Adaptive Discounting of Implicit Language Models in RNN-Transducers
IEEE International Conference on Acoustics, Speech, and Signal Processing (ICASSP)(2022)
摘要
RNN-Transducer (RNN-T) models have become synonymous with streaming end-to-end ASR systems. While they perform competitively on a number of evaluation categories, rare words pose a serious challenge to RNN-T models. One main reason for the degradation in performance on rare words is that the language model (LM) internal to RNN-Ts can be-come overconfident and lead to hallucinated predictions that are acoustically inconsistent with the underlying speech. To address this issue, we propose a lightweight adaptive LM dis-counting technique ADAPTLMD, that can be used with any RNN-T architecture without requiring any external resources or additional parameters. ADAPTLMD uses a two-pronged approach: 1. Randomly mask the prediction network output to encourage the RNN-T to not be overly reliant on it’s outputs. 2. Dynamically choose when to discount the implicit LM (ILM) based on rarity of recently predicted tokens and divergence between ILM and implicit acoustic model (IAM) scores. Comparing ADAPTLMD to a competitive RNN-T baseline, we obtain up to 4% and 14% relative reductions in overall WER and rare word PER, respectively, on a conversational, code-mixed Hindi-English ASR task.
更多查看译文
关键词
RNN-Transducer,Implicit Language Model,Rare Word ASR
AI 理解论文
溯源树
样例
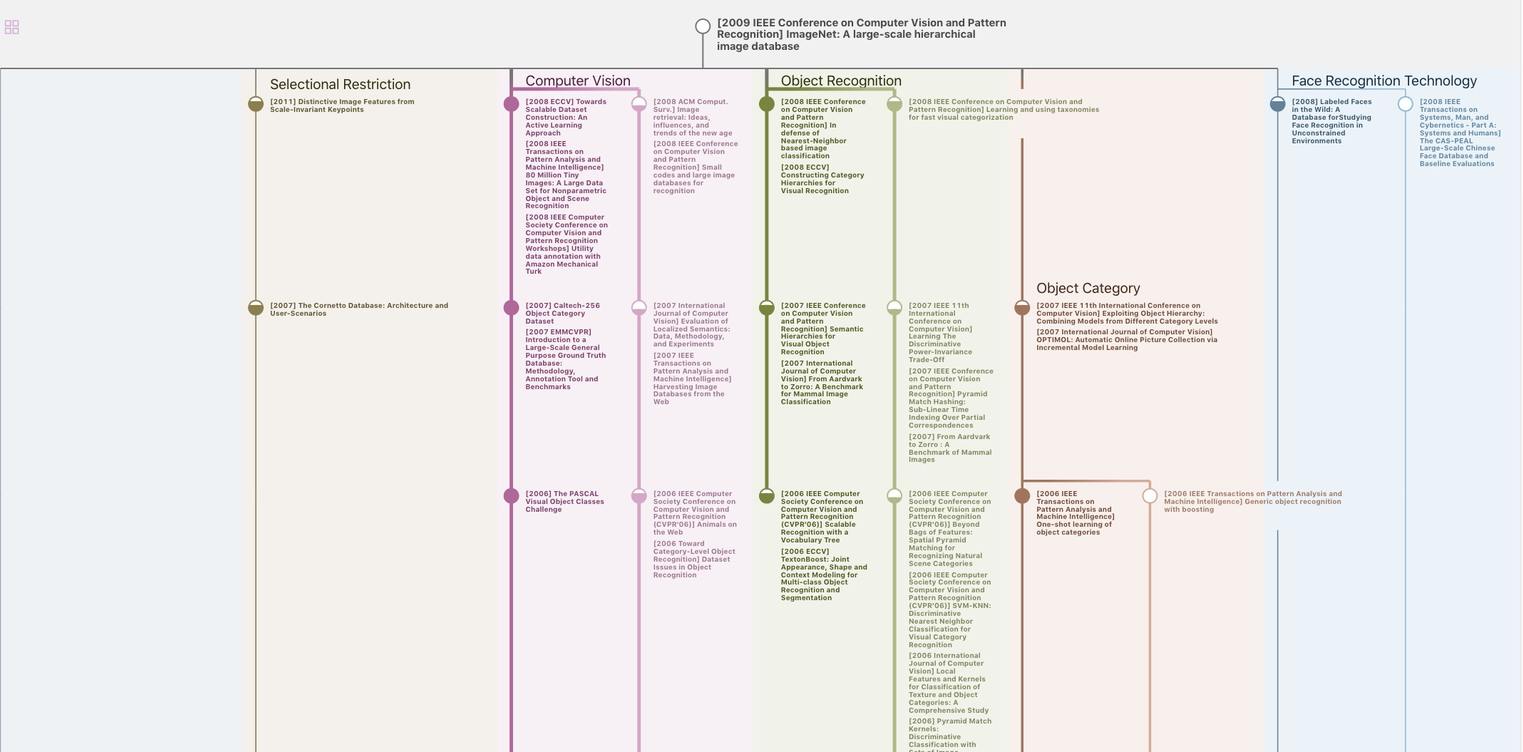
生成溯源树,研究论文发展脉络
Chat Paper
正在生成论文摘要