On a Dirichlet Process Mixture Representation of Phase-Type Distributions
BAYESIAN ANALYSIS(2022)
Abstract
An explicit representation of phase-type distributions as an infinite mixture of Erlang distributions is introduced. The representation unveils a novel and useful connection between a class of Bayesian nonparametric mixture mod-els and phase-type distributions. In particular, this sheds some light on two hot topics, estimation techniques for phase-type distributions, and the availability of closed-form expressions for some functionals related to Dirichlet process mixture models. The power of this connection is illustrated via a posterior inference al-gorithm to estimate phase-type distributions, avoiding some difficulties with the simulation of latent Markov jump processes, commonly encountered in phase-type Bayesian inference. On the other hand, closed-form expressions for functionals of Dirichlet process mixture models are illustrated with density and renewal function estimation, related to the optimal salmon weight distribution of an aquaculture study.
MoreTranslated text
Key words
Bayesian nonparametrics,Erlang distribution,mixture model,renewal function
AI Read Science
Must-Reading Tree
Example
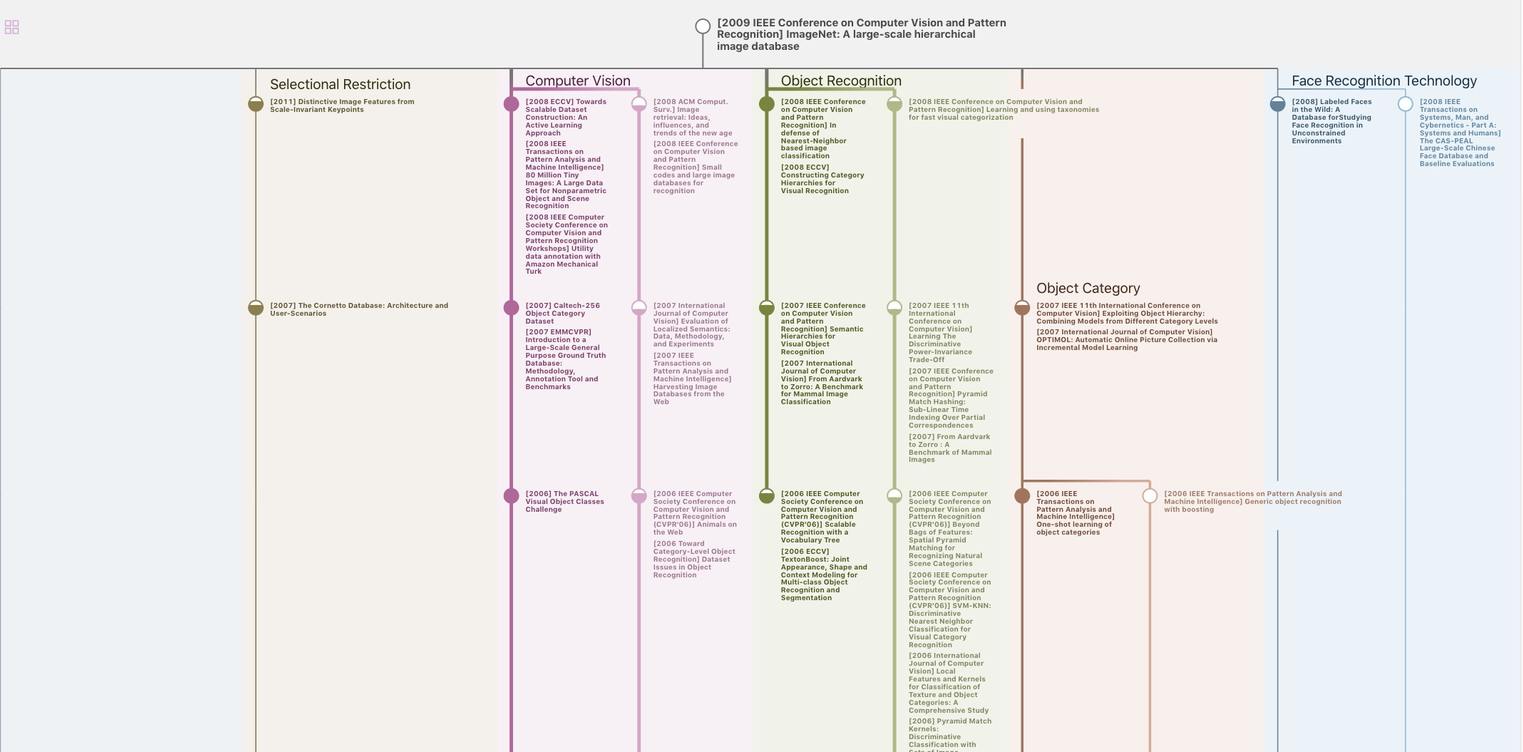
Generate MRT to find the research sequence of this paper
Chat Paper
Summary is being generated by the instructions you defined