Machine learning of Ising criticality with spin-shuffling
arxiv(2023)
摘要
We investigate the performance of neural networks in identifying critical behaviour in the 2D Ising model with next-to-nearest neighbour interactions. We train DNN and CNN based classifiers on the Ising model configurations with nearest neighbour interactions and test the ability of our models to identify the critical (cross-over) region, as well as the phases in the presence of next-to-nearest neighbour interactions. Our main objective is to investigate whether our models can learn the notion of criticality and universality, in contrast to merely identifying the phases by simply learning the value of the order parameter, magnetization in this case. We design a simple adversarial training process which forces our models to ignore learning about the order parameter while training. We find that such models are able to identify critical region with reasonable success, implying that it has learned to identify the structure of spin-spin correlations near criticality.
更多查看译文
AI 理解论文
溯源树
样例
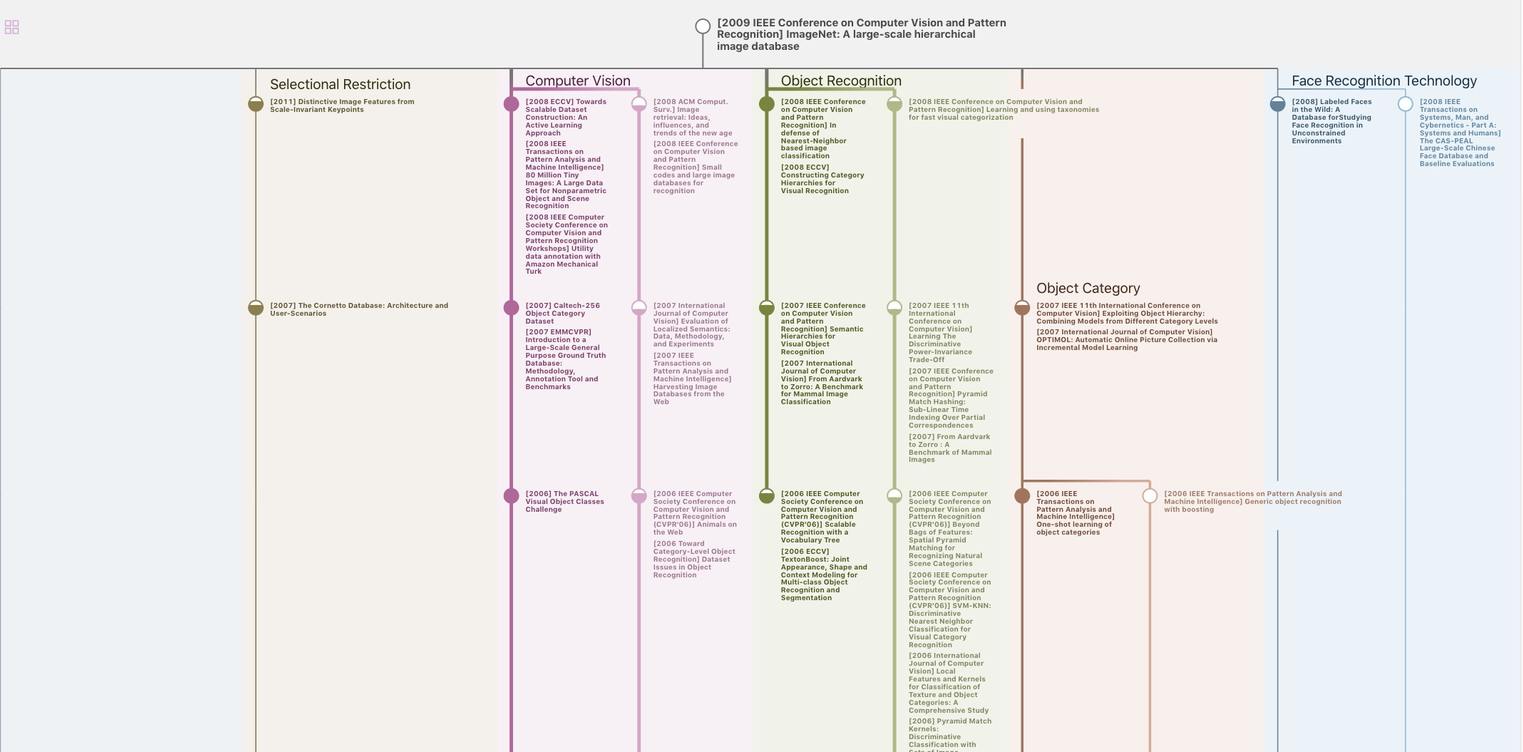
生成溯源树,研究论文发展脉络
Chat Paper
正在生成论文摘要