Matching 3D Facial Shape to Demographic Properties by Geometric Metric Learning: A Part-Based Approach
IEEE Transactions on Biometrics, Behavior, and Identity Science(2022)
摘要
Face recognition is a widely accepted biometric identifier, as the face contains a lot of information about the identity of a person. The goal of this study is to match the 3D face of an individual to a set of demographic properties (sex, age, BMI, and genomic background) that are extracted from unidentified genetic material. We introduce a triplet loss metric learner that compresses facial shape into a lower dimensional embedding while preserving information about the property of interest. The metric learner is trained for multiple facial segments to allow a global-to-local part-based analysis of the face. To learn directly from 3D mesh data, spiral convolutions are used along with a novel mesh-sampling scheme, which retains uniformly sampled points at different resolutions. The capacity of the model for establishing identity from facial shape against a list of probe demographics is evaluated by enrolling the embeddings for all properties into a support vector machine classifier or regressor and then combining them using a naive Bayes score fuser. Results obtained by a 10-fold cross-validation for biometric verification and identification show that part-based learning significantly improves the systems performance for both encoding with our geometric metric learner or with principal component analysis.
更多查看译文
关键词
Deep metric learning,face to DNA,geometric deep learning,multi biometrics,soft biometrics
AI 理解论文
溯源树
样例
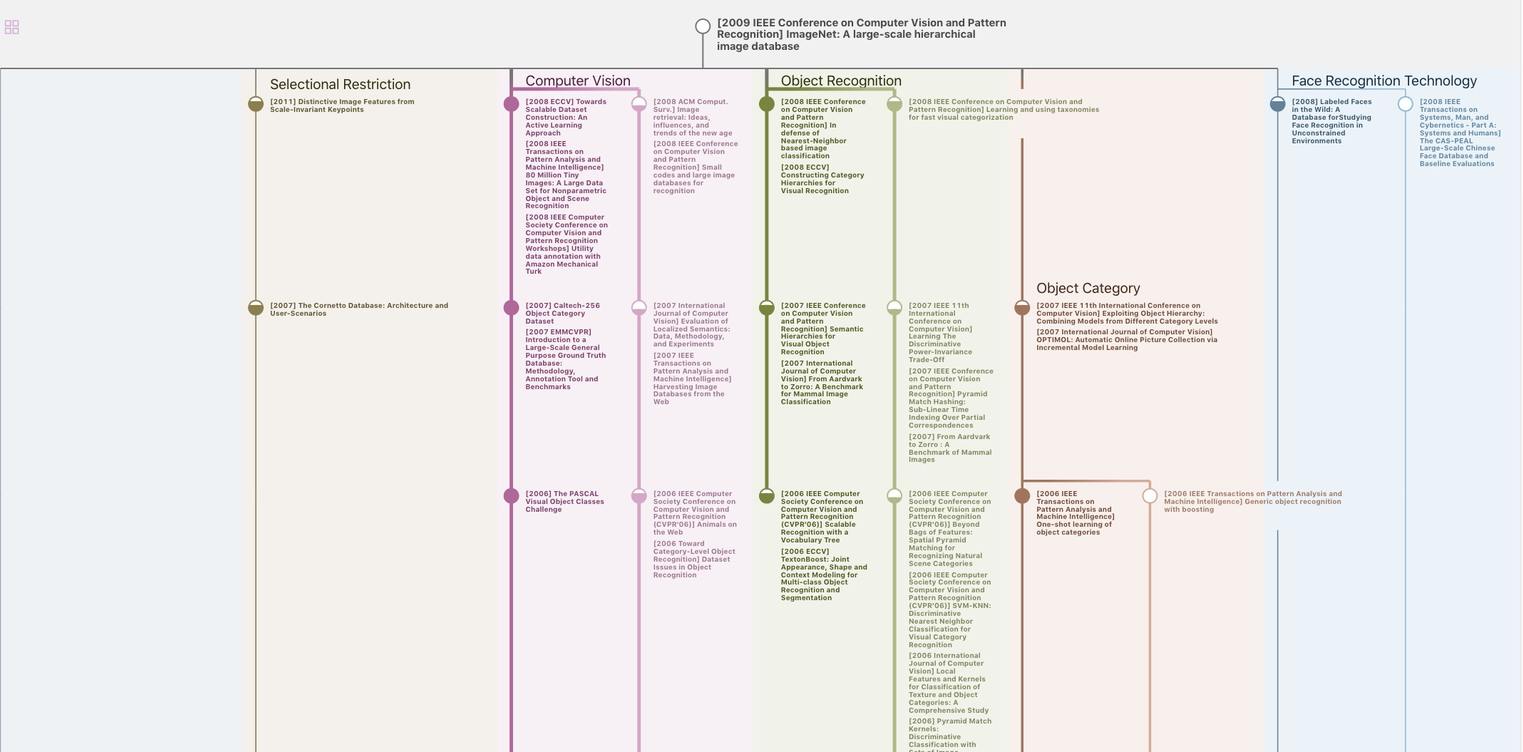
生成溯源树,研究论文发展脉络
Chat Paper
正在生成论文摘要