Adaptive Gradient Methods with Local Guarantees
ICLR 2023(2023)
摘要
Adaptive gradient methods are the method of choice for optimization in machine learning and used to train the largest deep models. In this paper we study the problem of learning a local preconditioner, that can change as the data is changing along the optimization trajectory. We propose an adaptive gradient method that has provable adaptive regret guarantees vs. the best local preconditioner. To derive this guarantee, we prove a new adaptive regret bound in online learning that improves upon previous adaptive online learning methods. We demonstrate the robustness of our method in automatically choosing the optimal learning rate schedule for popular benchmarking tasks in vision and language domains. Without the need to manually tune a learning rate schedule, our method can, in a single run, achieve comparable and stable task accuracy as a fine-tuned optimizer.
更多查看译文
关键词
adaptive gradient methods
AI 理解论文
溯源树
样例
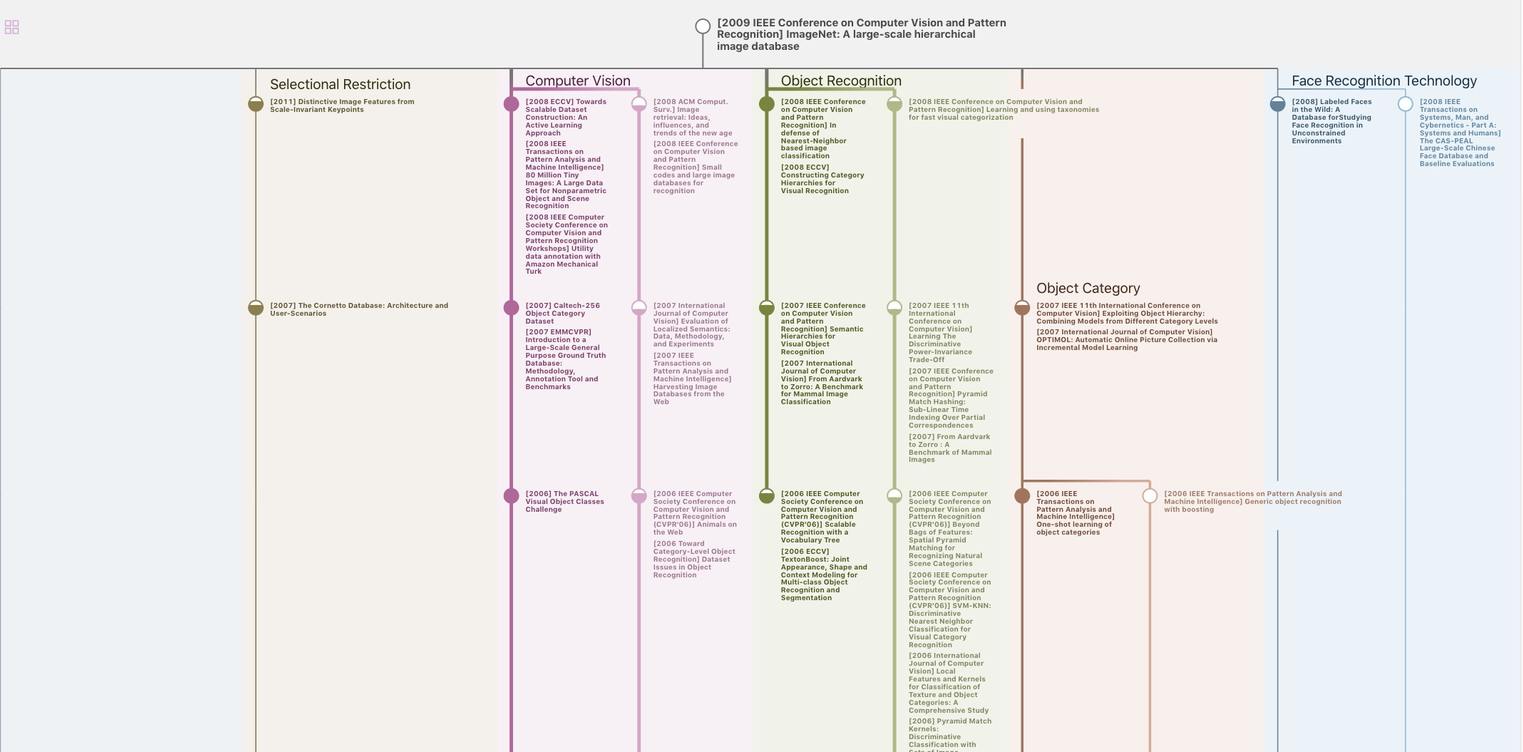
生成溯源树,研究论文发展脉络
Chat Paper
正在生成论文摘要