Detección de fallas en vehículos aéreos no tripulados mediante señales de orientación y técnicas de aprendizaje de máquina
Revista Iberoamericana De Automatica E Informatica Industrial(2021)
摘要
This work proposes an actuator fault detection and isolation scheme for a quadrotor unmanned aerial vehicle (UAV) under a data-driven approach using machine learning techniques. In this approach, an implicit model of the system is built through the information provided by the onboard sensors of the UAV. First, using a tailored flying platform, vibrations corresponding to the orientation, angular position and linear acceleration were captured with the UAV flying in hover mode under nominal conditions. This data is processed by Principal Component Analysis (PCA) for feature extraction. Subsequently, faults in the actuators are induced through a cut in each of the UAV propellers which generate a reduction in the thrust of the rotors. These data are also projected into the PCA subspace and compared to the nominal data. Hotelling’s T 2 statistic is used to discern between nominal data and data when the vehicle exhibits an actuator fault. Finally, the developed algorithms were complemented with k-nearest neighbors (k-NN) and support vector machine (SVM) classification algorithms. The results show a correct classification rate of 89.6 % (k-NN) and 92.4 % (SVM) respectively for 423 validation datasets.
更多查看译文
关键词
vehículo aéreo no tripulado,detección e identificación de fallas,análisis en componentes principales,aprendizaje de máquina,cuadrirrotor
AI 理解论文
溯源树
样例
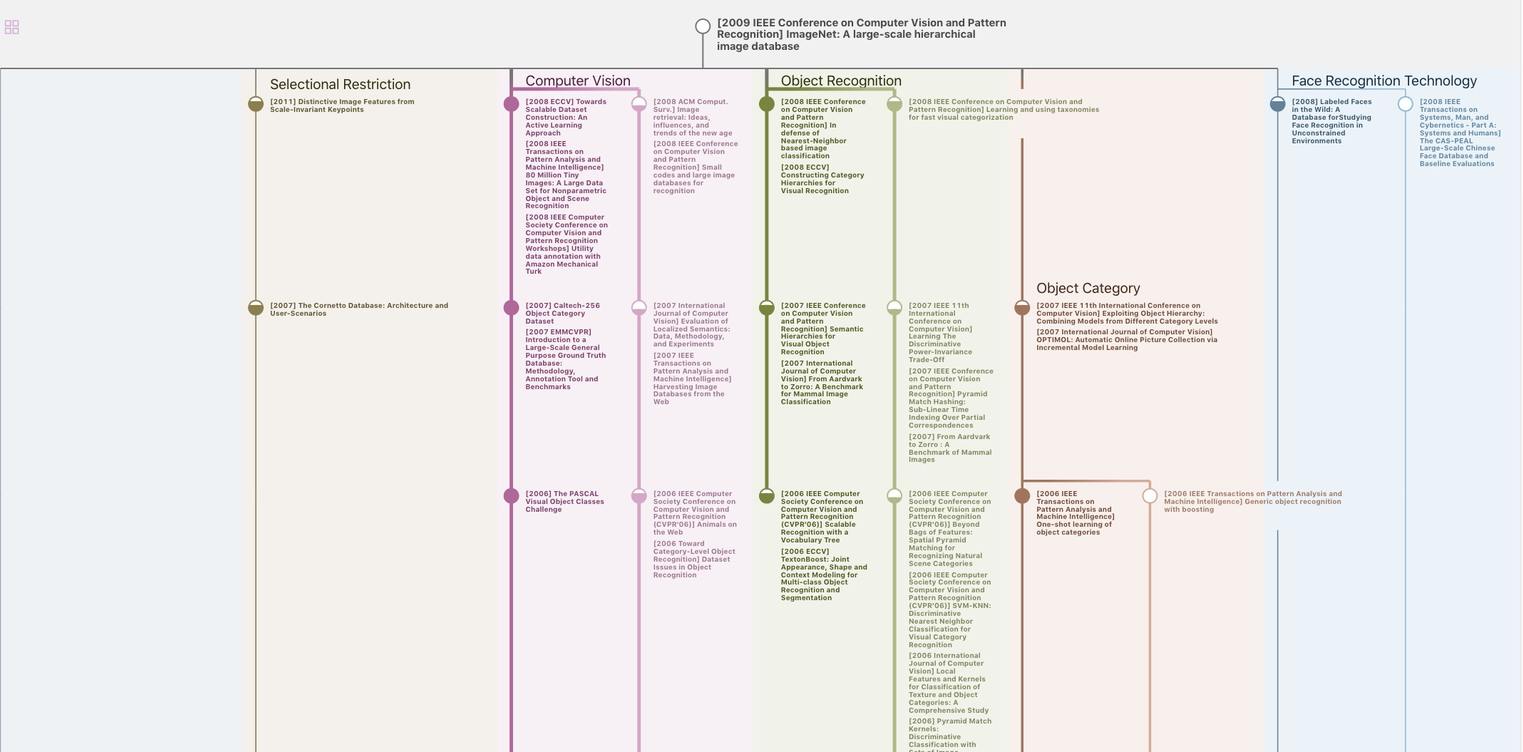
生成溯源树,研究论文发展脉络
Chat Paper
正在生成论文摘要