Wind Power Time Series Missing Data Imputation Based on Generative Adversarial Network
2021 IEEE 4th International Electrical and Energy Conference (CIEEC)(2021)
摘要
With the large scale integration of wind farms, wind power prediction with high accuracy becomes more important. Many research focuses on developing advanced and complex methods to improve the prediction accuracy based on statistical learning, which is highly dependent on the data set. But due to the wind curtailment or the equipment fault, there are some missing data in the wind power time series and enhancing the data quality of training data is crucial. Most research about wind power imputation is based on the power and wind speed curve. However, for some wind farms and the dispatching center, there are no complete wind speed data available. Therefore, we developed a generative adversarial network (GAN) based method for the wind power time series correction. When there is no wind speed measurement, it still can impute wind power. Besides, it can take into consideration the wind power distribution when imputing the wind power time series. Compare to the traditional way such as mean value filled method and other machine learning methods, it has higher imputation accuracy. Case studies on the field wind farm data showed that it outperformed other classical imputation methods.
更多查看译文
关键词
Wind Power Imputation,wind power prediction,generativee adversarial network,machine learning
AI 理解论文
溯源树
样例
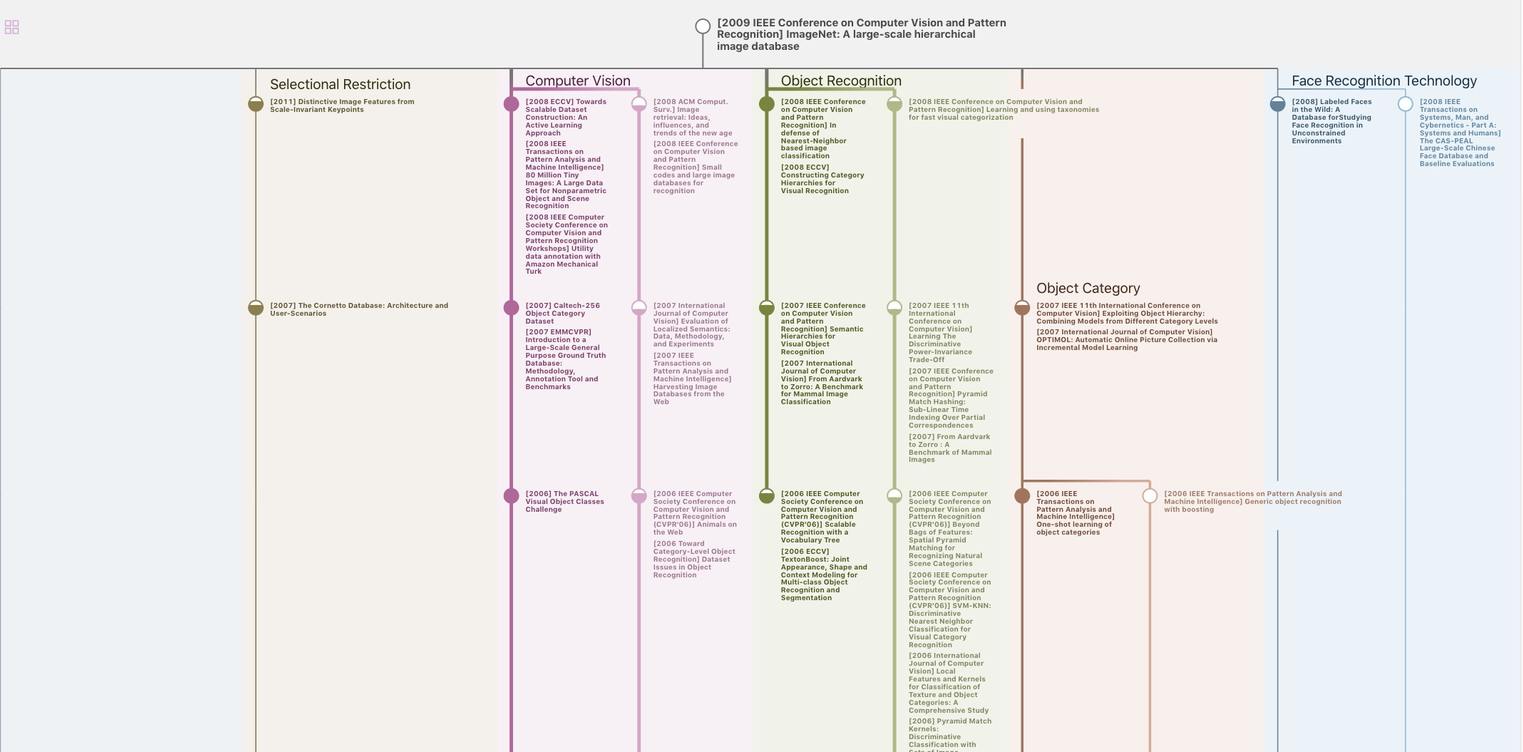
生成溯源树,研究论文发展脉络
Chat Paper
正在生成论文摘要