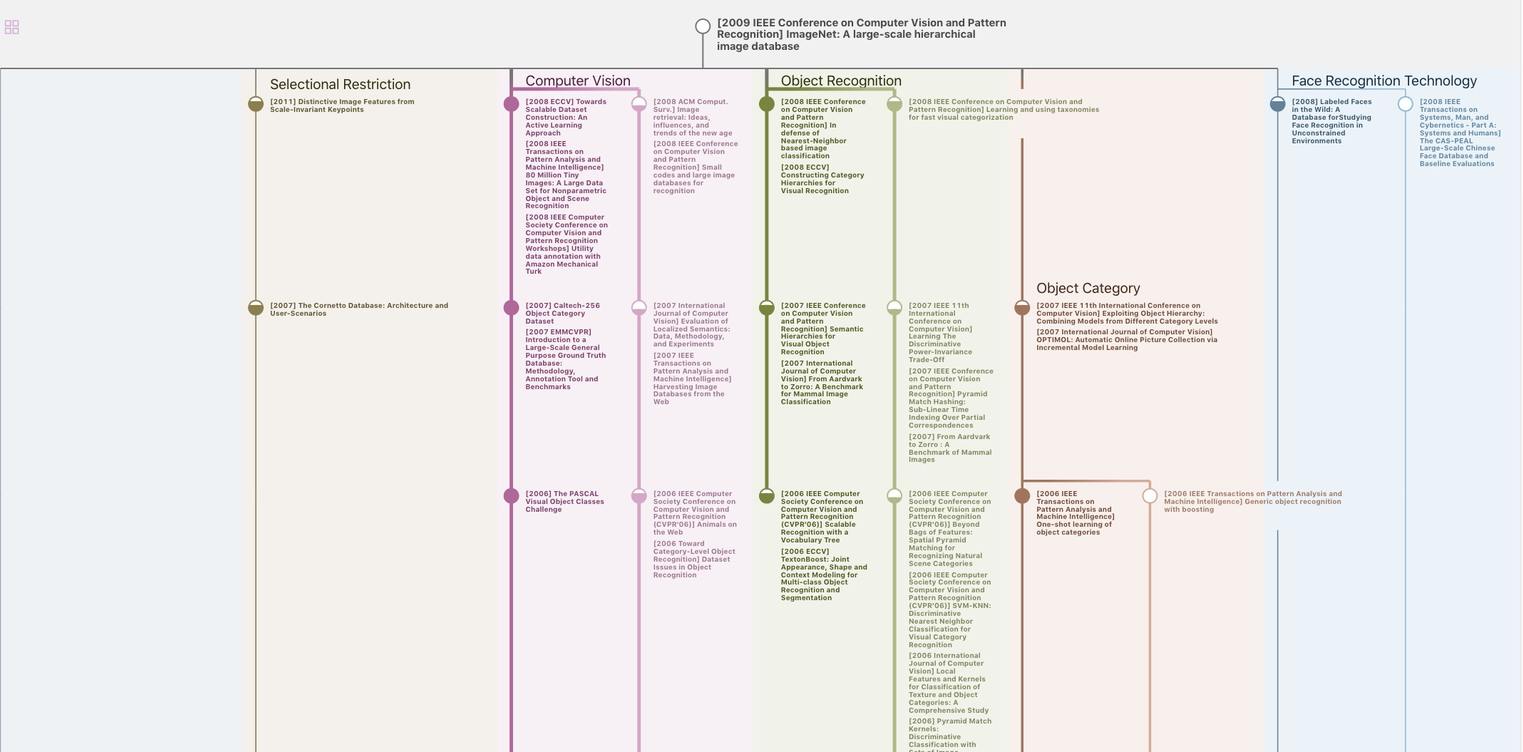
生成溯源树,研究论文发展脉络
Chat Paper
正在生成论文摘要