Deep Cascade Gradient RBF Networks With Output-Relevant Feature Extraction and Adaptation for Nonlinear and Nonstationary Processes.
IEEE transactions on cybernetics(2022)
摘要
The main challenge for industrial predictive models is how to effectively deal with big data from high-dimensional processes with nonstationary characteristics. Although deep networks, such as the stacked autoencoder (SAE), can learn useful features from massive data with multilevel architecture, it is difficult to adapt them online to track fast time-varying process dynamics. To integrate feature learning and online adaptation, this article proposes a deep cascade gradient radial basis function (GRBF) network for online modeling and prediction of nonlinear and nonstationary processes. The proposed deep learning method consists of three modules. First, a preliminary prediction result is generated by a GRBF weak predictor, which is further combined with raw input data for feature extraction. By incorporating the prior weak prediction information, deep output-relevant features are extracted using a SAE. Online prediction is finally produced upon the extracted features with a GRBF predictor, whose weights and structure are updated online to capture fast time-varying process characteristics. Three real-world industrial case studies demonstrate that the proposed deep cascade GRBF network outperforms existing state-of-the-art online modeling approaches as well as deep networks, in terms of both online prediction accuracy and computational complexity.
更多查看译文
关键词
Adaptation models,Feature extraction,Data models,Predictive models,Computational modeling,Adaptive systems,Radial basis function networks,Deep learning,gradient radial basis function (GRBF) network,high-dimensional and nonstationary processes,online adaptation,output-relevant features,stacked autoencoder (SAE)
AI 理解论文
溯源树
样例
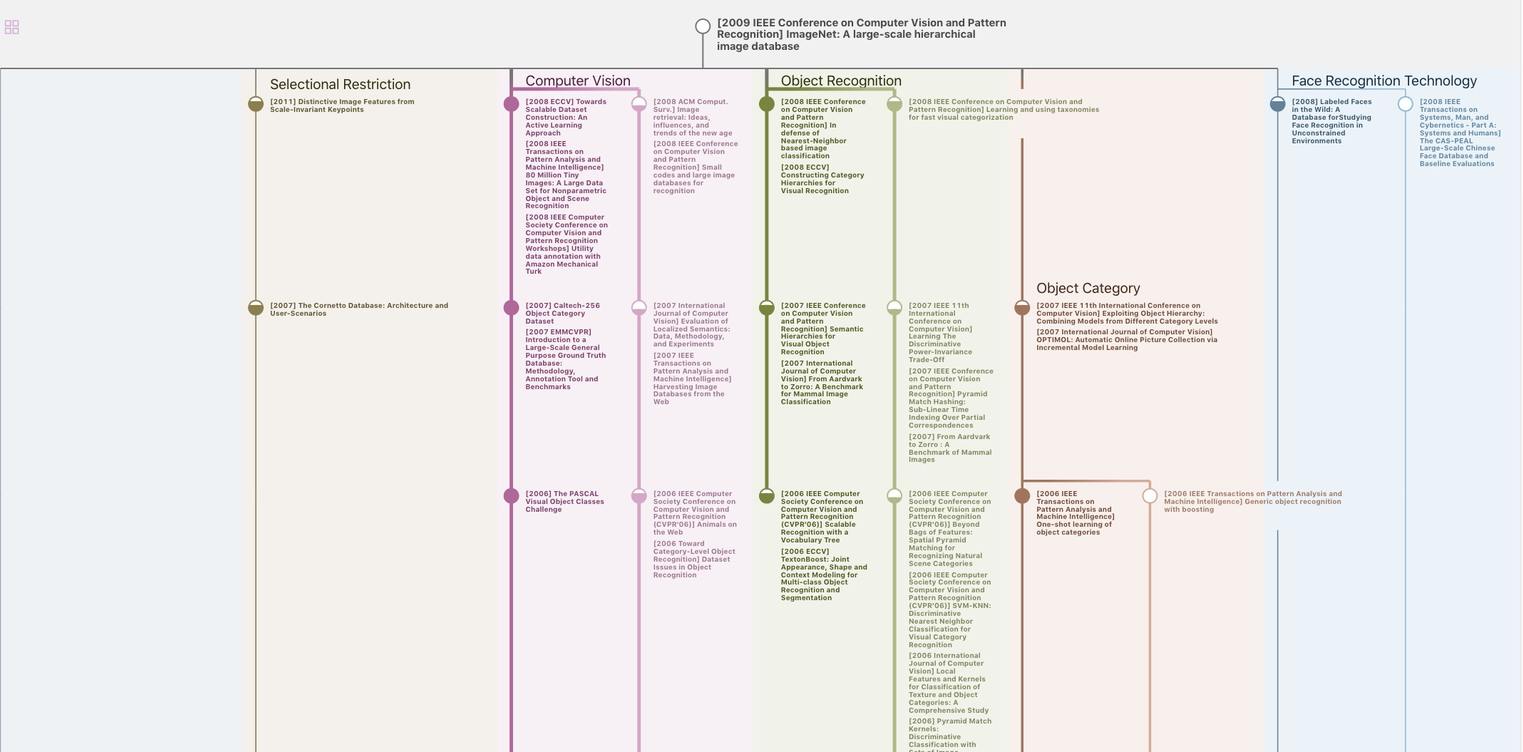
生成溯源树,研究论文发展脉络
Chat Paper
正在生成论文摘要