A transformer-based deep neural network for arrhythmia detection using continuous ECG signals.
Computers in biology and medicine(2022)
Abstract
Recently, much effort has been put into solving arrhythmia classification problems with machine learning-based methods. However, inter-heartbeat dependencies have been ignored by many researchers which possess the potential to boost arrhythmia classification performance. To address this problem, this paper proposes a novel transformer-based deep learning neural network, ECG DETR, which performs arrhythmia detection on continuous single-lead ECG segments. The proposed model simultaneously predicts the positions and categories of all the heartbeats within an ECG segment. Therefore, the proposed method is a more compact end-to-end arrhythmia detection algorithm compared with beat-by-beat classification methods as explicit heartbeat segmentation is not required. The performance and generalizability of our proposed scheme are verified on the MIT-BIH arrhythmia database and MIT-BIH atrial fibrillation database. Experiments are carried out on three different arrhythmia detection tasks including 8, 4, and 2 distinct labels respectively using 10-fold cross-validation. According to the results, the suggested method yields comparable performance in contrast with previous works considering both heartbeat segmentation and classification, which achieved an overall accuracy of 99.12%, 99.49%, and 99.23% on the three aforementioned tasks.
MoreTranslated text
AI Read Science
Must-Reading Tree
Example
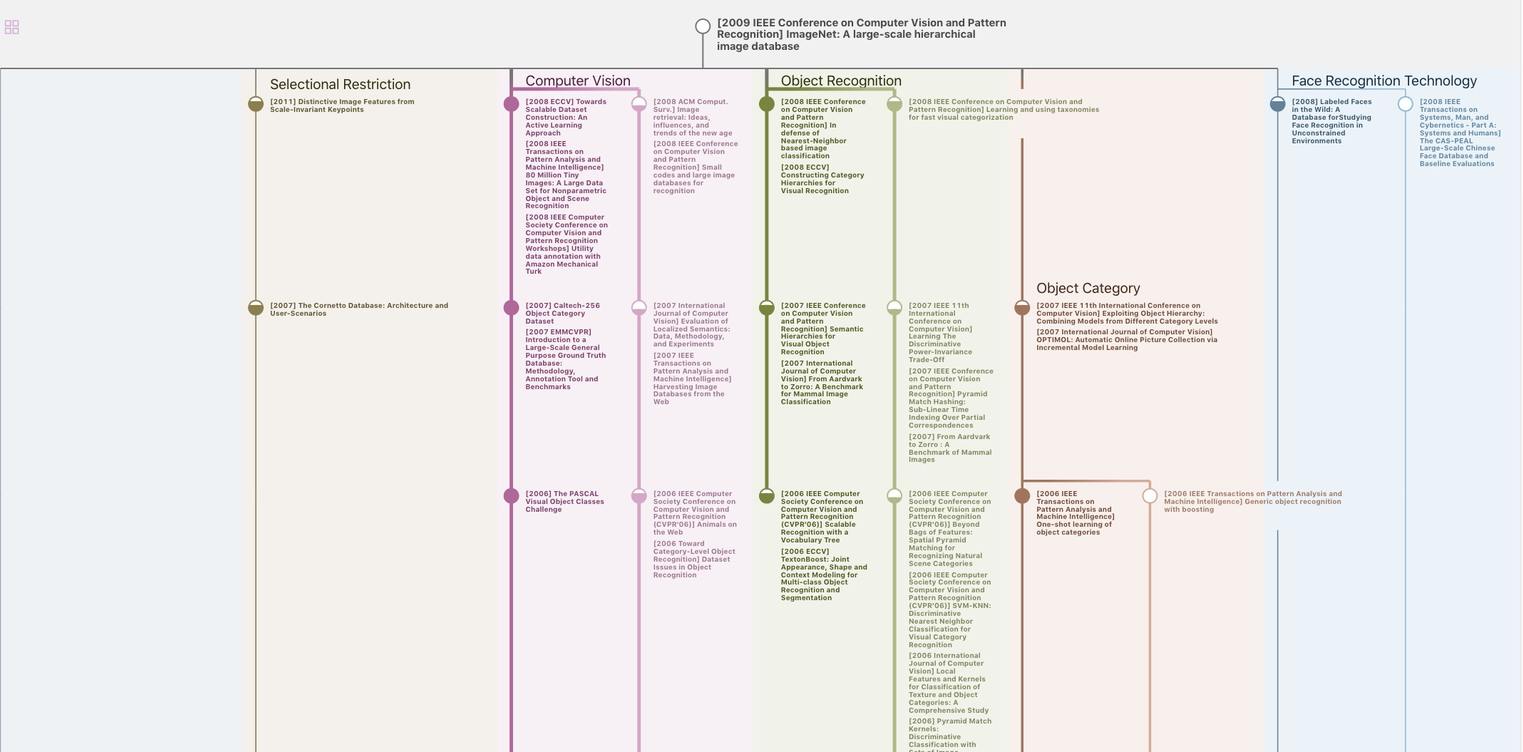
Generate MRT to find the research sequence of this paper
Chat Paper
Summary is being generated by the instructions you defined