Impact of Adversarial Training on Robustness and Generalizability of Language Models
conf_acl(2022)
摘要
Adversarial training is widely acknowledged as the most effective defense against adversarial attacks. However, it is also well established that achieving both robustness and generalization in adversarially trained models involves a trade-off. The goal of this work is to provide an in depth comparison of different approaches for adversarial training in language models. Specifically, we study the effect of pre-training data augmentation as well as training time input perturbations vs. embedding space perturbations on the robustness and generalization of BERT-like language models. Our findings suggest that better robustness can be achieved by pre-training data augmentation or by training with input space perturbation. However, training with embedding space perturbation significantly improves generalization. A linguistic correlation analysis of neurons of the learned models reveal that the improved generalization is due to `more specialized' neurons. To the best of our knowledge, this is the first work to carry out a deep qualitative analysis of different methods of generating adversarial examples in adversarial training of language models.
更多查看译文
关键词
adversarial training,robustness,language models,generalizability
AI 理解论文
溯源树
样例
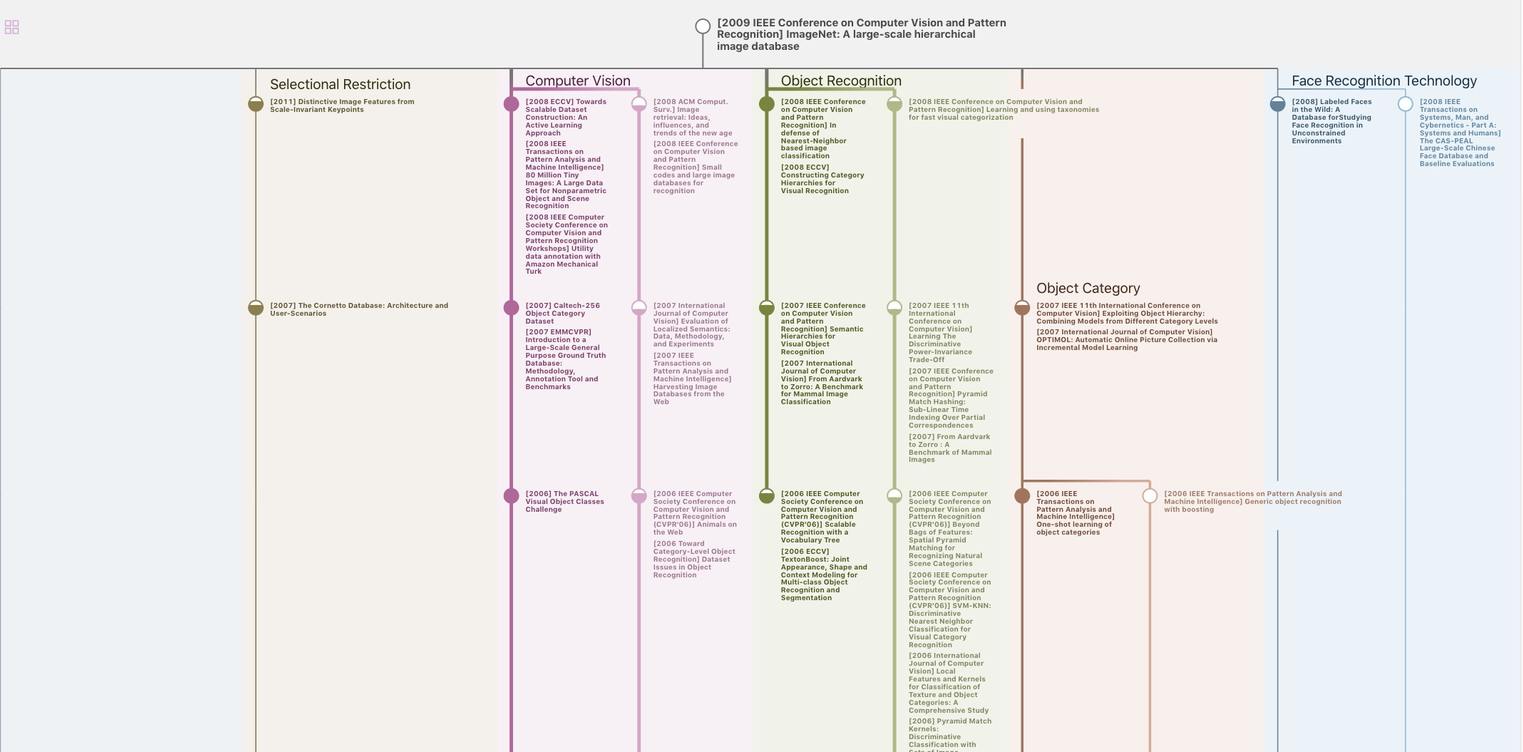
生成溯源树,研究论文发展脉络
Chat Paper
正在生成论文摘要