User-Level Membership Inference Attack against Metric Embedding Learning
arxiv(2022)
摘要
Membership inference (MI) determines if a sample was part of a victim model training set. Recent development of MI attacks focus on record-level membership inference which limits their application in many real-world scenarios. For example, in the person re-identification task, the attacker (or investigator) is interested in determining if a user's images have been used during training or not. However, the exact training images might not be accessible to the attacker. In this paper, we develop a user-level MI attack where the goal is to find if any sample from the target user has been used during training even when no exact training sample is available to the attacker. We focus on metric embedding learning due to its dominance in person re-identification, where user-level MI attack is more sensible. We conduct an extensive evaluation on several datasets and show that our approach achieves high accuracy on user-level MI task.
更多查看译文
关键词
learning,metric,user-level
AI 理解论文
溯源树
样例
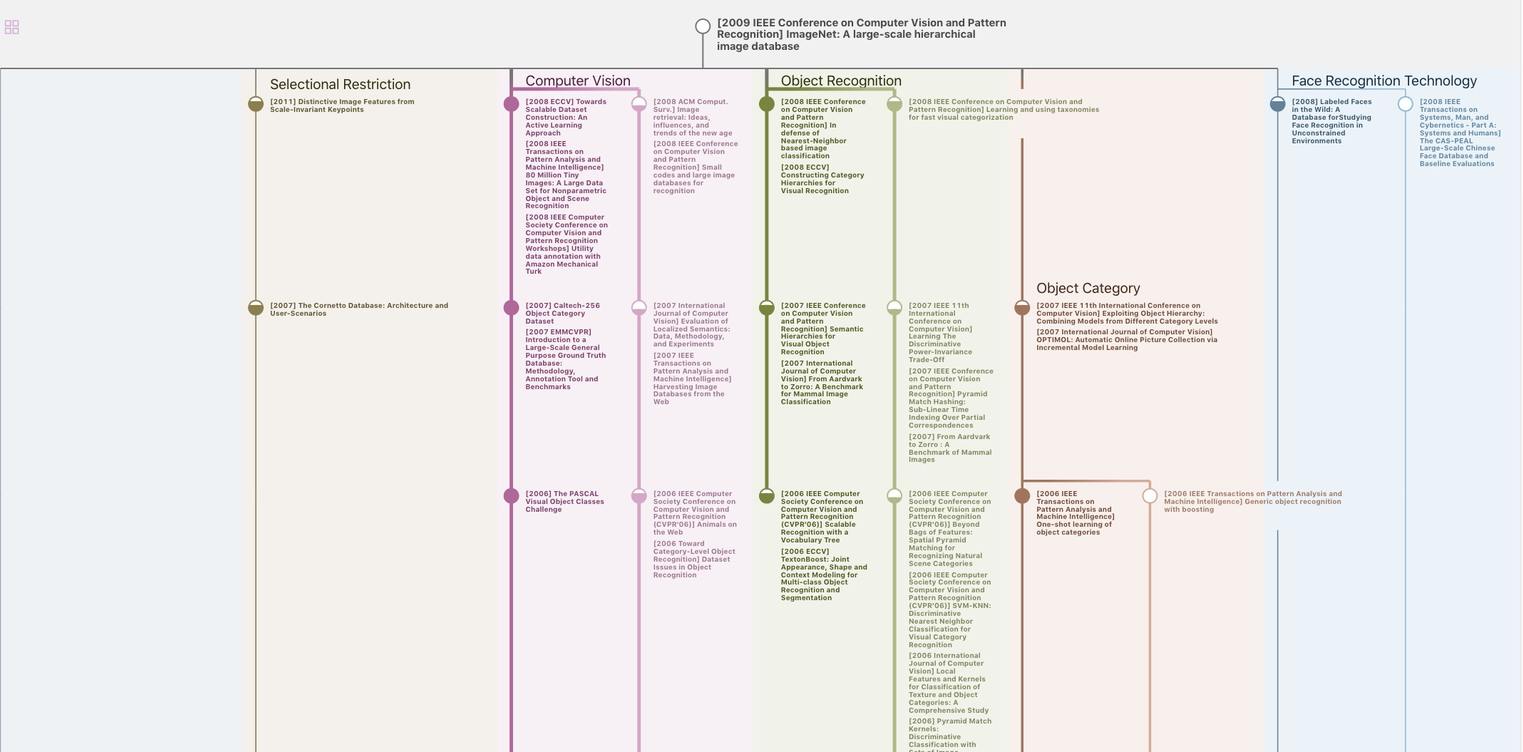
生成溯源树,研究论文发展脉络
Chat Paper
正在生成论文摘要