Density-Based Bonuses on Learned Representations for Reward-Free Exploration in Deep Reinforcement Learning
international conference on machine learning(2021)
Abstract
In this paper, we study the problem of representation learning and exploration in reinforcement learning. We propose a framework to compute exploration bonuses based on density estimation, that can be used with any representation learning method, and that allows the agent to explore without extrinsic rewards. In the special case of tabular Markov decision processes (MDPs), this approach mimics the behavior of theoretically sound algorithms. In continuous and partially observable MDPs, the same approach can be applied by learning a latent representation, on which a probability density is estimated.
MoreTranslated text
Key words
learned representations,reinforcement learning,exploration,density-based,reward-free
AI Read Science
Must-Reading Tree
Example
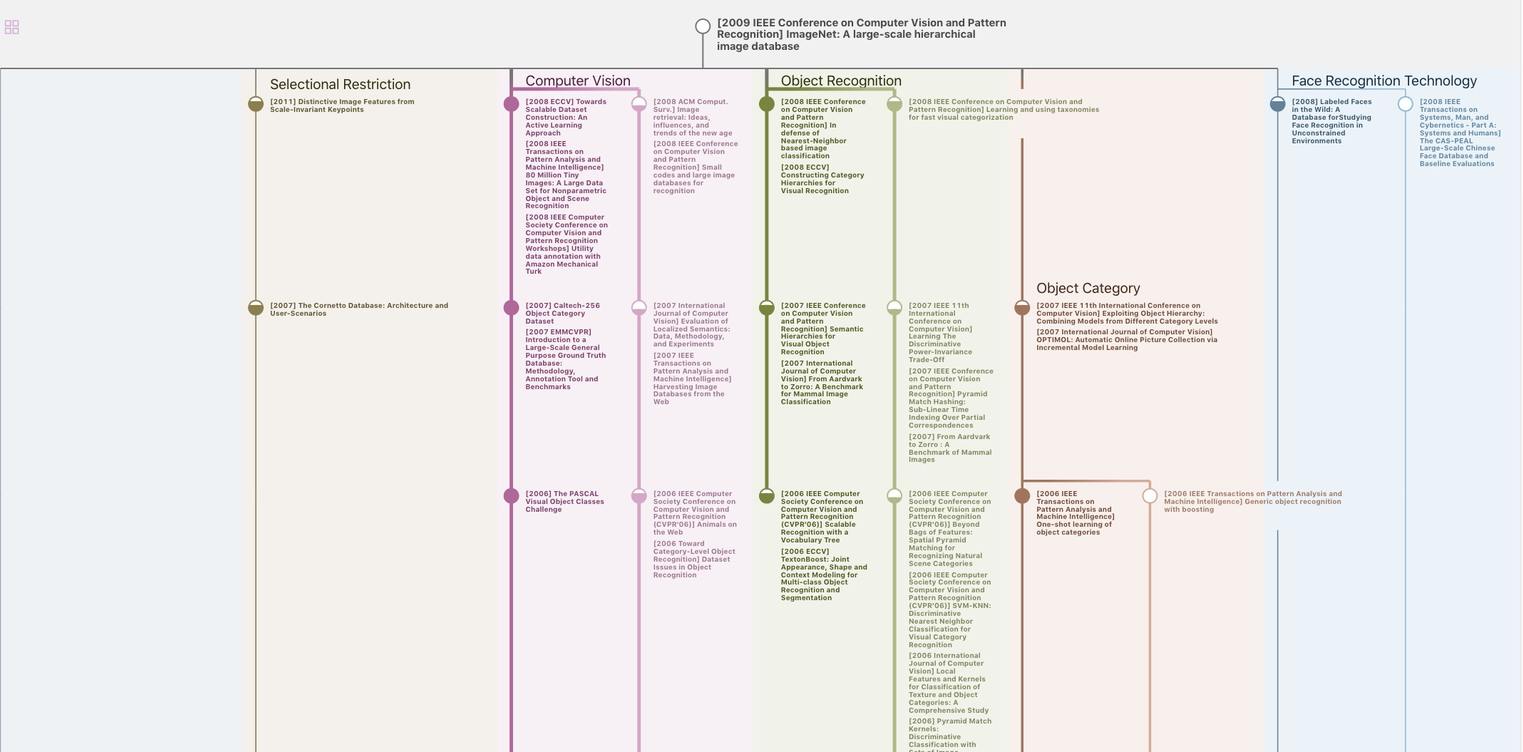
Generate MRT to find the research sequence of this paper
Chat Paper
Summary is being generated by the instructions you defined