An Intelligent handcrafted feature selection using Archimedes optimization algorithm for facial analysis
Soft Computing(2022)
摘要
Human facial analysis (HFA) has recently become an attractive topic for computer vision research due to technological progress and mobile applications. HFA explores several issues as gender recognition (GR), facial expression, age, and race recognition for automatically understanding social life. This study explores HFA from the angle of recognizing a person’s gender from their face. Several hard challenges are provoked, such as illumination, occlusion, facial emotions, quality, and angle of capture by cameras, making gender recognition more difficult for machines. The Archimedes optimization algorithm (AOA) was recently designed as a metaheuristic-based population optimization method, inspired by the Archimedes theory’s physical notion. Compared to other swarm algorithms in the realm of optimization, this method promotes a good balance between exploration and exploitation. The convergence area is increased By incorporating extra data into the solution, such as volume and density. Because of the preceding benefits of AOA and the fact that it has not been used to choose the best area of the face, we propose utilizing a wrapper feature selection technique, which is a real motivation in the field of computer vision and machine learning. The paper’s primary purpose is to automatically determine the optimal face area using AOA to recognize the gender of a human person categorized by two classes (Men and women). In this paper, the facial image is divided into several subregions (blocks), where each area provides a vector of characteristics using one method from handcrafted techniques as the local binary pattern (LBP), histogram-oriented gradient (HOG), or gray-level co-occurrence matrix (GLCM). Two experiments assess the proposed method (AOA): The first employs two benchmarking datasets: the Georgia Tech Face dataset (GT) and the Brazilian FEI dataset. The second experiment represents a more challenging large dataset that uses Gallagher’s uncontrolled dataset. The experimental results show the good performance of AOA compared to other recent and competitive optimizers for all datasets. In terms of accuracy, the AOA-based LBP outperforms the state-of-the-art deep convolutional neural network (CNN) with 96.08% for the Gallagher’s dataset.
更多查看译文
关键词
Archimedes optimization algorithm (AOA), Human facial analysis (HFA), Wrapper feature selection (FS), Handcrafted methods, Automatic selection
AI 理解论文
溯源树
样例
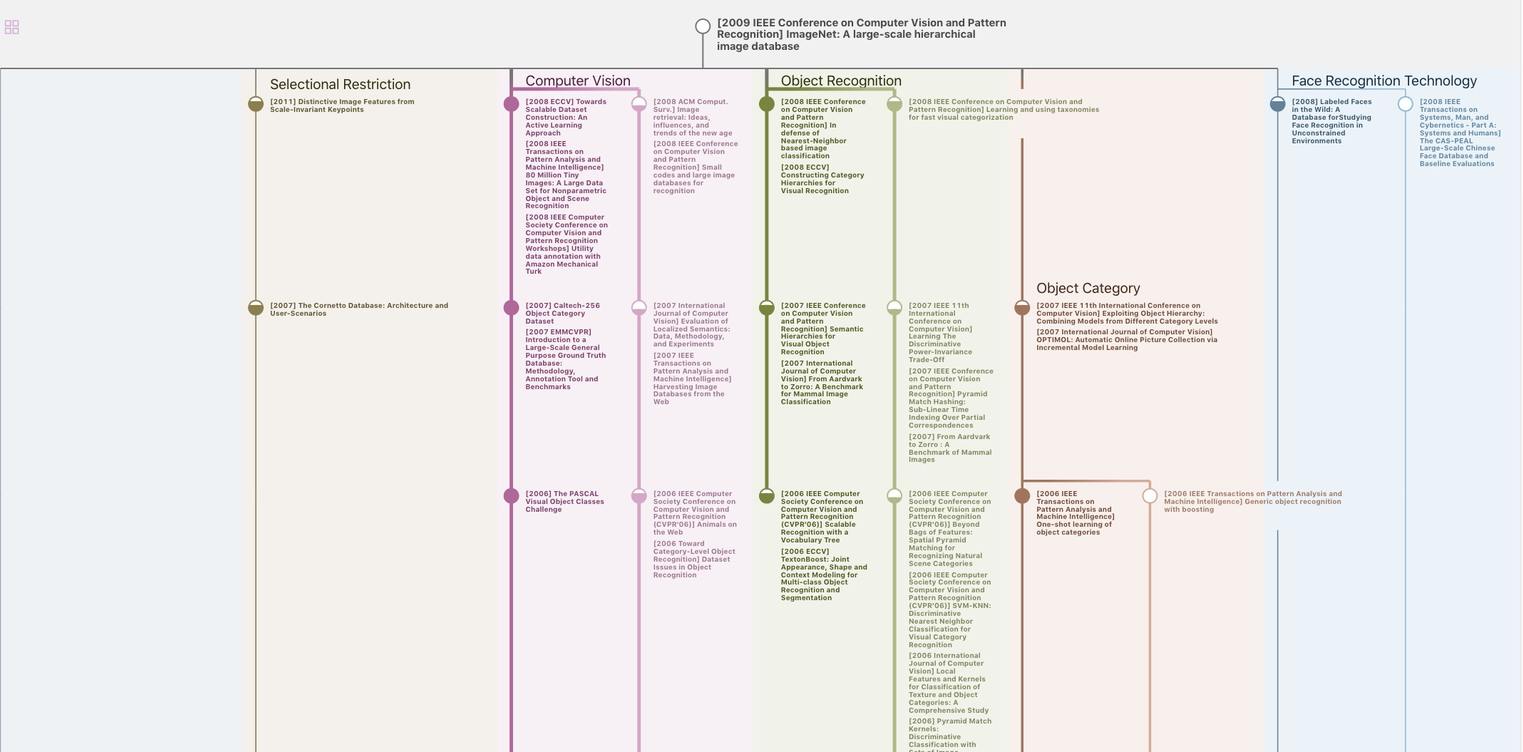
生成溯源树,研究论文发展脉络
Chat Paper
正在生成论文摘要