Efficient Underground Formation Characterization Using Transitional Markov Chain Monte Carlo Sampling
2020 IEEE USNC-CNC-URSI North American Radio Science Meeting (Joint with AP-S Symposium)(2020)
摘要
The underground formation characterization, which requires the inversion of the subsurface sensing data such as well logging, is challenging because of the non-linearity of the forward model and the high dimensionality of the unknown model parameters. Though the Markov chain Monte Carlo (MCMC) methods have been extensively used in geophysical inversion, the sampling time can be prohibitively long due to the low efficiency and the sequential property of the methods. The transitional Markov chain Monte Carlo (TMCMC), as an efficient sampling algorithm based-on MCMC, can improve the performance of MCMC and greatly reduce the sampling time. However, this method has not drawn much attention in the geophysical inverse problems. In this paper, we use the TMCMC to infer the underground formation from the electromagnetic well logging data. Since that the TMCMC is naturally suitable for parallel computing, the inversion process takes a much shorter time to achieve a similar result as the traditional MCMC sampling.
更多查看译文
关键词
electromagnetic well logging data,geophysical inverse problems,efficient sampling algorithm,TMCMC,sampling time,geophysical inversion,Markov chain Monte Carlo methods,unknown model parameters,forward model,subsurface sensing data,underground formation characterization,traditional MCMC sampling,transitional Markov chain Monte Carlo
AI 理解论文
溯源树
样例
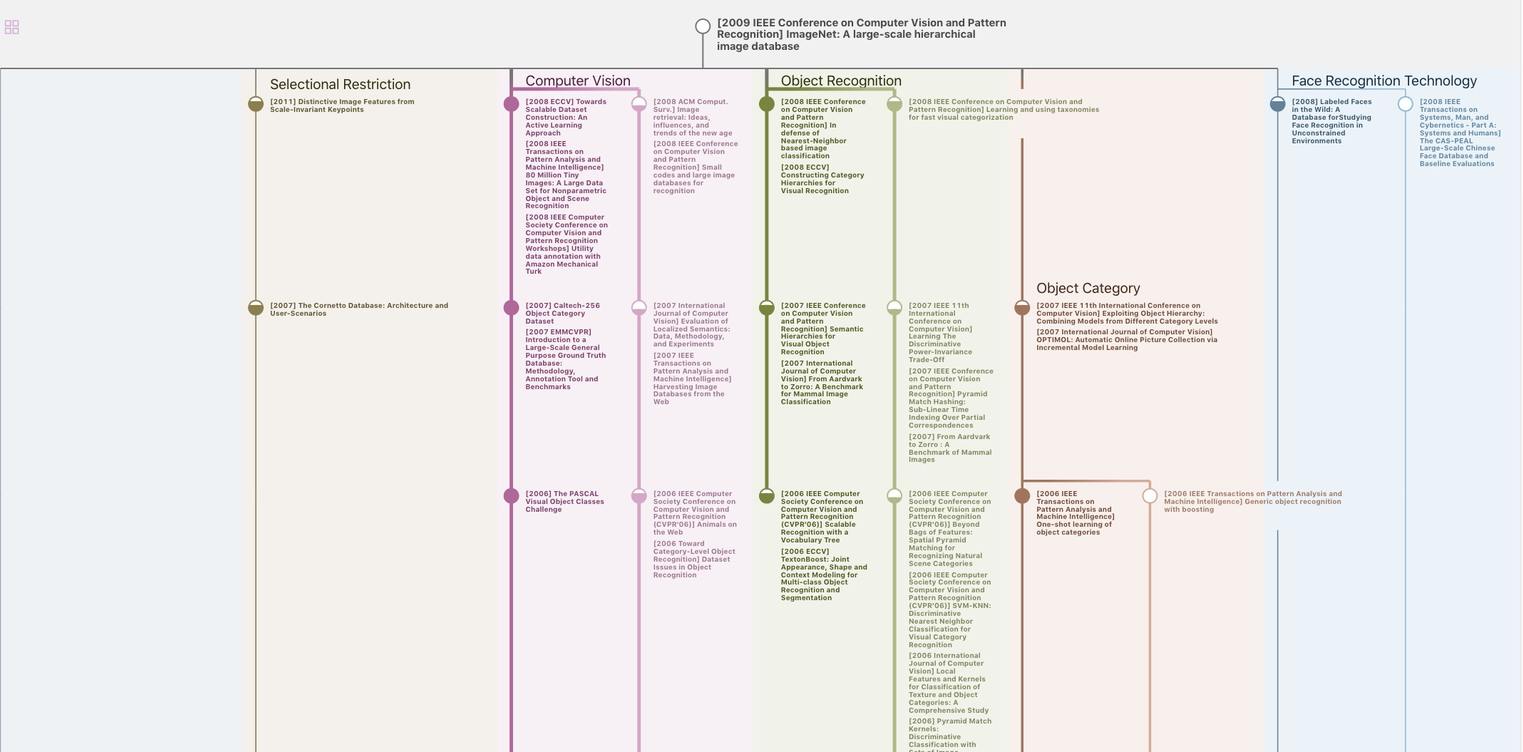
生成溯源树,研究论文发展脉络
Chat Paper
正在生成论文摘要