Optional Verification and Signaling in Online Matching Markets: Evidence from a Randomized Field Experiment
Social Science Research Network(2020)
摘要
Online matching platforms that lack common informational mechanisms such as ratings and reviews often resort to mandatory verification of their users to enhance credibility. However, such mandatory verification may suppress the transmission of useful information for individual users. This study focuses on an alternate role of verification - its ability to serve as a credible signal for a user, when such verification is made optional and visible to other users. In collaboration with a leading online dating platform with no reputation mechanisms and where most of the information is self-disclosed, we design and conduct a randomized field experiment to examine not only who chooses to verify, but also the effectiveness of such optional verification for different types of users. Interestingly, we find users on the two sides use the same signal very differently. Males act consistent with the conventional prediction of signaling with High type males being more likely to opt-in to verification. As for females, we find Medium type females are the most likely to opt-in to verification as compared to High type females. We also find that such differential opt-in decisions are related to the differences in the credibility of the existing key attribute of each side, viz. income for males and beauty for females. In examining the outcomes of verification, we find that verified users receive more contacts from higher-type users, with the High type males and Medium type females benefiting the most. More interestingly, we find that verified users become more proactive and reach out to more and better potential partners. Further, the introduction of this voluntary verification facilitates desirable matching outcomes and benefits the platform as a whole. These findings have useful implications for research as well as practice.
更多查看译文
关键词
two-sided matching markets,signaling,optional verification,randomized field experiment,deep learning
AI 理解论文
溯源树
样例
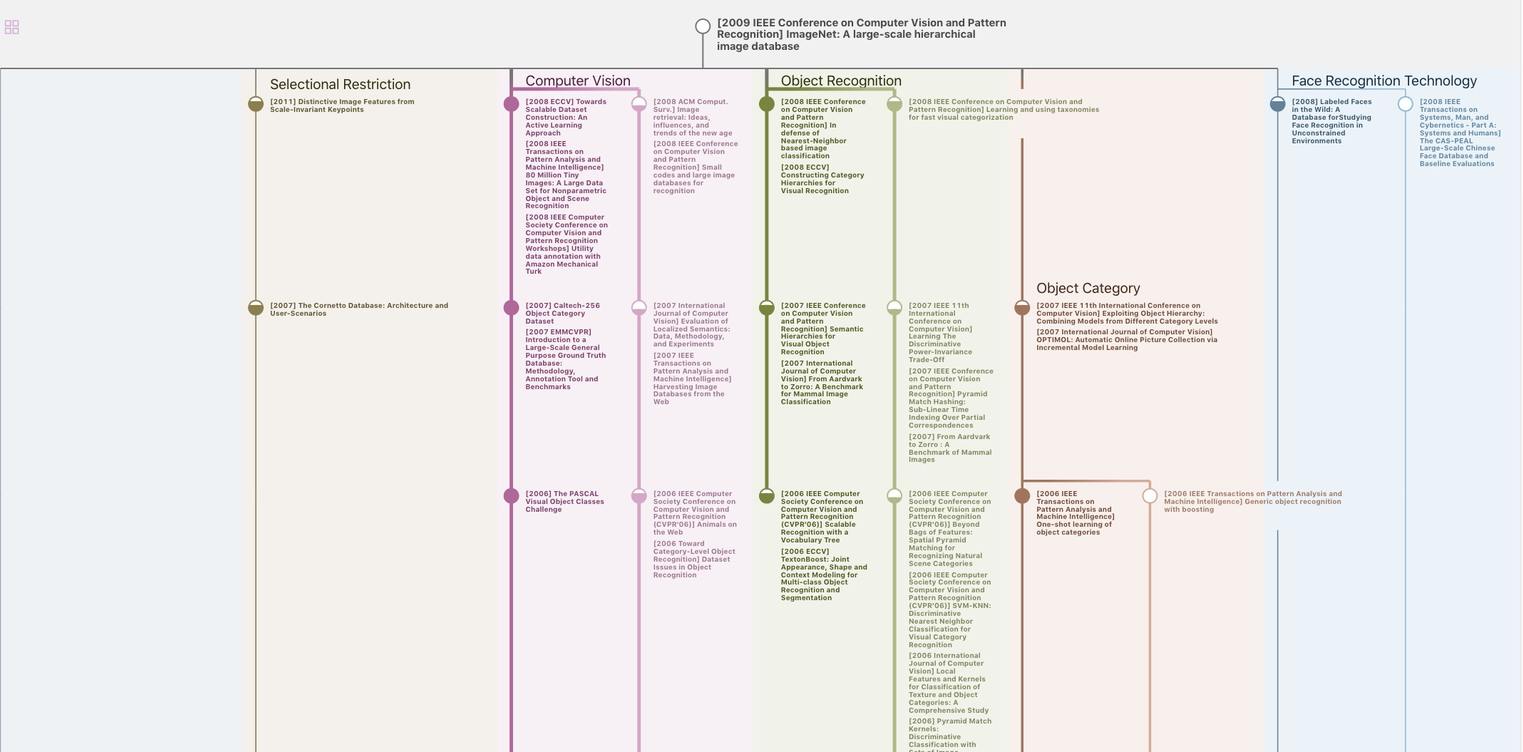
生成溯源树,研究论文发展脉络
Chat Paper
正在生成论文摘要