An Uncertainty-based Neural Network for Explainable Trajectory Segmentation
ACM Transactions on Intelligent Systems and Technology(2022)
摘要
AbstractAs a variant task of time-series segmentation, trajectory segmentation is a key task in the applications of transportation pattern recognition and traffic analysis. However, segmenting trajectory is faced with challenges of implicit patterns and sparse results. Although deep neural networks have tremendous advantages in terms of high-level feature learning performance, deploying as a blackbox seriously limits the real-world applications. Providing explainable segmentations has significance for result evaluation and decision making. Thus, in this article, we address trajectory segmentation by proposing a Bayesian Encoder-Decoder Network (BED-Net) to provide accurate detection with explainability and references for the following active-learning procedures. BED-Net consists of a segmentation module based on Monte Carlo dropout and an explanation module based on uncertainty learning that provides results evaluation and visualization. Experimental results on both benchmark and real-world datasets indicate that BED-Net outperforms the rival methods and offers excellent explainability in the applications of trajectory segmentation.
更多查看译文
关键词
Trajectory segmentation, time series, explainable neural network, uncertainty learning
AI 理解论文
溯源树
样例
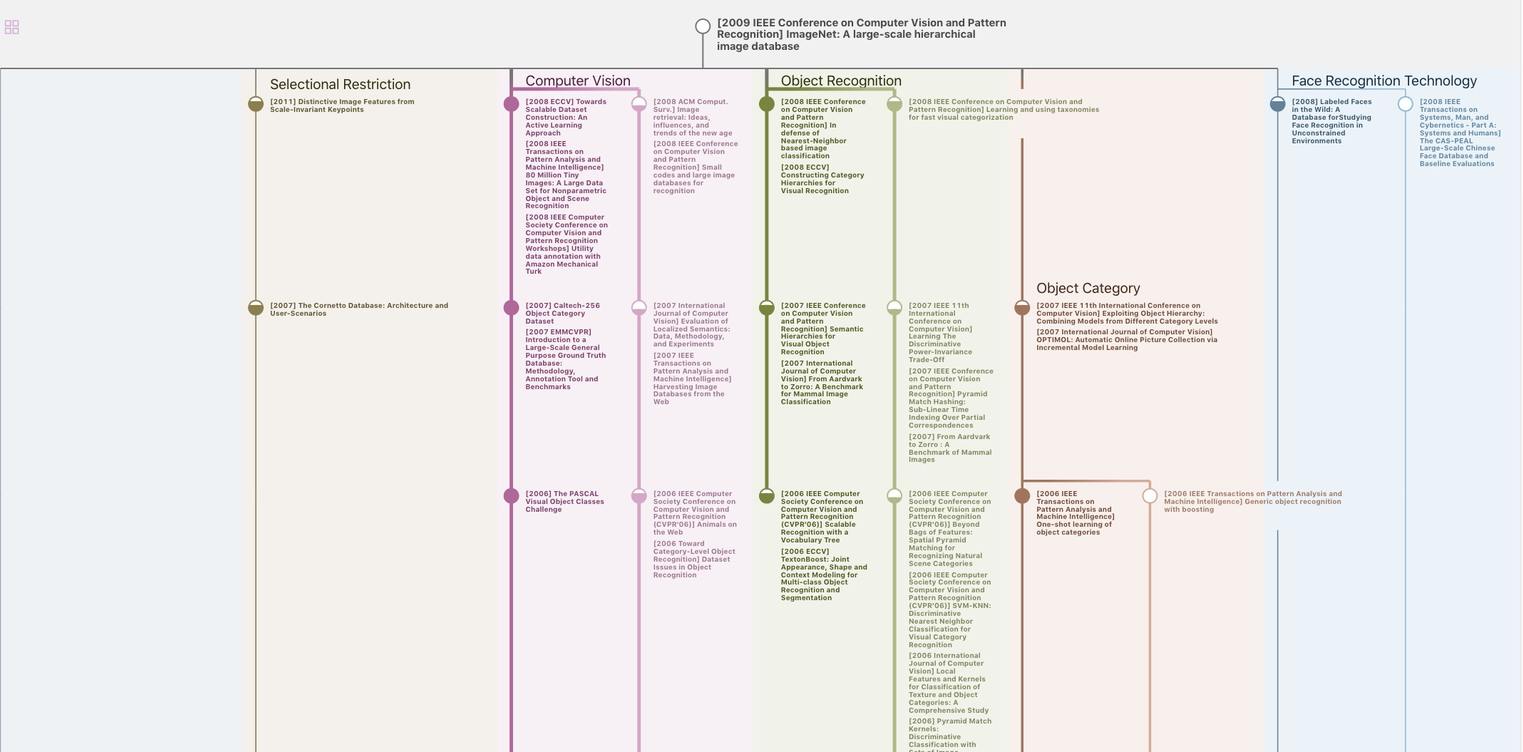
生成溯源树,研究论文发展脉络
Chat Paper
正在生成论文摘要