Contextformer: A Transformer with Spatio-Channel Attention for Context Modeling in Learned Image Compression.
European Conference on Computer Vision(2022)
摘要
Entropy modeling is a key component for high-performance image compression algorithms. Recent developments in autoregressive context modeling helped learning-based methods to surpass their classical counterparts. However, the performance of those models can be further improved due to the underexploited spatio-channel dependencies in latent space, and the suboptimal implementation of context adaptivity. Inspired by the adaptive characteristics of the transformers, we propose a transformer-based context model, named Contextformer, which generalizes the de facto standard attention mechanism to spatio-channel attention. We replace the context model of a modern compression framework with the Contextformer and test it on the widely used Kodak, CLIC2020, and Tecnick image datasets. Our experimental results show that the proposed model provides up to 11% rate savings compared to the standard Versatile Video Coding (VVC) Test Model (VTM) 16.2, and outperforms various learning-based models in terms of PSNR and MS-SSIM.
更多查看译文
关键词
Context model,Learned image compression,Transformers
AI 理解论文
溯源树
样例
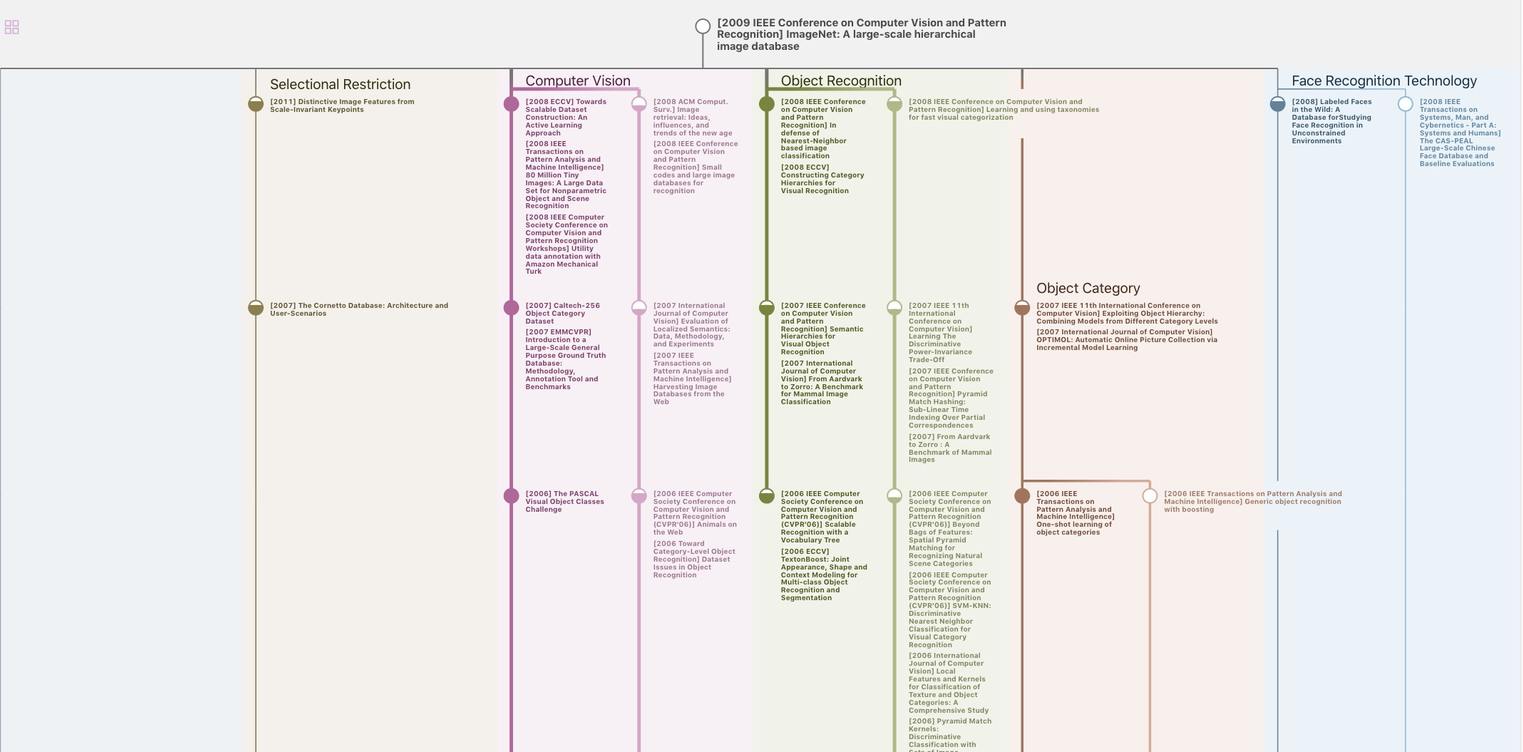
生成溯源树,研究论文发展脉络
Chat Paper
正在生成论文摘要