Diagnostic Performance of Convolutional Neural Network-Based TW3 Bone Age Assessment System
Social Science Research Network(2019)
Abstract
Purpose: This study established and validated a novel optimized TW3 artificial intelligence (TW3-AI) bone age assessment system by using the convolutional neural network (CNN).
Materials and Methods: A data set of 9,059 clinical radiographs of the left hand was obtained from PACS system between Jan 2012 and Dec 2016. Among these, 8005/9059 (88%) samples were treated as the training set for model implementation, 804/9059 (9%) samples as the validation set for parameters optimization, and the remaining 250/9059 (3%) samples were used to verify the accuracy and reliability of the model compared to that of four experienced endocrinologists and two experienced radiologists. The overall variation of TW3-RUS and TW3-Carpal bone score as well as each bone (13 RUS+7 Carpal) between reviewers and AI were compared by BA chart and Kappa test, respectively. Furthermore, the time consumption between the model and reviewers were also compared.
Results: The performance of TW3-AI model was highly consistent with the reviewers' overall estimation, and the RMS was 0.50 years. The accuracy of bone age assessment of TW3-AI model was generally better than the estimation of reviewer 5. Further analysis revealed that human interpretations of male capitate, hamate, the first distal and fifth middle phalanx and female capitate, trapezoid, the third and fifth middle phalanx were most inconsistent. The average image processing time was 1.5±0.2s in TW3-AI model, which was significantly shorter than manual interpretation.
Conclusion: The diagnostic performance of CNN-based TW3 bone age assessment was accurate and timesaving, and has a better stability compared to diagnostics made by experienced experts.
Funding Statement: This study was supported by the National Key Research and Development Program of China (No. 2016YFC1305301), National Natural Science Foundation of China (Nos. 81570759 and 81270938), Key Disciplines of Medicine (Innovation discipline, 11-CX24), Fundamental Research Funds for the Central Universities (2017XZZX001-01), Research Fund of Zhejiang Major Medical and Health Science and Technology & National Ministry of Health (WKJ-ZJ-1804), and Public Welfare Technology Application Research Program of Zhejiang Provincial Science and Technology Project (2016C33130),Zhejiang Province Natural Sciences Foundation -Zhejiang Society for Mathematical Medicine(LSZ19H070001).
Declaration of Interests: Xuelian Zhou, Ergang Wang, Qiang Lin, Guanping Dong, Wei Wu, Ke Huang, Gang Yu, Can Lai, Haichun Zhou, Xiaohui Ma, Xun Jia, Yongsheng Zheng, Lanxuan Liu, Da Ha, Hao Ni, Jun Yang and Junfen Fu declare there are no commercial relationships that might pose a conflict of interest in connection with the submitted manuscript.
Ethics Approval Statement: The institutional review boards of our Hospital approved the study and patients were informed consent.
MoreTranslated text
Key words
Vertebrae Detection,Texture Analysis,Computer-Aided Diagnosis,Medical Image Analysis
AI Read Science
Must-Reading Tree
Example
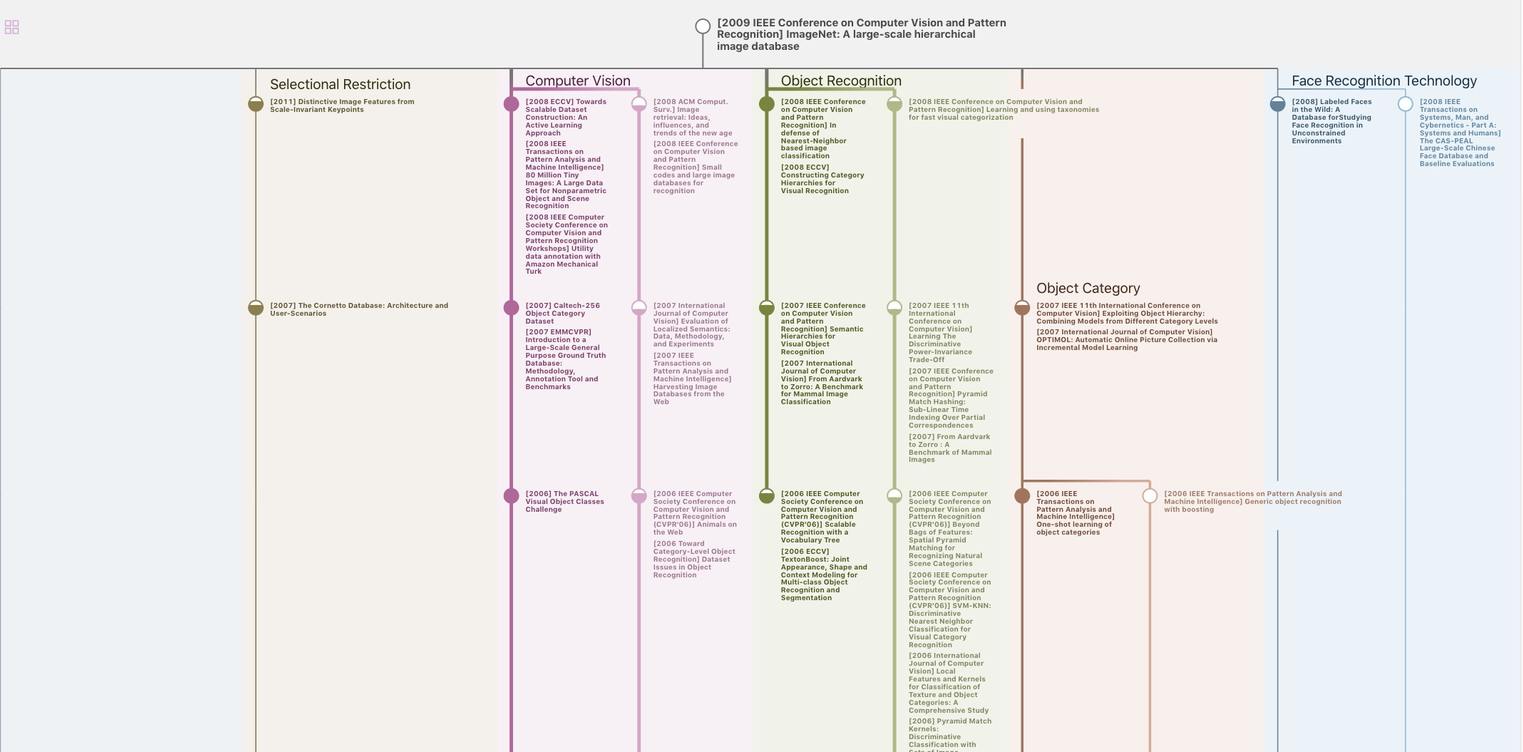
Generate MRT to find the research sequence of this paper
Chat Paper
Summary is being generated by the instructions you defined