An Open Challenge for Inductive Link Prediction on Knowledge Graphs
arxiv(2022)
摘要
An emerging trend in representation learning over knowledge graphs (KGs) moves beyond transductive link prediction tasks over a fixed set of known entities in favor of inductive tasks that imply training on one graph and performing inference over a new graph with unseen entities. In inductive setups, node features are often not available and training shallow entity embedding matrices is meaningless as they cannot be used at inference time with unseen entities. Despite the growing interest, there are not enough benchmarks for evaluating inductive representation learning methods. In this work, we introduce ILPC 2022, a novel open challenge on KG inductive link prediction. To this end, we constructed two new datasets based on Wikidata with various sizes of training and inference graphs that are much larger than existing inductive benchmarks. We also provide two strong baselines leveraging recently proposed inductive methods. We hope this challenge helps to streamline community efforts in the inductive graph representation learning area. ILPC 2022 follows best practices on evaluation fairness and reproducibility, and is available at https://github.com/pykeen/ilpc2022.
更多查看译文
关键词
inductive link prediction,knowledge graphs,open challenge
AI 理解论文
溯源树
样例
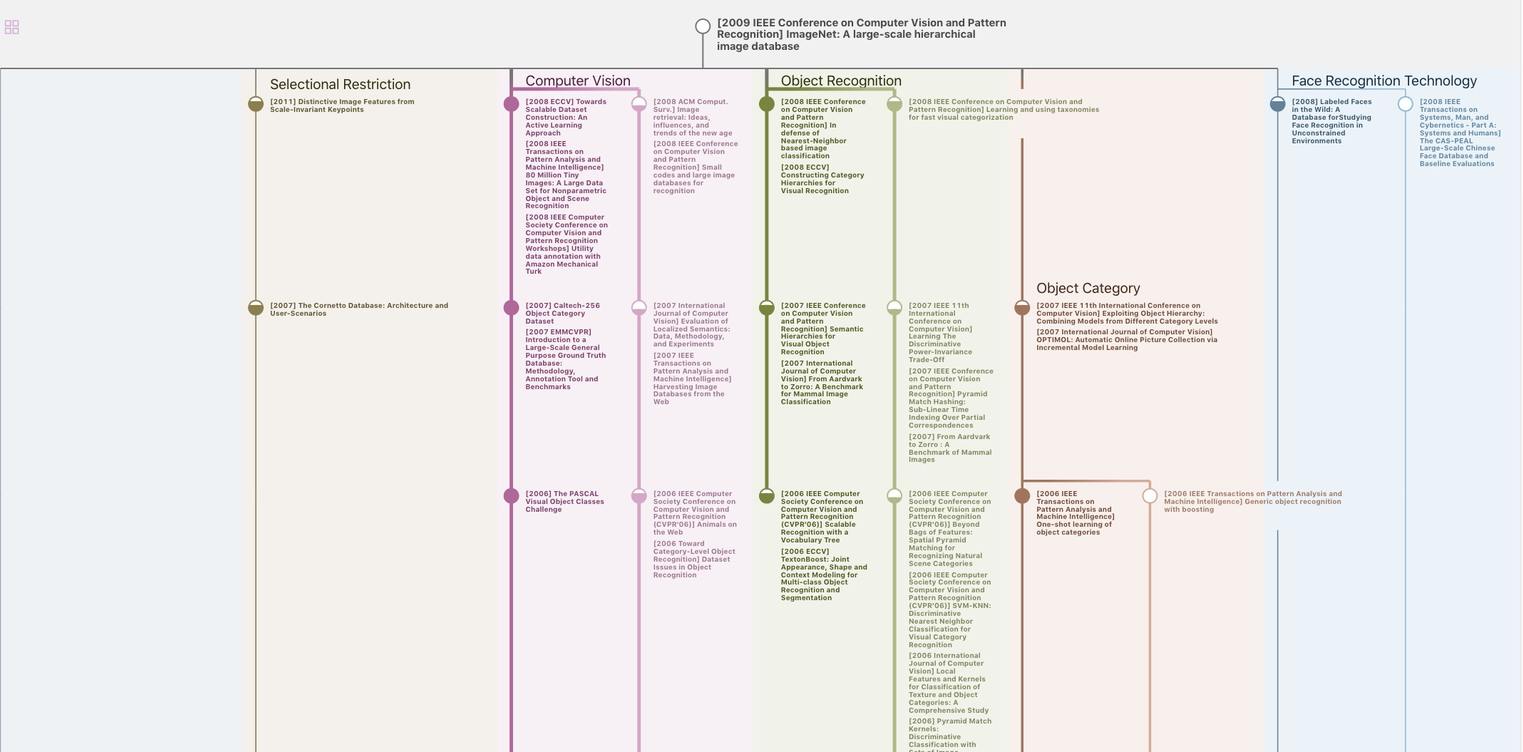
生成溯源树,研究论文发展脉络
Chat Paper
正在生成论文摘要