Improved image classification explainability with high-accuracy heatmaps
iScience(2022)
摘要
Deep learning models have become increasingly used for image-based classification. In critical applications such as medical imaging, it is important to convey the reasoning behind the models' decisions in human-understandable forms. In this work, we propose Pyramid Localization Network (PYLON), a deep learning model that delivers precise location explanation by increasing the resolution of heatmaps produced by class activation map (CAM). PYLON substantially improves the quality of CAM’s heatmaps in both general image and medical image domains and excels at pinpointing the locations of small objects. Most importantly, PYLON does not require expert annotation of the object location but instead can be trained using only image-level label. This capability is especially important for domain where expert annotation is often unavailable or costly to obtain. We also demonstrate an effective transfer learning approach for applying PYLON on small datasets and summarize technical guidelines that would facilitate wider adoption of the technique.
更多查看译文
关键词
Artificial intelligence,Computer science,Signal processing
AI 理解论文
溯源树
样例
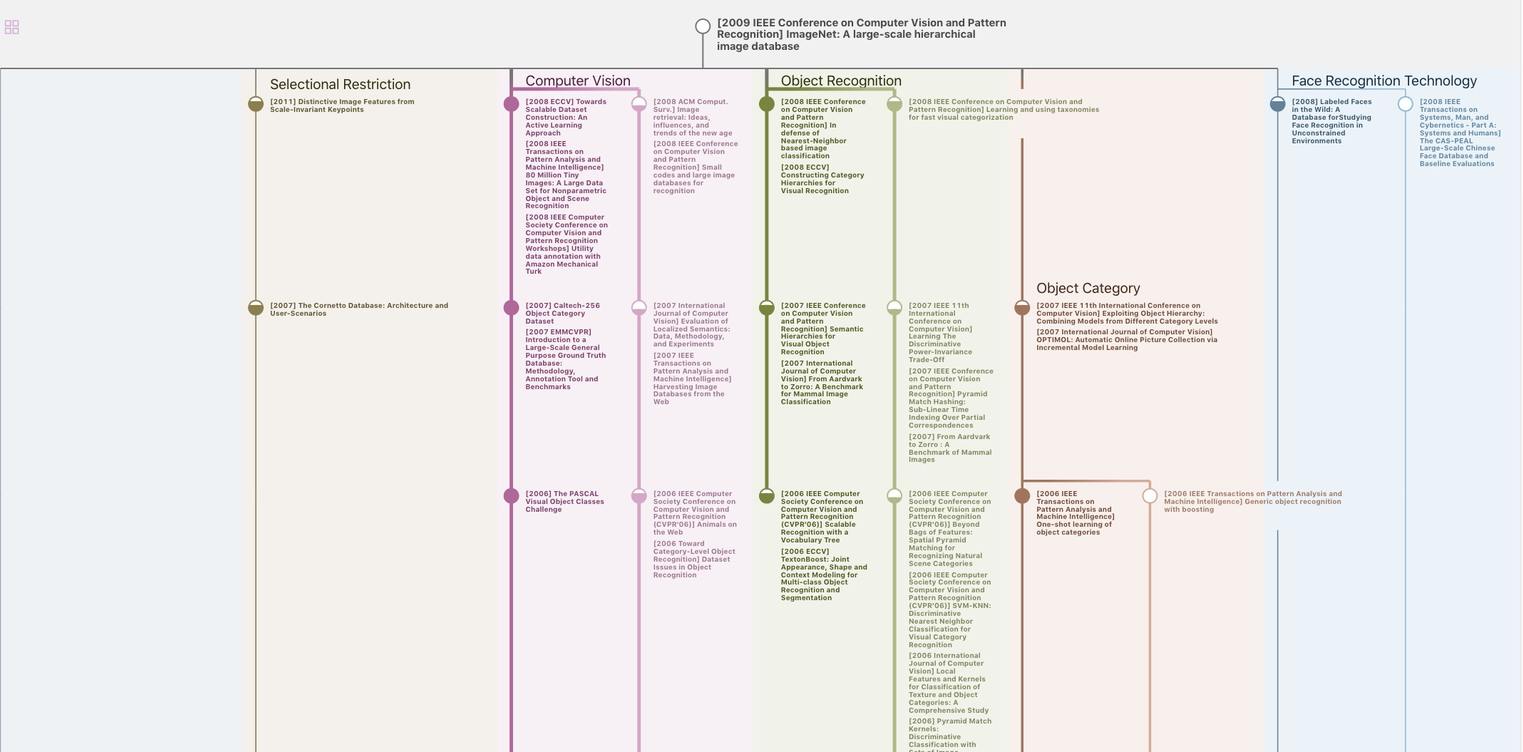
生成溯源树,研究论文发展脉络
Chat Paper
正在生成论文摘要