Triggered Gradient Tracking for asynchronous distributed optimization
Automatica(2023)
摘要
This paper proposes ASYNCHRONOUS TRIGGERED GRADIENT TRACKING, i.e., a distributed optimization algorithm to solve consensus optimization over networks with asynchronous communication. As a building block, we devise the continuous-time counterpart of the recently proposed (discrete-time) distributed gradient tracking called CONTINUOUS GRADIENT TRACKING. By using a Lyapunov approach, we prove exponential stability of the equilibrium corresponding to agents' estimates being consensual to the optimal solution, with arbitrary initialization of the local estimates. Then, we propose two triggered versions of the algorithm. In the first one, the agents continuously integrate their local dynamics and exchange with neighbors their current local variables in a synchronous way. In ASYNCHRONOUS TRIGGERED GRADIENT TRACKING, we propose a totally asynchronous scheme in which each agent sends to neighbors its current local variables based on a triggering condition that depends on a locally verifiable condition. The triggering protocol preserves the linear convergence of the algorithm and avoids the Zeno behavior, i.e., an infinite number of triggering events over a finite interval of time is excluded. By using the stability analysis of CONTINUOUS GRADIENT TRACKING as a preparatory result, we show exponential stability of the equilibrium point holds for both triggered algorithms and any estimate initialization. Finally, the simulations validate the effectiveness of the proposed methods on a data analytics problem, showing also improved performance in terms of inter-agent communication. (c) 2022 Elsevier Ltd. All rights reserved.
更多查看译文
关键词
Distributed optimization,Multi-agent systems,Large scale optimization problems and ,methods
AI 理解论文
溯源树
样例
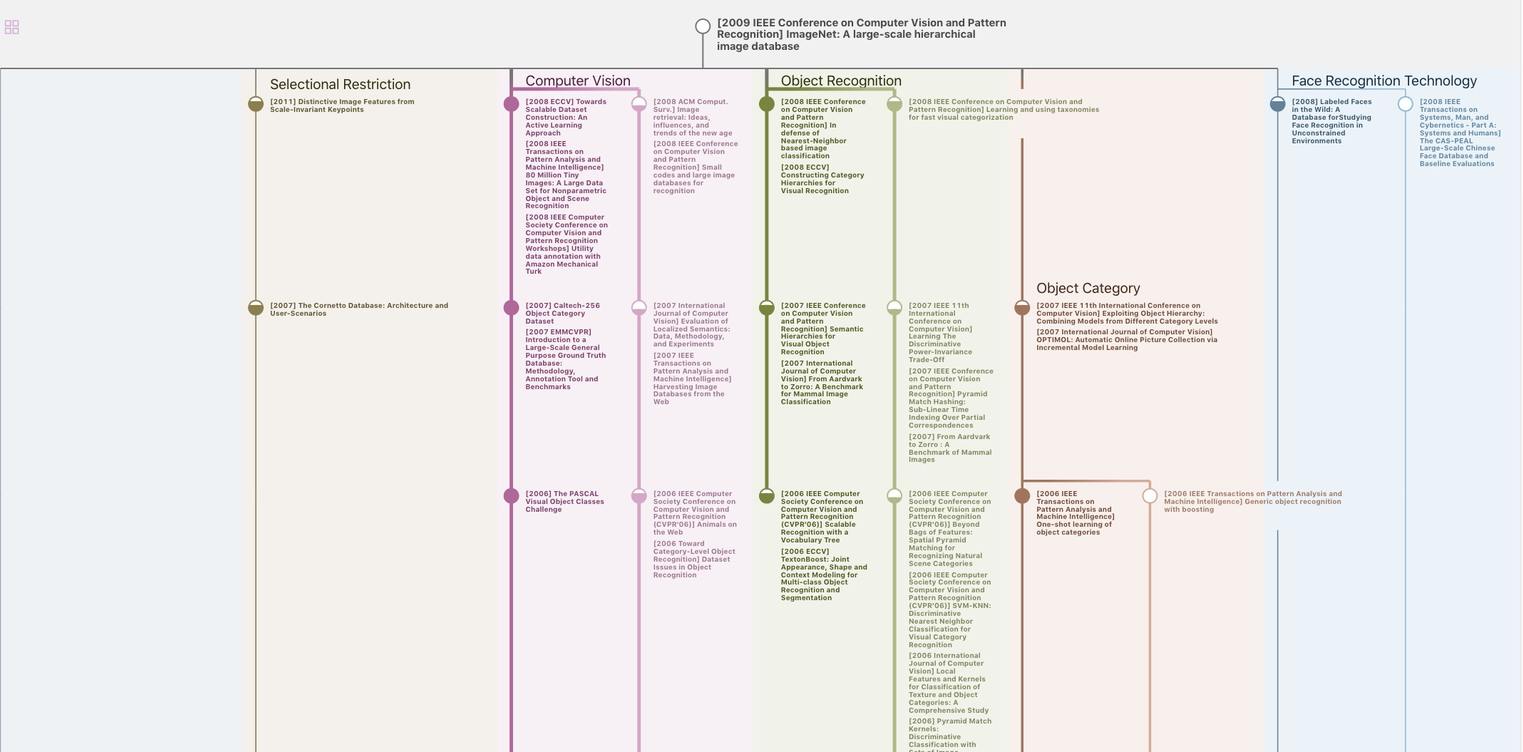
生成溯源树,研究论文发展脉络
Chat Paper
正在生成论文摘要