Multimodal Mobility Framework: Towards Seamless Mobility Experience
2021 Joint Rail Conference(2021)
摘要
Mobility is no longer just a necessity for travelers, but choices among several possible routes and transportation modes. Urban passenger rail transport plays an essential role because it is affordable, convenient, safe, and fast. On the other hand, rail lines are limited to high passenger density corridors. Inevitably, rail has to be placed together with different transport modes, forming a multimodal network. However, to enable this integration with other modes of transport, numerous practical problems remain, such as making a smooth transition from the existing siloed, mode specific operational structure towards an interconnected system of transportation modes and business models for a seamless connected journey. The current isolated operational structure lacks a single truth and accurate visibility, which further discourages participation from augmenting transportation modes and leads to the extended reaction time for new technology integration. This research article introduces a Multimodal Mobility (MMM) solution framework that provides a functional interface to integrate and synchronize the railroad operations with other public transit networks (including train-bus-rapid transits) and micro-mobility services. The known approach to addressing the users' seamless mobility experience entails a centralized, prearranged, a priori knowledge and mechanism for operating intermodal transport systems. In contrast, the method defined in this paper focuses on a market-driven demand-responsive system that allows for dis-intermediation in a network of peer-level transportation modes operations. The framework facilitates blockchain-based decentralized and multi-organizational engagement. The focus here is the role of railroad in the multimodal ecosystem and its performance advancements in this integrated solutions framework. Leveraging a combination of graph analytics and machine learning algorithms, we provide methods to address challenges in encoding spatial and temporal dependencies of multimodal transit networks and handle complex optimization problems such as mixed time window and volume variation for resource allocation and transit operational analytics. This enables operation of different transit modes with varied resolution and flexibility for operational parameters like time, capacity, ridership, revenue management, etc. The analytics enable solutions for recommendations on synchronizing and integrating operations of transportation systems. Further, the network's decentralization and modular handling enable market-driven co-optimization of operational resources across various transportation modes to ensure seamless transit experience for users.
更多查看译文
关键词
Multimodal mobility,blockchain,graph neural network,machine learning,transit analytics,optimization,multiorganization engagement
AI 理解论文
溯源树
样例
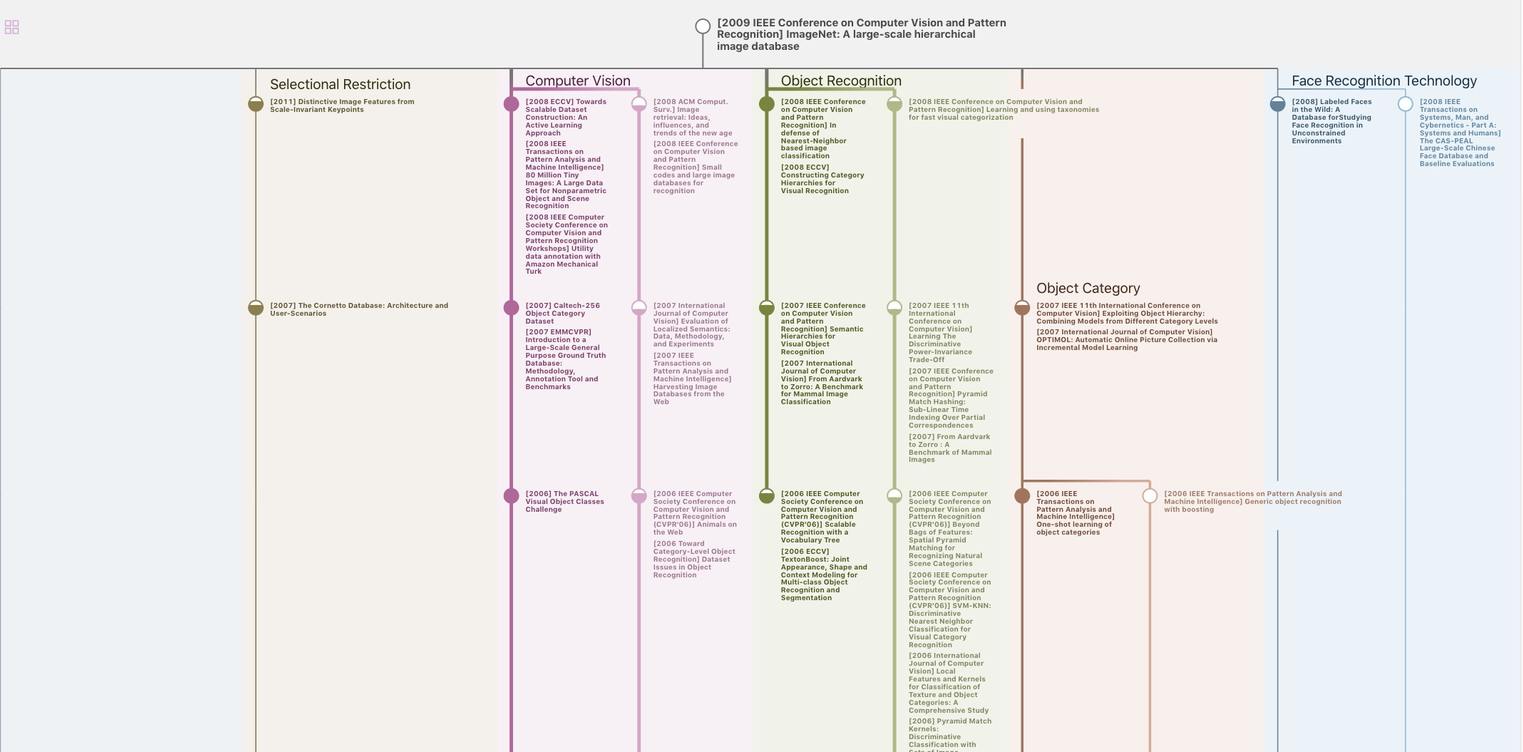
生成溯源树,研究论文发展脉络
Chat Paper
正在生成论文摘要