Adversarial Immunization for Improving Certifiable Robustness on Graphs
web search and data mining(2021)
摘要
Despite achieving strong performance in the semi-supervised node classification task, graph neural networks (GNNs) are vulnerable to adversarial attacks, similar to other deep learning models. Existing research works either focus on developing robust GNN models or attack detection methods against attacks on graphs. However, little research attention is paid to the potential and practice of immunization to adversarial attacks on graphs. In this paper, we formulate the problem of graph adversarial immunization as a bilevel optimization problem, i.e., vaccinating an affordable fraction of node pairs, connected or unconnected, to improve the certifiable robustness of the graph against any admissible adversarial attack. We further propose an efficient algorithm, called AdvImmune, which optimizes meta-gradient in a discrete way to circumvent the computationally expensive combinatorial optimization when solving the adversarial immunization problem. Experiments are conducted on two citation networks and one social network. Experimental results demonstrate that the proposed AdvImmune immunization method remarkably improves the fraction of robust nodes by 12%, 42%, 65%, with an affordable immune budget of only 5% edges.
更多查看译文
AI 理解论文
溯源树
样例
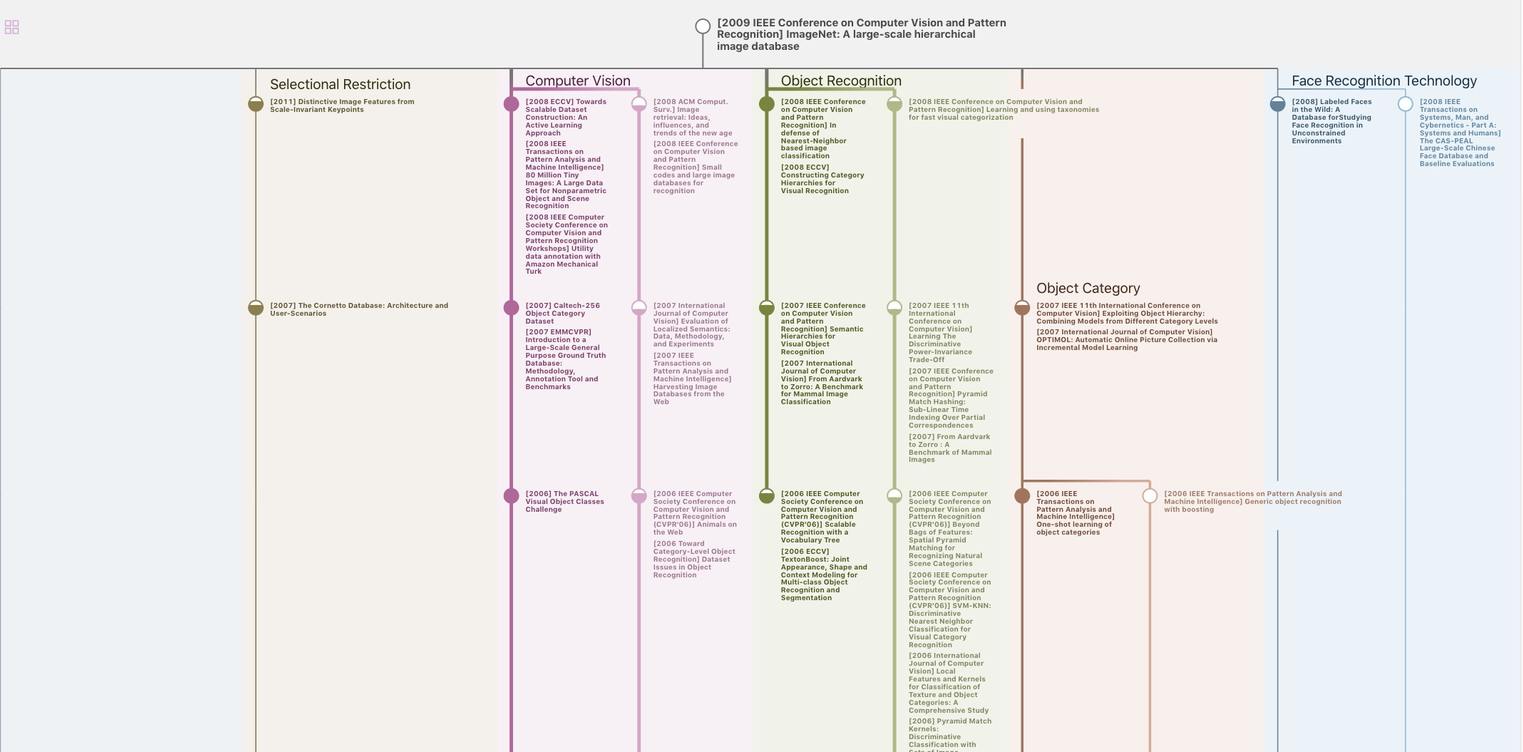
生成溯源树,研究论文发展脉络
Chat Paper
正在生成论文摘要