A CT-Derived Deep Neural Network Predicts for Programmed Death-1 Ligand-1 (PD-L1) Expression Status in Advanced Lung Adenocarcinomas (LUAD)
Social Science Research Network(2019)
摘要
Objectives: The current study aimed to non-invasively predict PD-L1 expression based on chest computed tomography (CT) images in advanced lung adenocarcinomas (LUAD), thus help to select optimal patients who can potentially benefit from immunotherapy. Methods: A total of 127 patients with stage III and IV unresectable LUAD were enrolled into this study. Pretreatment enhanced thin-section CT images were available for all patients and were analyzed in terms of both morphologic characteristics and deep learning (DL), so to further determine the association between CT features and PD-L1 expression status. Univariate Analysis and Multivariate Logical Regression Analysis were applied to evaluate significant variables. For DL, the 3D DenseNet Model was built and validated. Fivefold cross validation was practiced to evaluate the prediction efficacy of the model. The study cohort were grouped by PD-L1 TPS cutoff value of 1% (positive/negative expression) and 50% (weakly/strongly positive expression) respectively. Results: Among 127 LUAD patients, 46 (36.2%) patients were PD-L1-positive and 38 (29.9%) patients were strongly positive. For morphologic characteristics, univariate analysis showed that vascular involvement and lung metastasis were associated with PD-L1-negative/positive expression status (P =0.022 and P=0.002, respectively) and they were more commonly presented in PD-L1-negative subgroup. Whilst, only lung metastasis was associated with PD-L1-weakly/strongly positive expression status (P=0.010), and it was more commonly presented in PD-L1-weakly positive expression subgroup. Multivariate Logistic Regression Analysis revealed that only lung metastasis was significantly associated with PD-L1 expression status despite of different PD-L1 TPS cutoff values, and its AUC for predicting PD-L1 expression were less than 0.700. On the other hand, the predictive value of DL-3D DenseNet Model was higher than that of the morphologic characteristics, with AUC more than 0.750 in LUAD. Conclusions: CT features were significantly associated with PD-L1 expression status. PD-L1-negative and weakly positive LUAD showed lung metastasis more frequently than that of PD-L1-positive and strongly positive ones. Compared to morphologic CT features, DL-3D DenseNet Model improves the prediction efficacy, it has promising application value in predicting PD-L1 expression status in advanced LUAD.
Funding Statement: This work was supported by the National Natural Science Foundation of China (NO.81600065, NO.81771908 and NO.81571750). Declaration of Interests: The authors declare that they have no competing interests.
Ethics Approval Statement: This retrospective study was approved by the ethics committee of our institute and informed consents were waived.
更多查看译文
AI 理解论文
溯源树
样例
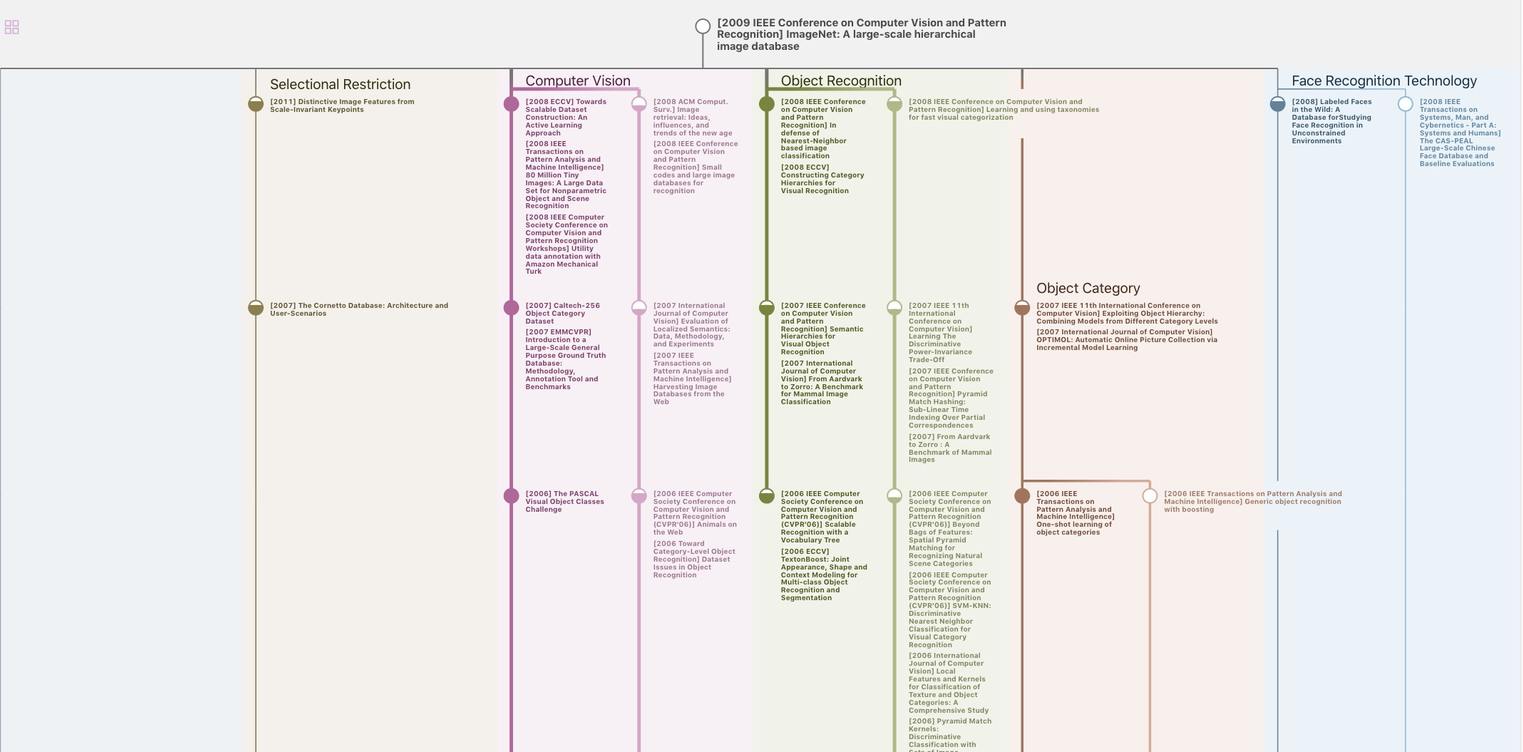
生成溯源树,研究论文发展脉络
Chat Paper
正在生成论文摘要