A Novel Graph Metalearning Method for SAR Target Recognition
IEEE GEOSCIENCE AND REMOTE SENSING LETTERS(2022)
摘要
Deep neural networks have been successfully applied to synthetic aperture radar automatic target recognition (SAR-ATR). The high performance of related methods relies on a large amount of training data. However, in practical applications, collecting SAR data is an expensive and time-consuming process, which results in few data available for training. Recognition with limited training data has become an essential issue in SAR-ATR. To solve this problem, we propose a novel graph metalearning method, in which the graph neural network is combined with metalearning to recognize SAR targets using few labeled SAR images. First, we use simulated SAR data to acquire metaknowledge in the form of feature extractor parameters. The feature extractor is then employed to encode the labeled and unlabeled real SAR images into embedding vectors. Second, the embedding vectors are constructed as a fully connected graph, where each node represents an image and each edge is the similarity between two nodes. After iteratively updating the graph by neighborhood aggregation, the new representations of the nodes and their relationship are obtained. Finally, we generate a prediction distribution on the target classes by fusing the node and edge information of the unlabeled image. The experimental results demonstrate the proposed method's superiority in recognition accuracy with few training data.
更多查看译文
关键词
Feature extraction,Radar polarimetry,Task analysis,Synthetic aperture radar,Image edge detection,Training data,Target recognition,Graph neural network,metalearning,synthetic aperture radar-automatic target recognition (SAR-ATR)
AI 理解论文
溯源树
样例
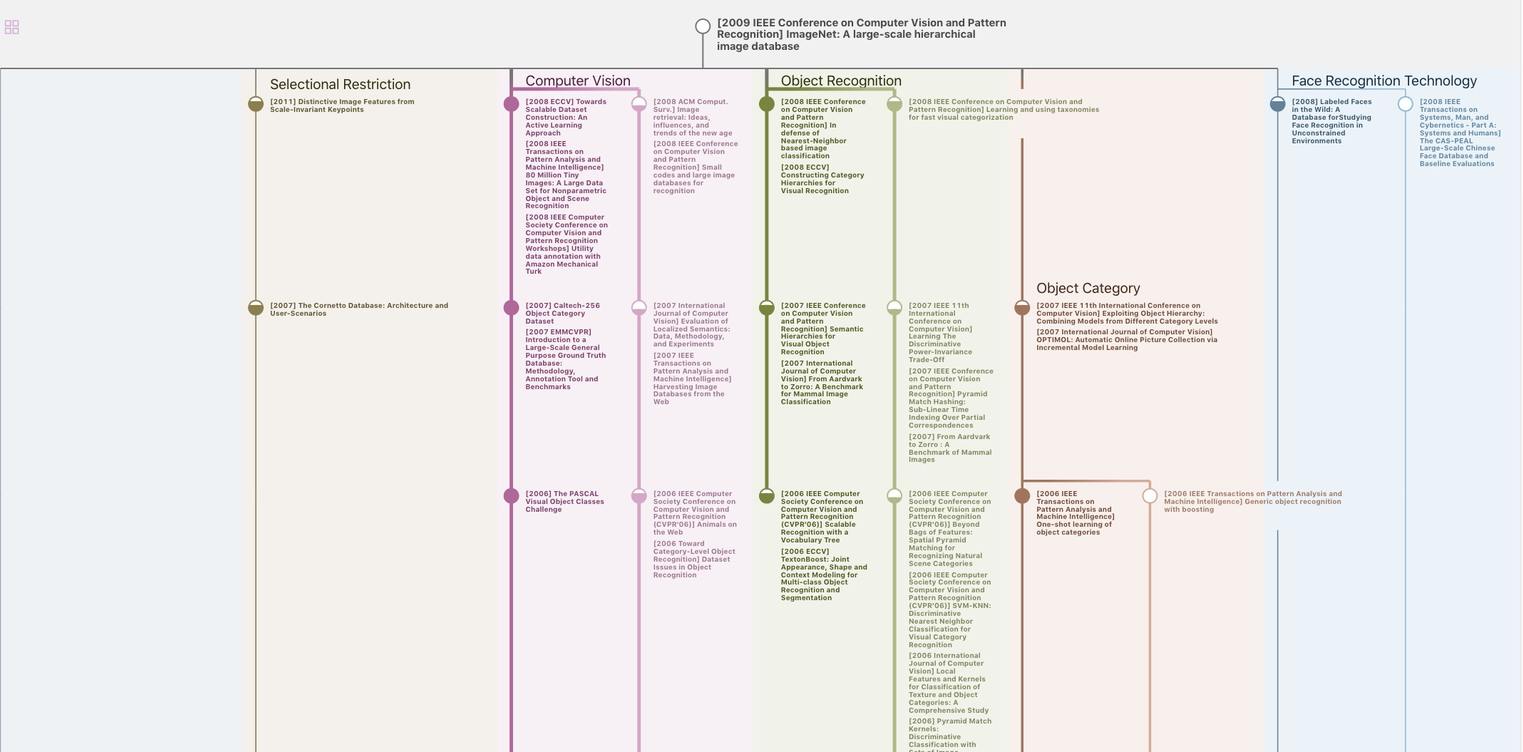
生成溯源树,研究论文发展脉络
Chat Paper
正在生成论文摘要