A General Null Space Property for Sparse Principal Component Analysis
Circuits, Systems, and Signal Processing(2022)
Abstract
Sparse principal component analysis (SPCA) has achieved great success in improving interpretable ability of the derived results and has become a powerful technique for modern data analysis. It presents that principal component can be modified to produce sparse loadings by imposing sparsity-induced penalty, which is often l_1 -regularized constraint. In order to analyze the l_1 -regularized sparsity-induced model, in this paper, we propose a general null space property of a matrix 𝐀 relative to a index set S and give a necessary and sufficient condition for the exact or approximate sparse principal components. Meanwhile, the conclusions with respect to the stable and robust situations are given in the case of exact or approximate sparse principal components, respectively.
MoreTranslated text
Key words
Principal component analysis (PCA), Sparse principal component analysis (SPCA), General null space property (GNSP)
AI Read Science
Must-Reading Tree
Example
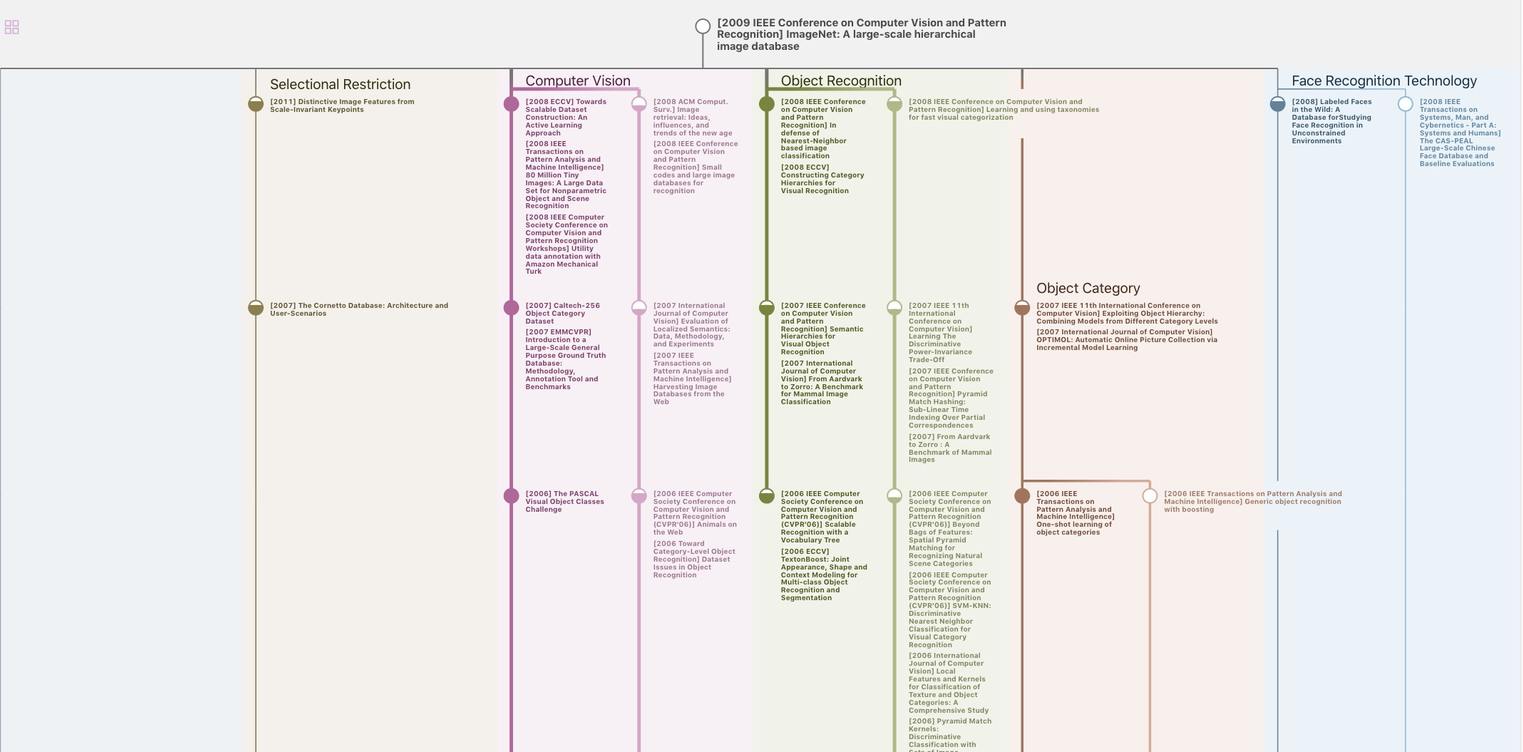
Generate MRT to find the research sequence of this paper
Chat Paper
Summary is being generated by the instructions you defined