Precognition in Task-oriented Dialogue Understanding: Posterior Regularization by Future Context
CoRR(2022)
Abstract
Task-oriented dialogue systems have become overwhelmingly popular in recent researches. Dialogue understanding is widely used to comprehend users' intent, emotion and dialogue state in task-oriented dialogue systems. Most previous works on such discriminative tasks only models current query or historical conversations. Even if in some work the entire dialogue flow was modeled, it is not suitable for the real-world task-oriented conversations as the future contexts are not visible in such cases. In this paper, we propose to jointly model historical and future information through the posterior regularization method. More specifically, by modeling the current utterance and past contexts as prior, and the entire dialogue flow as posterior, we optimize the KL distance between these distributions to regularize our model during training. And only historical information is used for inference. Extensive experiments on two dialogue datasets validate the effectiveness of our proposed method, achieving superior results compared with all baseline models.
MoreTranslated text
Key words
dialogue understanding,posterior
AI Read Science
Must-Reading Tree
Example
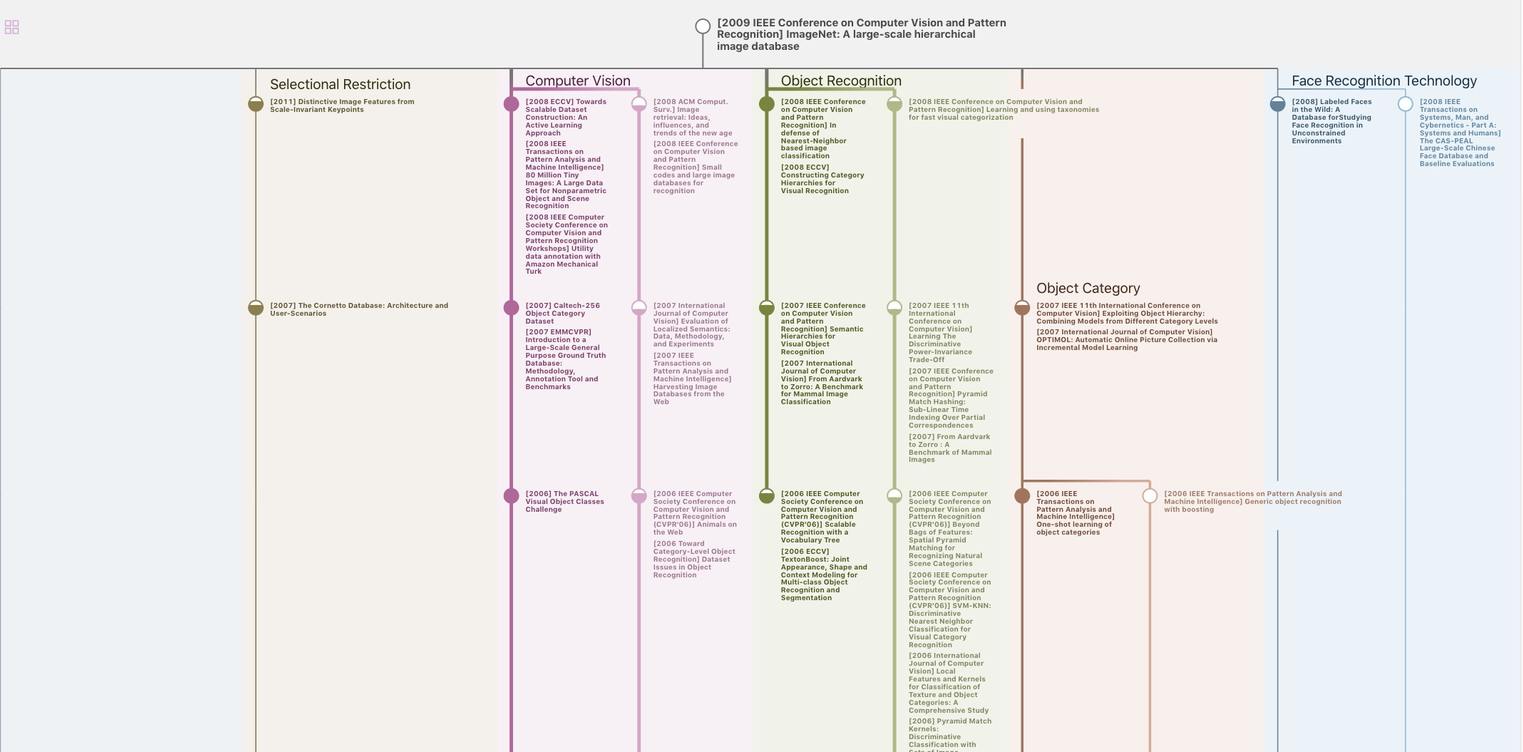
Generate MRT to find the research sequence of this paper
Chat Paper
Summary is being generated by the instructions you defined