Statistical Approach for Classifying Sentiment Reviews by Reducing Dimension using Truncated Singular Value Decomposition
2019 1st International Conference on Advances in Science, Engineering and Robotics Technology (ICASERT)(2019)
Abstract
Human sentiments, the attitudes, thoughts, or the judgments backed by feelings are the most important factors for the field of science and business which can be expressed through the web. The rapid growth of unstructured sentiment data has created a very big challenge to provide a fast and cost-efficient model for sentiment analysis. The aim of this paper is to find positive and negative emotions from the public comments rapidly and effectively. In this paper, to vectorize the text reviews and to reduce the dimension of the vectorized data, Term Frequency-Inverse Document Frequency (TF-IDF) and Truncated Singular Value Decomposition (TSVD) is used respectively. An efficient approach has been provided using the logistic regression algorithm employed in a vector space model of affective knowledge which gives fast learning speed. In result, this proposed method has reduced the learning time by 42.63% with the reduction of dimension from 12209 to 100 and has reported almost the same accuracy which is 85.7% and 89.3% for the movie review and yelp restaurant review dataset respectively. In comparison to existing methods, this method has shown almost the same accuracy after dimension reduction. Our result showed that our proposed system is useful.
MoreTranslated text
Key words
Sentiment Analysis,Logistic Regression,Truncated Singular Value Decomposition,Vector Space Model
AI Read Science
Must-Reading Tree
Example
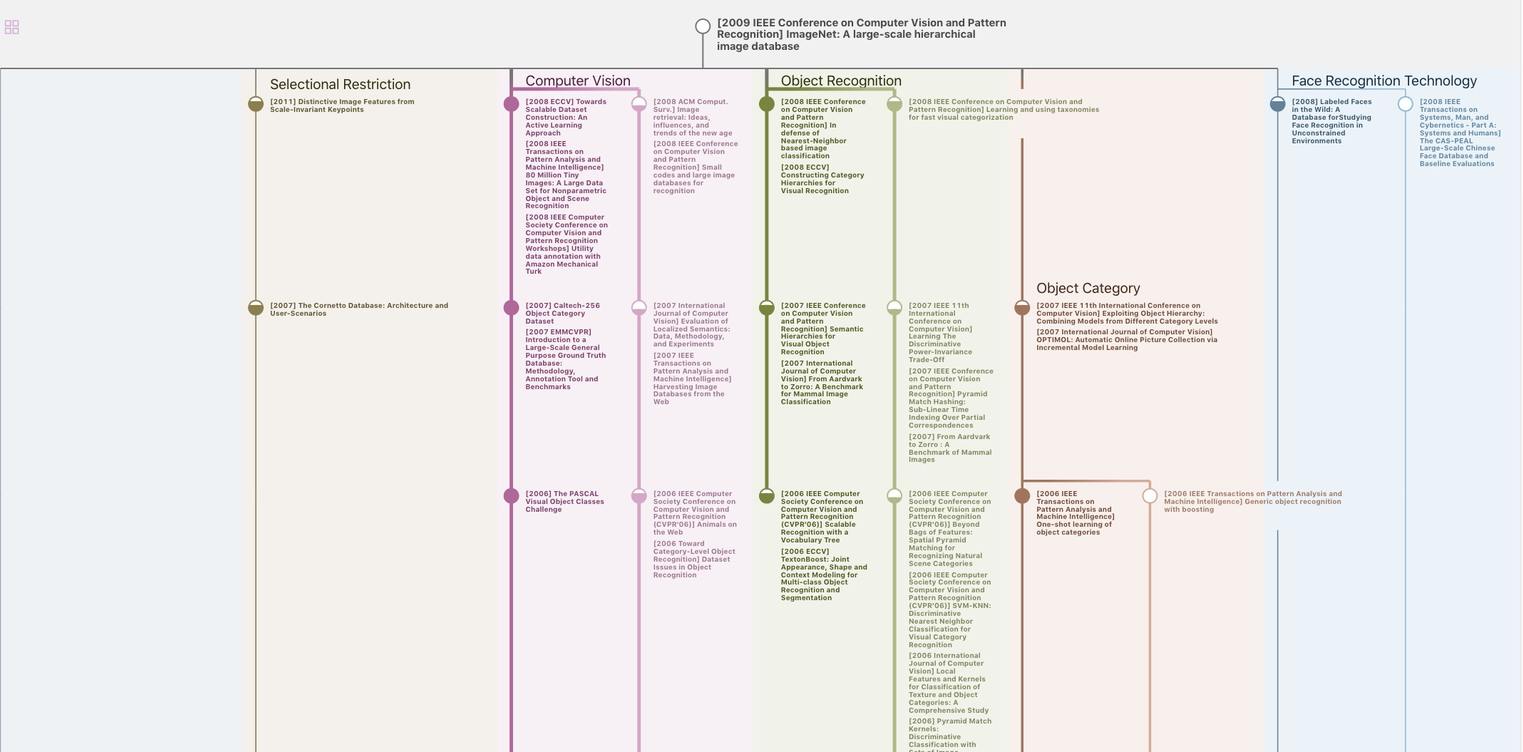
Generate MRT to find the research sequence of this paper
Chat Paper
Summary is being generated by the instructions you defined