A hybrid tensor factorization approach for QoS prediction in time-aware mobile edge computing
Applied Intelligence(2021)
摘要
Withthe rapid development of mobile Internet and wireless technologies, edge servers in mobile edge computing (MEC) provide a large number of mobile services to develop advanced and complex mobile applications. Quality of Service (QoS) is an essential indicator for recommendation and selection from such a huge number of candidate mobile services. However, QoS records are sparse in reality because users only invoke certain mobile services. How to predict missing QoS values is a key issue in operations related to mobile services. Some collaborative filtering (CF) methods have been used to solve QoS prediction problem of traditional Web services. Neverless, the high dimensionality, high sparsity and dynamic variability of QoS data in MEC limit the application of these methods. This paper proposes a temporal regularized tensor factorization method based on hybrid CF for QoS prediction of mobile services. The overall structure of QoS data is represented by constructing a user-service-time tensor model. As QoS data possesses certain characteristics of time series, a temporal regular term is introduced into CP decomposition to mine the relationship between QoS values in adjacent time intervals. The alternating least square (ALS) algorithm is used to optimize the approximate tensor iteratively. The low-rank estimation of the target tensor is obtaind to predict missing values. Extensive experimental studies on the large-scale QoS dataset WS-Dream show that our method has significantly better prediction accuracy than the other nine state-of-the-art methods under different data densities. Especially in the case of sparse data, the prediction effect is more prominent, and the accuracy increases up to 62.4 % at most.
更多查看译文
关键词
QoS prediction, Tensor factorization, Time series, Collaborative filtering, Mobile edge computing
AI 理解论文
溯源树
样例
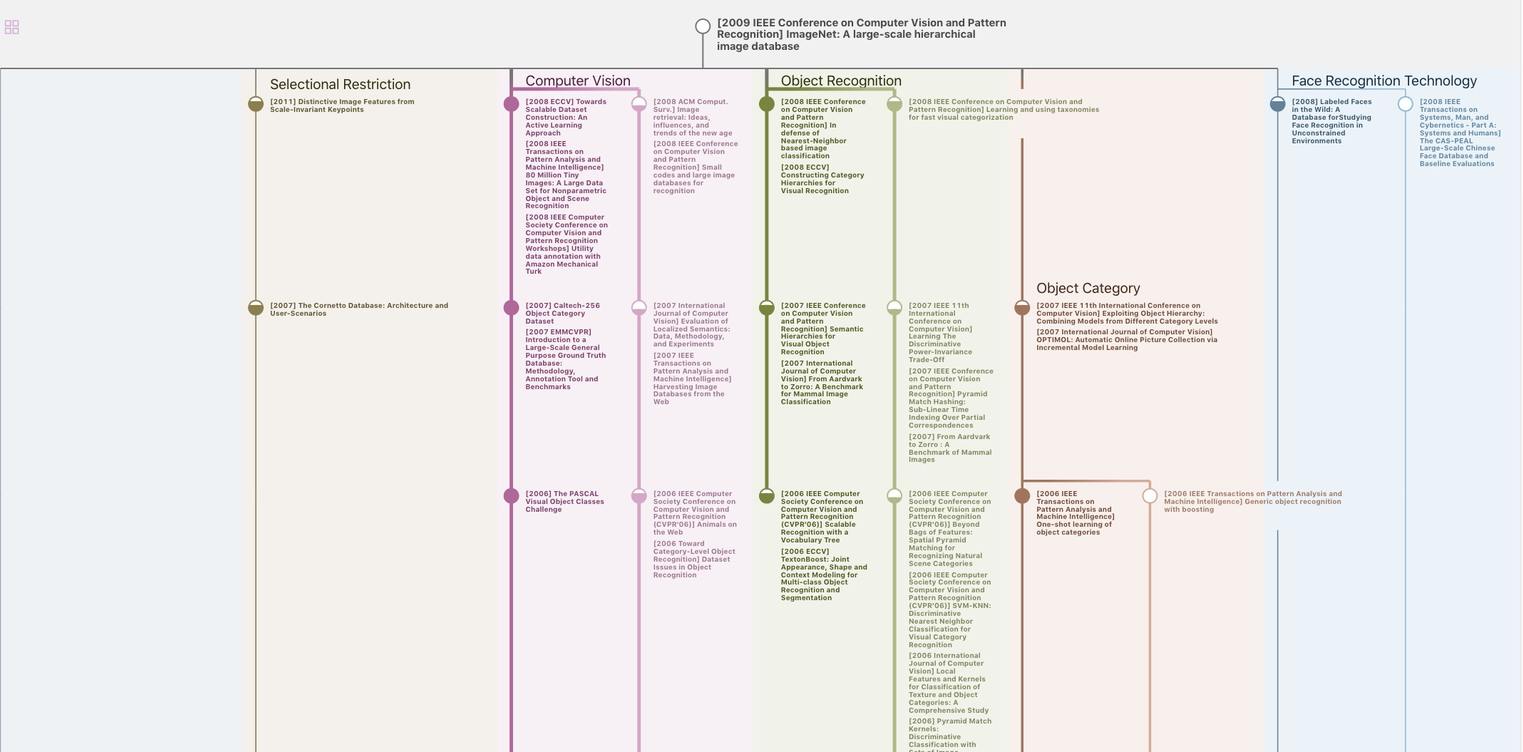
生成溯源树,研究论文发展脉络
Chat Paper
正在生成论文摘要