GeoDiff: A Geometric Diffusion Model for Molecular Conformation Generation
International Conference on Learning Representations (ICLR)(2022)
摘要
Predicting molecular conformations from molecular graphs is a fundamental problem in cheminformatics and drug discovery. Recently, significant progress has been achieved with machine learning approaches, especially with deep generative models. Inspired by the diffusion process in classical non-equilibrium thermodynamics where heated particles will diffuse from original states to a noise distribution, in this paper, we propose a novel generative model named GeoDiff for molecular conformation prediction. GeoDiff treats each atom as a particle and learns to directly reverse the diffusion process (i.e., transforming from a noise distribution to stable conformations) as a Markov chain. Modeling such a generation process is however very challenging as the likelihood of conformations should be roto-translational invariant. We theoretically show that Markov chains evolving with equivariant Markov kernels can induce an invariant distribution by design, and further propose building blocks for the Markov kernels to preserve the desirable equivariance property. The whole framework can be efficiently trained in an end-to-end fashion by optimizing a weighted variational lower bound to the (conditional) likelihood. Experiments on multiple benchmarks show that GeoDiff is superior or comparable to existing state-of-the-art approaches, especially on large molecules.
更多查看译文
关键词
molecular conformation generation,deep generative models,diffusion probabilistic models
AI 理解论文
溯源树
样例
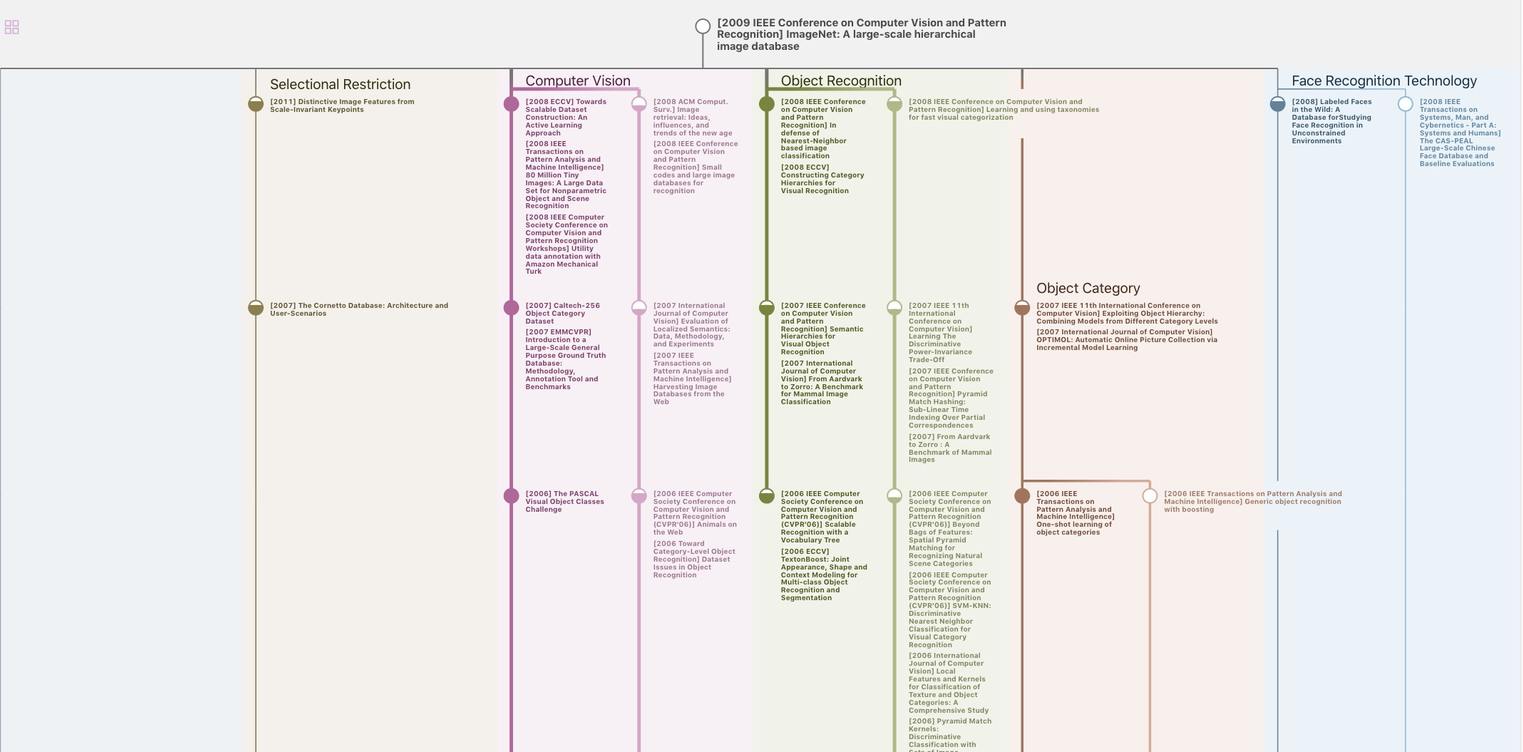
生成溯源树,研究论文发展脉络
Chat Paper
正在生成论文摘要