A Multi-branch Decoder Network Approach toAdaptive Temporal Data Selection andReconstruction for Big Scientific Simulation Data
IEEE Transactions on Big Data(2021)
摘要
A key challenge in scientific simulation is that the simulation outputs often require intensive I/O and storage space to store the results for effective post hoc analysis. This article focuses on a
quality-aware adaptive temporal data selection and reconstruction
problem where the goal is to adaptively select simulation data samples at certain key timesteps in situ and reconstruct the discarded samples with quality assurance during post hoc analysis. This problem is motivated by the limitation of current solutions that a significant amount of simulation data samples are either discarded or aggregated during the sampling process, leading to inaccurate modeling of the simulated phenomena. Two unique challenges exist: 1) the sampling decisions have to be made in situ and adapted to the dynamics of the complex scientific simulation data; 2) the reconstruction error must be strictly bounded to meet the application requirement. To address the above challenges, we develop
DeepSample
, an error-controlled convolutional neural network framework, that jointly integrates a set of coherent multi-branch deep decoders to effectively reconstruct the simulation data with rigorous quality assurance. The results on two real-world scientific simulation applications show that DeepSample significantly outperforms other state-of-the-art methods on both sampling efficiency and reconstructed simulation data quality.
更多查看译文
关键词
Big scientific simulation data,adaptive temporal data selection and reconstruction,multi-branch decoder network
AI 理解论文
溯源树
样例
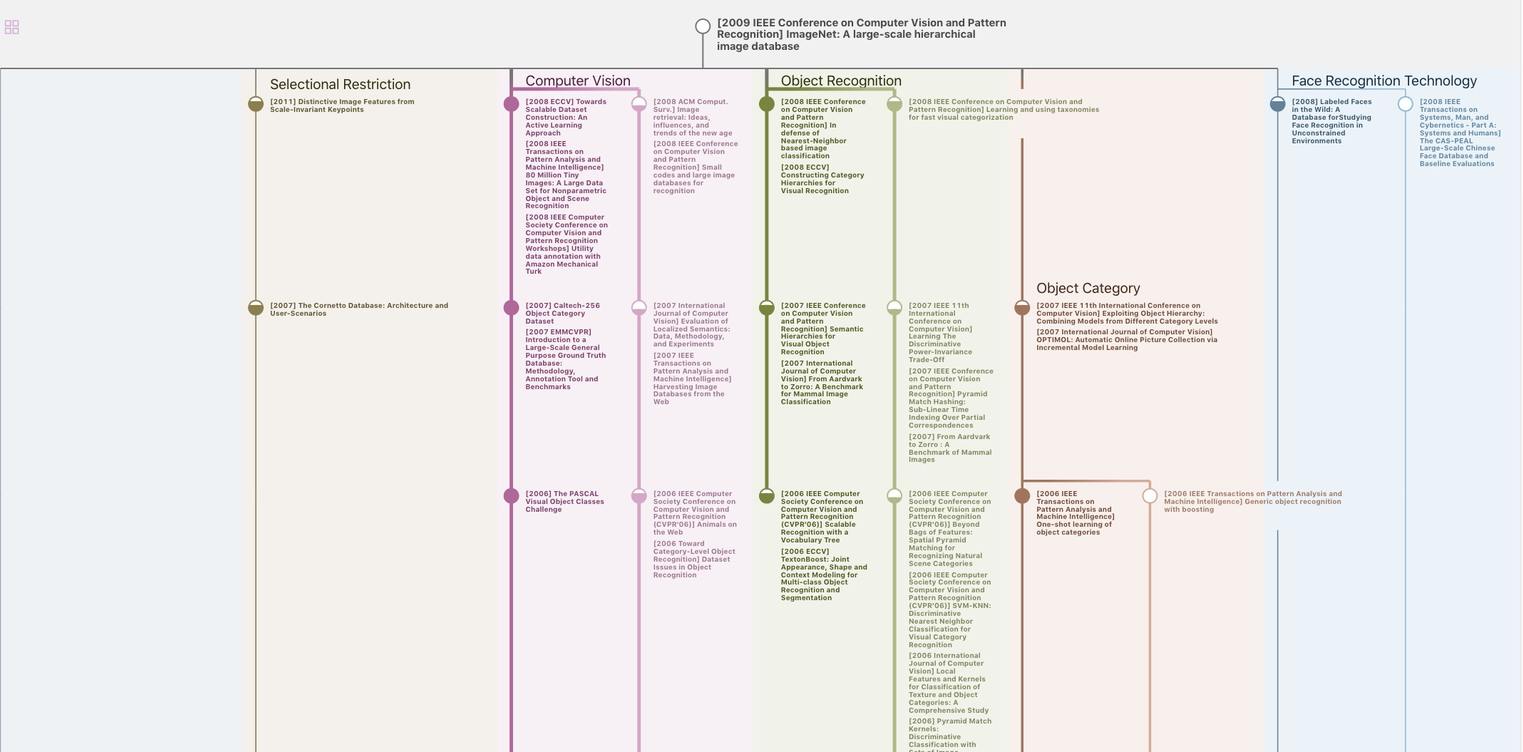
生成溯源树,研究论文发展脉络
Chat Paper
正在生成论文摘要