Detection of Tremor Associated with Rest and Effort Activity Using Machine Learning
2020 International Conference Automatics and Informatics (ICAI)(2020)
摘要
Tremor or handshaking can be provoked by neurological diseases like Parkinson's, physical efforts, stress, medicine, etc. To investigate the tremor characteristics, we asked volunteers to make three kinds of exercises: postural, rest, and effort. Accelerometer data were collected using sensors positioned on the wrist and finger of the participant's dominant hand. In the study, models for estimation and prediction of the tremor was generated. Machine learning was applied to detect and classify rest, effort, and postural tasks according to two scenarios. In the first scenario, we separated the tasks into three classes, while in the second one, two classes (effort and rest) were used. To compute the statistical features as maximum, minimum, and mean amplitude, number of peaks above the mean, standard deviation (STD), root mean square (RMS), and Pearson correlation of the finger and wrist acceleration, the accelerometer data were divided on windows with different length (128, 256, 320, and 512 samples per window). The following algorithms were used to build the models for the events' classification: decision tree, support vector machine, k-nearest neighbor, and ensemble bagging classifier. A cross-validation method was applied to train and test the models. The achieved performance of the models was from 85.0% to 94.1% for the 3-classes scenario and 86.5% to 94.9% for the two-classes scenario.
更多查看译文
关键词
activity recognition,decision tree,ensemble classifier,k-nearest neighbor,support vector machine,tremor
AI 理解论文
溯源树
样例
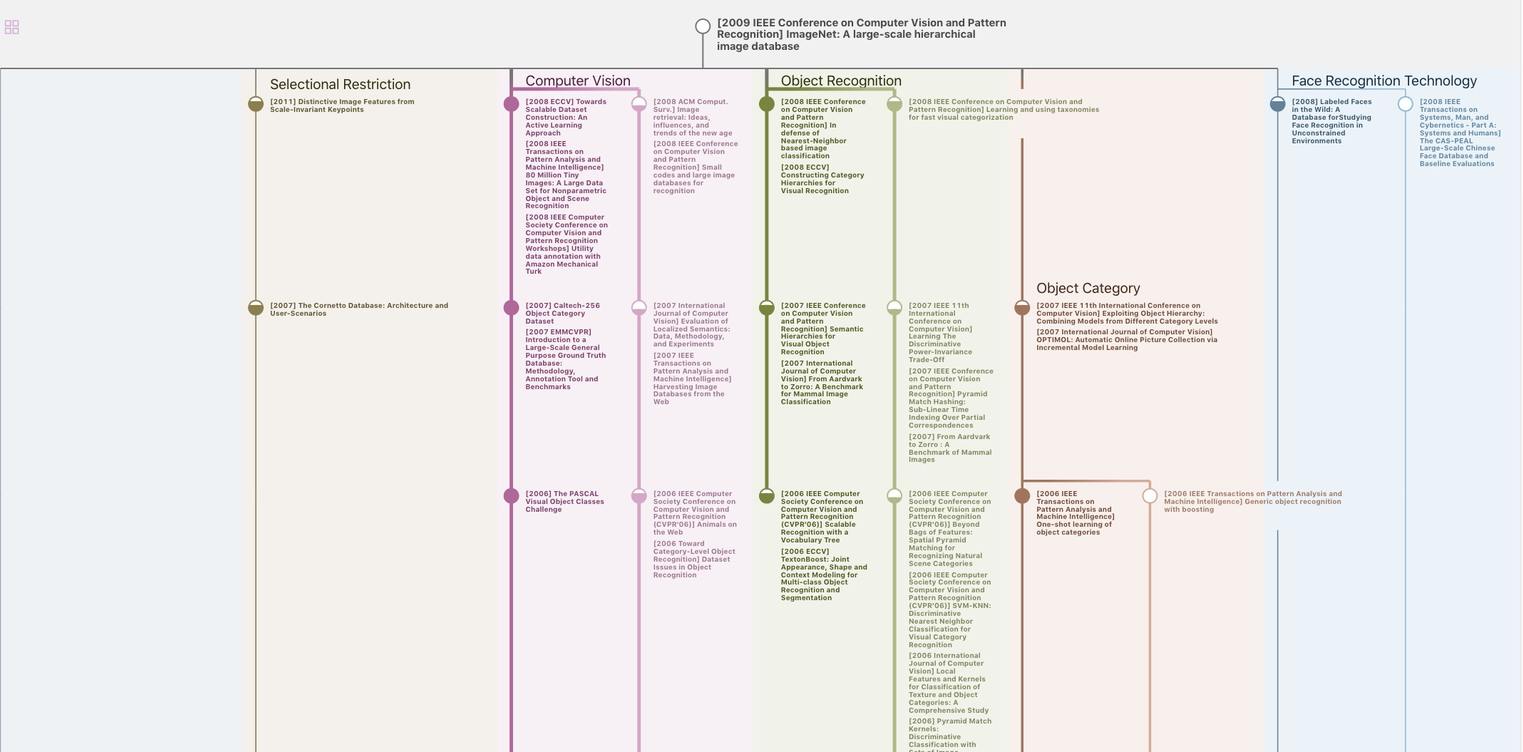
生成溯源树,研究论文发展脉络
Chat Paper
正在生成论文摘要