Subject-Independent, Biological Hip Moment Estimation During Multimodal Overground Ambulation Using Deep Learning
IEEE Transactions on Medical Robotics and Bionics(2022)
摘要
Estimating biological joint moments using wearable sensors could enable out-of-lab biomechanical analyses and exoskeletons that assist throughout daily life. To realize these possibilities, this study introduced a subject-independent hip moment estimator using a temporal convolutional network (TCN) and validated its performance and generalizability during multimodal ambulation. Electrogoniometer and simulated IMU data from sixteen participants walking on level ground, ramps and stairs were used to evaluate our approach when benchmarked against a fully-connected neural network, a long short-term memory network, and a baseline method (i.e., using subject-average moment curves based on ambulation mode and gait phase). Additionally, the generalizability of our approach was evaluated by testing on ground slopes, stair heights, and gait transitions withheld during model training. The TCN outperformed the benchmark approaches on the hold-out data (p < 0.05), with an average RMSE of 0.131±0.018 Nm/kg and R
2
of 0.880±0.030 during steady-state ambulation. When tested on the 20 leave-one-out slope and stair height conditions, the TCN significantly increased RMSE only on the steepest (+18°) incline (p < 0.05). Finally, the TCN RMSE and R
2
was 0.152±0.027 Nm/kg and 0.786±0.055, respectively, during mode transitions. Thus, our approach accurately estimated hip moments and generalized to unseen gait contexts using data from three wearable sensors.
更多查看译文
关键词
Biomechanics estimation,machine learning,multimodal ambulation,neural networks,wearable sensors
AI 理解论文
溯源树
样例
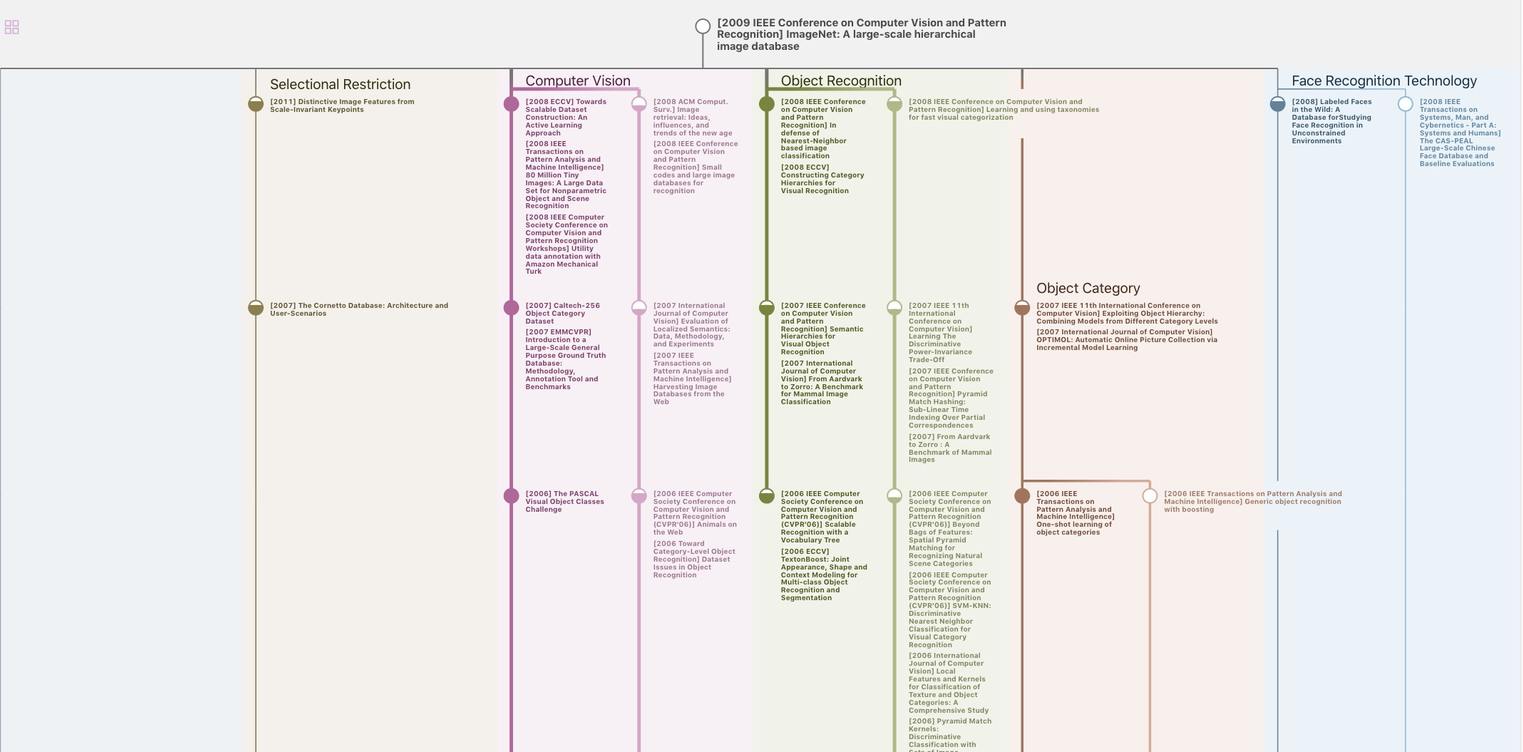
生成溯源树,研究论文发展脉络
Chat Paper
正在生成论文摘要