F04 Linear mixed model for the age of onset prediction in huntington disease from a peruvian cohort
Journal of Neurology, Neurosurgery, and Psychiatry(2021)
摘要
Background Based on heritability analyses, unidentified genetic modifiers explain up to 38% of the remaining genetic variance after accounting for the CAG repeat number. We hypothesized that pedigree information can harness unknown genetic modifiers to increase the accuracy of age of onset prediction Aims To assess whether pedigree information can increase the accuracy of age of onset predictive models. Methods We included 139 unrelated subjects and 81 related individuals from 33 families (total n=220) from the HD registry of the Neurogenetics Research Center at the Instituto Nacional de Ciencias Neurologicas, Lima, Peru. We fit a mixed linear model (MLM) of the age of onset with CAG repeat number, age, and sex as covariates. Polygenic additive effects were modeled by a matrix of kinship coefficients. Next, we measure the prediction accuracy by the Variance Explained based on Leve-one-out cross-validation (VEcv). The prediction accuracy was separately measured for test subjects with relatives in the training set and for subjects without relatives in the training set. The training sample size was kept constant for both groups. As a reference, we also fit a linear regression including only unrelated subjects (n=172), and repeated the MLM matching the sample size (81 subjects from 33 families + 91 unrelated subjects). Results The MLM (n=172) for subjects with relatives in the training set had a greater accuracy (VEcv=63%) than subjects without relatives (VEcv=56%). The linear regression of unrelated subjects (n=172) had a lower accuracy (VEcv=53%) than the MLM with matched sample size (n=172, VEcv=63%). Conclusion Including pedigree information in age of onset predictive models increases their accuracy.
更多查看译文
关键词
huntington disease,onset prediction,mixed model
AI 理解论文
溯源树
样例
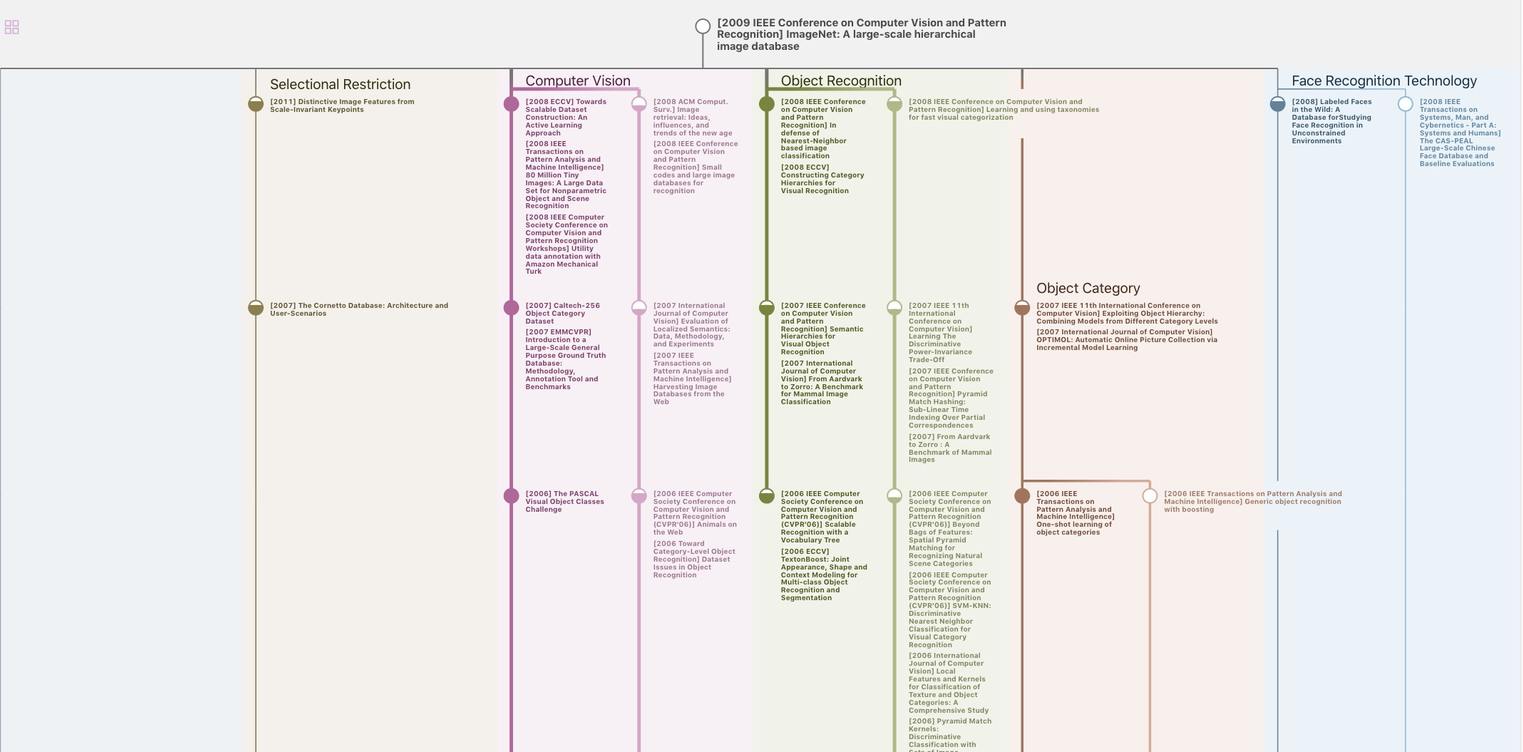
生成溯源树,研究论文发展脉络
Chat Paper
正在生成论文摘要