Unbiased estimation using a class of diffusion processes
Journal of Computational Physics(2023)
摘要
We study the problem of unbiased estimation of expectations with respect to (w.r.t.) π a given, general probability measure on (Rd,B(Rd)) that is absolutely continuous with respect to a standard Gaussian measure. We focus on simulation associated to a particular class of diffusion processes, sometimes termed the Schrödinger-Föllmer Sampler, which is a simulation technique that approximates the law of a particular diffusion bridge process {Xt}t∈[0,1] on Rd, d∈N0. This latter process is constructed such that, starting at X0=0, one has X1∼π. Typically, the drift of the diffusion is intractable and, even if it were not, exact sampling of the associated diffusion is not possible. As a result, [10], [16] consider a stochastic Euler-Maruyama scheme that allows the development of biased estimators for expectations w.r.t. π. We show that for this methodology to achieve a mean square error of O(ϵ2), for arbitrary ϵ>0, the associated cost is O(ϵ−5). We then introduce an alternative approach that provides unbiased estimates of expectations w.r.t. π, that is, it does not suffer from the time discretization bias or the bias related with the approximation of the drift function. We prove that to achieve a mean square error of O(ϵ2), the associated cost (which is random) is, with high probability, O(ϵ−2|log(ϵ)|2+δ), for any δ>0. We implement our method on several examples including Bayesian inverse problems.
更多查看译文
关键词
Diffusions,Unbiased approximation,Schrödinger bridge,Markov chain simulation
AI 理解论文
溯源树
样例
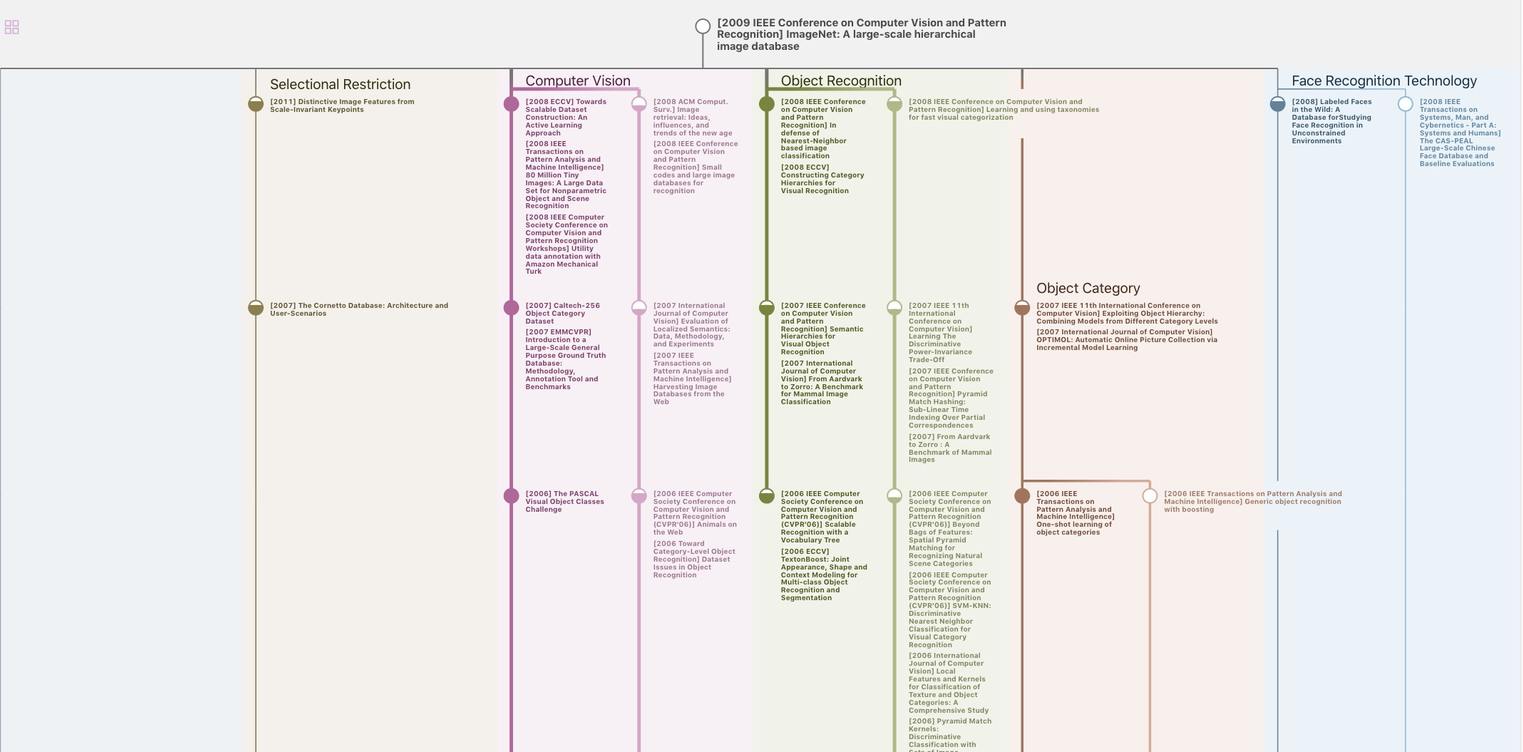
生成溯源树,研究论文发展脉络
Chat Paper
正在生成论文摘要